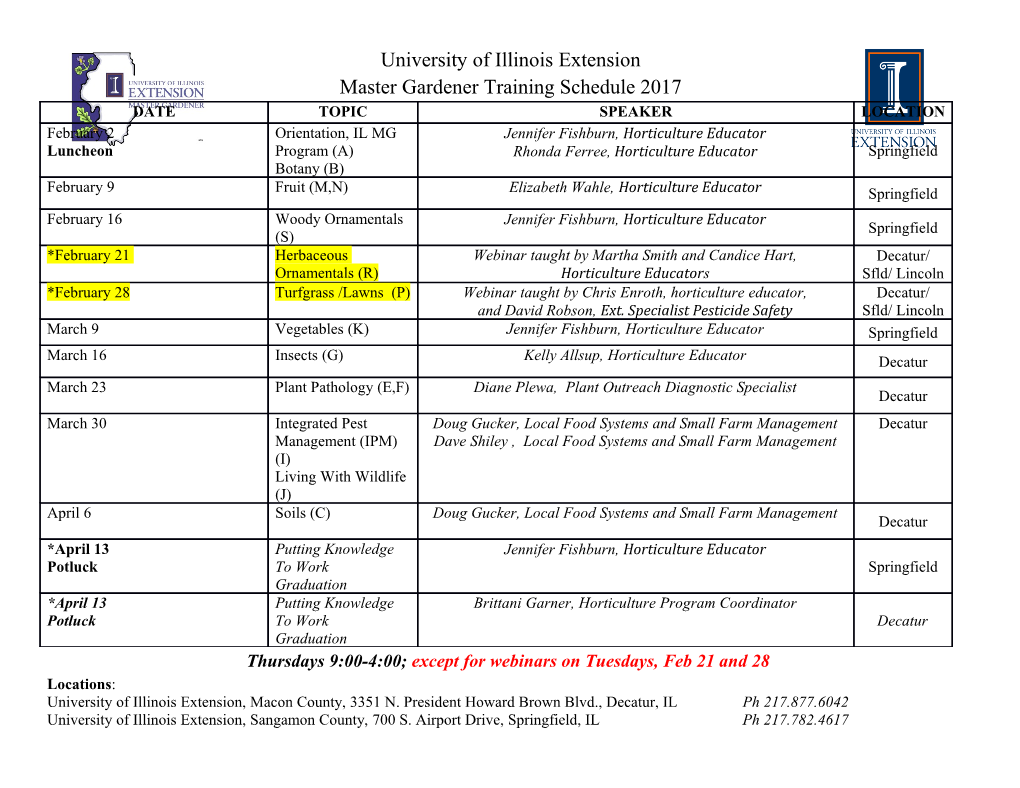
Earthq Sci (2009)22: 315−320 315 Doi: 10.1007/s11589-009-0315-8 Application of support vector machine to ∗ synthetic earthquake prediction Chun Jiang 1, Xueli Wei 2 Xiaofeng Cui 1 and Dexiang You 2 1 Earthquake Administration of Tianjin Municipality, Tianjin 300201, China 2 Tianjin University of Technology, Tianjin 300191, China Abstract This paper introduces the method of support vector machine (SVM) into the field of synthetic earthquake pre- diction, which is a non-linear and complex seismogenic system. As an example, we apply this method to predict the largest annual magnitude for the North China area (30°E−42°E, 108°N−125°N) and the capital region (38°E−41.5°E, 114°N−120°N) on the basis of seismicity parameters and observed precursory data. The corresponding prediction rates for the North China area and the capital region are 64.1% and 75%, respectively, which shows that the method is feasible. Key words: support vector machine; seismicity parameter; precursory data; synthetic earthquake prediction CLC number: P315.75 Document code: A 1 Introduction 2 Basic principle of SVM regression The support vector machine (SVM) has not only a algorithm (Vapnik, 1995) strict theory basis, but also a strong generalization (pre- It is firstly to consider the linear regression model. diction) capacity, which can better solve the practical Suppose that a sample with k cases is described as problems of small samples, nonlinearity, higher dimen- ∈n × sion and local minimum point. At present, SVM are (x1 ,y 1 ), ( x 2 , y 2 ),L ,(xk ,y k )RR , (1) widely used in text classification, handwriting recogni- the linear discriminant function is tion, image classification and bioinformatics, and now it =• + (2) has been extended to synthetic estimates and prediction f ()x w x b of time series. Wang et al (2005, 2006) introduced the and assume that all the training data can be fitted as a method to predict strong earthquakes in Chinese linear function without error under the accuracy of ε, mainland and studied the non-linear relations between that is, the time series of strong seismicity in China and the −• + ≤ ε ⎧yi ()w xi b global large earthquakes as well as sunspot activities. ⎨ i=1, 2, , k . (3) • + − ≤ ε L His applications have obtained some meaningful results. ⎩()w xi b yi In view of that earthquake preparation-occurrence Considering the case of permissible fitting error, is a complex nonlinear dynamic process, we introduce * the relaxation indexes ξi≥0 and ξ ≥0 are introduced. SVM into a kind of synthetic earthquake prediction. The i Then equation (3) turns into the following equation: preliminary research by this method on the basis of seismic parameters has obtained a better result (Jiang et ⎧y −()w• x +b ≤ε + ξ i i i = ⎨ ∗ i1,2,L , k , (4) al, 2006). In this paper, SVM is used for a synthetic • + − ≤ε + ξ ⎩()w xi b yi i earthquake prediction in the North China area and the ξ ≥ ξ * ≥ capital region on the basis of both seismicity parameters where i 0 and i 0. That the SVM used for regres- and observed precursory data. sion estimate is minimizing function (5) under con- straints (4). ∗ Received 21 October 2008; accepted in revised form 2 February 2009; published 10 June 2009. Corresponding author. e-mail: [email protected] 316 Earthq Sci (2009)22: 315−320 k φ •φ * 1 * If K(xi, xj)= (xi) (xj), then equation (9) is turned ξ ξ =• +ξ + ξ R(,,)w w w C∑ ()i i (5) 2 i=1 into k The first term of equation (5) is to make the regression ω~ α α * = −1 α − α* α − α * ⋅ (,) * ( )( ) w,,,b ξ ξ ∑ i i j j function more flat, so as to enhance the generalization 2 i, j= 1 ability. The second term is for reducing errors, the con- k k −α + α* ε + α − α * K(,)()()xi x j ∑ i i ∑ i iy i , (10) stant C>0 controls the punishment of samples whose i=1 i=1 errors are more than ε, where ε is a positive constant. If where − | f (xi) yi|<ε, then it will be ignored, otherwise, the error k =α − α * φ counted as | f (xi)−yi|−ε. w ∑ ()(i ix i ). (11) i=1 With fewer samples, we generally use the dual φ theory to find a solution for SVMs and turn it into a Denoting w• (x)=w0, f (x) can be expressed as k quadratic programming problem. Then the Lagrangian =α − α * + = + f ()()(,)x ∑ i iK x i x j b w0 b. (12) function is introduced: i=1 * ** 1 The theory of SVM uses the scalar product K(x , x ) = L(,,,,,,,)w b ξ ξ α α γ γ =w• w + i j φ • φ 2 (xi) (xj) in the high-dimensional eigenspace rather k k ξ+ ξ* − α ξ + ε − + − than uses function φ directly, thus the problem that w C∑ ()[i i ∑ i i yi f (xi )] i=1 i=1 cannot be expressed with the unknown φ is solved clev- k k α** ξ+ ε + − −ξ γ + ξ** γ erly, where K(xi, xj) is called kernel function. It has been ∑ i[ i yi f (xi )]∑ (i i i i ). (6) i=1 i=1 proved that a symmetric function can be a kernel as long α α * γ γ * ⋅⋅⋅ as it meets the Mercer condition. The common kernel where, i, i , i and i (i=1, 2, , k) are not less than functions are: 1 Polynomial kernel function K(xi, xj) = zero. Then the dual function of the Lagrange function (6) d (x • x +c) , c>0, d =1, 2, ⋅⋅⋅; 2 Radial basis kernel func- is i j = − − 2σ 2 tion (RBF) K(xi , x j ) exp( ||xi x j || 2 ); 3 Sig- k * 1 * * ω~ α α = −α − α α − α • − (,) * ( )( )(x x ) w,,,b ξ ξ ∑ i i j j i j moid kernel function K(x , x )= tanh[b (x• x )+ c ] . 2 i, j= 1 i j i j k k It should be noted that these kernel functions have α+ α* ε + α − α * ∑()()i i ∑ i iy i . (7) their own input ranges, which should be taken a i=1 i=1 data-scale transformation before specific applications. The dual solution to this problem is maximizing equa- Selecting kernel function needs certain prior knowledge tion (7) under constraints (8) (Xi, 1983) and there is no general conclusion at present. Scholkopf k α− α * = et al (1998) discussed the selection and construction of ∑ (i i ) 0, (8) i=1 kernel functions. * According to the Karush-Kuhn-Tucker (KKT) where 0≤αi≤C and ≤ 0≤α ≤ C , and i=1, 2, ⋅⋅⋅, k. i conditions (Xi, 1983), at the optimal solution we have The basic idea of non-linear approximation is: α ε+ ξ − + = firstly, mapping the input space into the ⎧ i[ iy i f (xi )] 0 ⎨ i = 1, 2, , k (13) high-dimensional eigen-space by the non-linear trans- α* ε+ ξ * + − = L ⎩ i[ iy i f (xi )] 0 formation φ (x); secondly, doing linear approximation in and the high-dimensional eigenspace, that is f (x)=w•φ (x)+b; ξ γ = then obtaining the non-linear regression result in the ⎧ i i 0 ⎨ i=1, 2, , k . (14) original space. Thus the issue of non-linear regression is ξ** γ = L ⎩ i i 0 turned into maximizing function α α * = k From equation (13) we have i i 0 , and ω~ α α * = −1 α − α* α − α * ⋅ (,) * ( )( ) w,,,b ξ ξ ∑ i i j j = = −ε − • φ 2 i, j 1 ⎧b yi w() xi k k ⎨ . (15) * * =ε + − • φ φ• φ −α + α ε + α − α ⎩b yi w() xi [ (xi ) ( x j )]∑ (i i )∑ (i i )y i (9) i=1 i=1 The above-mentioned algorithms can be achieved easily under constraints (8). by using the existing optimized software packages. Earthq Sci (2009)22: 315−320 317 3 Application of SVM to synthetic area based on the analysis of 210 earthquake cases from Earthquake Cases in China (Zhang, 1988, 1990a, b, earthquake prediction 1999, 2000; Chen, 2002a, b, c) and determine the fol- According to the incomplete figures, dozens of lowing rules for selecting earthquake cases and seismic seismicity parameters and precursors are used in moni- precursory abnormal indexes: toring and predicting earthquakes in China at present, 1) Earthquake cases with relatively concentrated such as hydrochemistry, water level, geoelectricity, seismic precursory anomalies in the observation items geomagnetism, electromagnetic wave, crustal deforma- should be the first choice. The seismic precursory ab- tion, gravity, stress and so on. However, earthquake normal indexes of M5.0−5.9, M6.0−6.9 and M≥7.0 preparation is a non-linear and unstable process, ac- earthquakes should make up 15%−20%, 30%−40% and companying a large number of different random phe- ≥50% of the total observation items, respectively. nomena. And seismicity parameters and earthquake 2) Seismic precursory anomalies relatively concen- precursors often show their single or group abnormal trated in a larger scope in the vicinity of epicentral re- features in different forms. No matter in the synthetic gion are selected. The seismic precursory anomalies of earthquake prediction based on different groups of seis- M5.0−5.9, M6.0−6.9 and M≥7.0 earthquakes should lo- mic precursory anomalies by the same method or based cate within a radius of 200 km, 300 km and 500 km to on the same group of seismic precursory anomalies by the epicenters, respectively. different methods, the conclusions drawn from different 3) Single seismic precursory anomaly with a phases or the same phase of seismic activities are often high-R score should be firstly selected.
Details
-
File Typepdf
-
Upload Time-
-
Content LanguagesEnglish
-
Upload UserAnonymous/Not logged-in
-
File Pages6 Page
-
File Size-