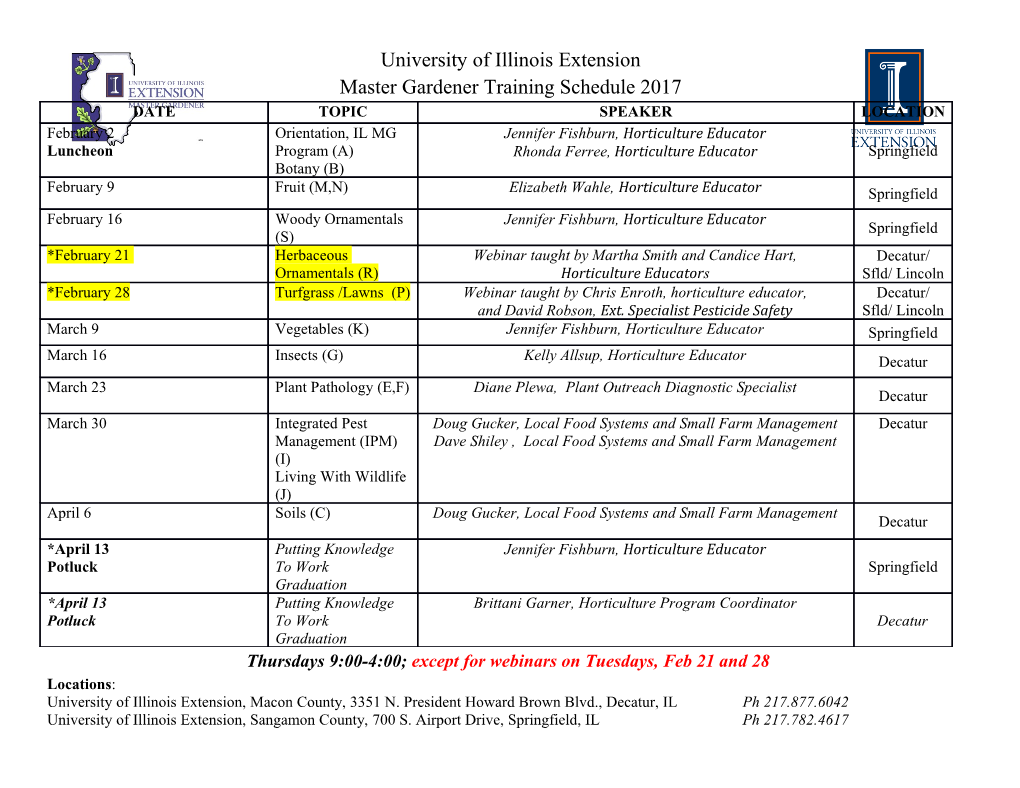
Exploring Task Parallelism for Heterogeneous Systems Using Multicore Task Management API Suyang Zhu1, Sunita Chandrasekaran2, Peng Sun1, Barbara Chapman1, Marcus Winter3, and Tobias Schuele4 1 Dept. of Computer Science, University of Houston [email protected], [email protected], [email protected] 2 Dept. of Computer and Information Sciences, University of Delaware [email protected] 3 Hypnolords Gbr [email protected] 4 Siemens Corporate Technology [email protected] Abstract. Current trends in multicore platform design indicate that heterogeneous systems are here to stay. Such systems include processors with specialized accelerators supporting different instruction sets and different types of memory spaces among several other features. Unfor- tunately, these features increase the effort for programming and porting applications to different target platforms. To solve this problem, effec- tive programming strategies that can exploit the rich feature set of such heterogeneous multicore architectures are required without leading to an increased learning curve for applying these strategies. In this paper, we explore the Multicore Task Management API (MTAPI), a standard for task-based parallel programming in embedded systems. MTAPI provides interfaces for decomposing a problem into a set of tasks while abstracting from the heterogeneous nature of the target platforms. This way, it allows to efficiently distribute work in systems consisting of different cores running at different speed. For evaluation purposes, we use an NVIDIA Jetson TK1 board (ARM + GPU) as our test bed. As applications, we employ codes from benchmark suites such as Rodinia and BOTS. Keywords: Multicore Systems, Runtime, Heterogeneity, Accelerators, MTAPI 1 Introduction Embedded multicore systems are widely used in areas such as networking, au- tomobiles, and robotics. Some of these systems even compete with HPC plat- forms [19] and are promising to deliver high GFLOPs/Watt. Since parallelism has become a major driving force in computing, microprocessor vendors concen- trate on integrating accelerators together with the CPU on the same platform. The current trend of such platforms is that they are heterogeneous in nature, i.e., their instruction sets and memory spaces are usually different [13]. For exam- ple, Qualcomm's heterogeneous processor Snapdragon 810 integrates an ARM Cortex CPU and an Adreno 430 GPU on the same chip. Such an integration pro- duces hardware platforms that satisfy the requirements regarding performance, flexibility, and energy consumption of embedded systems. Unfortunately, the available runtime systems and tools to port applications to other platforms are often still immature and typically fine-tuned for specific product families. As a result, maintaining a single code base across multiple target platforms is hardly feasible, which is a major concern in the industry. Programming models designed for high performance computing (HPC) plat- forms are not necessarily the best for handling embedded multicore systems, especially when these systems have limited resources or are subject to real-time constraints. Among these models, OpenMP [7] has been recently extended to support accelerators. In its current state, however, it still lacks support for the requirements in embedded systems. An alternative is OpenCL [18], which is known for its portability but also involves a steep learning curve making it a challenge to adopt applications to new hardware. The Multicore Association (MCA)5, formed by a group of companies from different domains, aims to address these challenges by providing a set of open specifications for programming embedded multicore platforms. MCA has re- leased APIs for sharing resources among different types of cores via the Multicore Resource Management API (MRAPI), inter-core communication via the Multi- core Communication API (MCAPI), and for task management via the Multicore Task Management API (MTAPI). Since these APIs are system agnostic, they facilitate development of portable code, thus improving the feasibility of running an application on more than just one hardware platform. This paper makes the following contributions: { Presents a light-weight software stack based on MTAPI targeting resource- constrained heterogeneous embedded systems { Showcases two open source MTAPI implementations6 { Evaluates the implementations using case studies on an embedded platform equipped with an ARM processor and a GPU The paper does not aim to compare and contrast between the two imple- mentations. Instead, the goal is to discuss how they can be used by software developers to port applications to heterogeneous multicore systems. The rest of the paper is organized as follows: Section 2 discusses related work, and Section 3 gives an overview of MTAPI. The design and usage of MTAPI runtime systems is described in Section 4. Section 5 presents our experimental results, and Section 6 concludes with some ideas for future work. 5 https://www.multicore-association.org 6 UH-MTAPI and Siemens MTAPI: Libraries created by the University of Houston and Siemens, respectively. 2 Related Work In this section, we discuss state-of-the-art parallel programming models for het- erogeneous multicore systems from the task parallelism perspective. OpenMP has been widely used in HPC for exploiting shared memory paral- lelism [5] and recently extended to support heterogeneous systems. OpenMP 3.1 ratified tasks, and task parallelism for multicore SoCs was implemented by sev- eral frameworks [4, 9, 14]. OpenMP 4.0 extended tasks to support dependencies evaluated in [11]|again using traditional shared memory-based architectures. Other task-based approaches include Intel's TBB [17] that assigns tasks to multiple cores through a runtime library. As most frameworks, however, TBB targets desktop and server applications and is not designed for low-footprint embedded and heterogeneous systems. Cilk [2] is a C language extensions originally developed by MIT for multi- threaded parallel computing. While Cilk simplifies the implementation of task parallel applications, it only supports shared memory environments which limits its application to homogeneous systems. OpenCL [18] is a standard designed for data parallel processing and used to program CPUs, GPUs, DSPs, etc. Although the standard can target multiple platforms, there is a steep learning curve making it a challenge to be adaptable. Moreover, achieving high performance often requires significant refactoring when switching between different hardware platforms. OmpSs (OpenMP SuperScalar) [8] is a task-based programming model which exploits parallelism based on annotations using pragmas. OmpSs has been ex- tended to many-core processors with accelerators such as multiple GPU systems. However, OmpSs needs special compiler support which hampers its usage in em- bedded systems. StarPU [1] is a tasking API that allows developers to design applications in heterogeneous environments. StarPU's runtime schedules the tasks and cor- responding data transfers among the CPU and GPUs. However, the necessary extension plug-in for GCC puts constraints on the deployment of StarPU to embedded systems with limited resources or bare-metal devices. As discussed above, there are many approaches that explore task parallelism. However, they may not be best suited for embedded platforms which, unlike traditional platforms, often lack resources and sometimes do not even have an operating systems. Additionally, many embedded systems are subject to real- time constraints and forbid dynamic memory allocation during operation which is completely ignored by the approaches discussed above. Our prior work in [20] uses MCAPI to establish communication through well-pipelined DMA protocols between Freescale's P4080 Power Architecture and the specialized RegEx Pattern Matching Engine (PME). We also created an abstraction layer for easy programmability by translating OpenMP to MRAPI [23, 24]. Designed and implemented in ANSI C, MCAPI and MRAPI do not require specific compiler support or user-defined language extensions. 3 MTAPI Overview Figure 1 gives an overview of MTAPI. Applications can be developed by directly calling the MTAPI interface or via further abstraction layers such as OpenMP (a translation from OpenMP to MTAPI is described in [21]). MTAPI can be implemented on top of most operating systems and may even run bare metal to its simple design and minimal dependencies. DOMAIN Applications High-Level Programming Model Interfaces (e.g., OpenMP) NODE 0 NODE 2 NODE 2 NODE 3 MTAPI Runtime Library OS 1 OS 2 OS 3 CUDA lib Bare Metal CPU CPU CPU CPU GPU Co-Proc Core 0 Core 1 Core 2 Core 3 Flash CPU Memory GPU Memory Memory Fig. 1. MTAPI framework In the following, we describe the main concepts of MTAPI. Node: An MTAPI node is an independent unit of execution. A node can be a process, a thread, a thread pool, a general purpose processor or an accelerator. Job and Action: A job is an abstraction representing the work and is imple- mented by one or more actions. For example, a job can be implemented by one action on the CPU and another action on the GPU. The MTAPI system binds tasks to the most suitable actions during runtime. Task: An MTAPI task is an instance of a job together with its data en- vironment. Since tasks are fine granular pieces of work, numerous tasks can be created, scheduled, and executed in parallel. A task can be offloaded to a neighboring node other than its origin node depending on the dynamic action binding. Therefore, optimized and efficient
Details
-
File Typepdf
-
Upload Time-
-
Content LanguagesEnglish
-
Upload UserAnonymous/Not logged-in
-
File Pages12 Page
-
File Size-