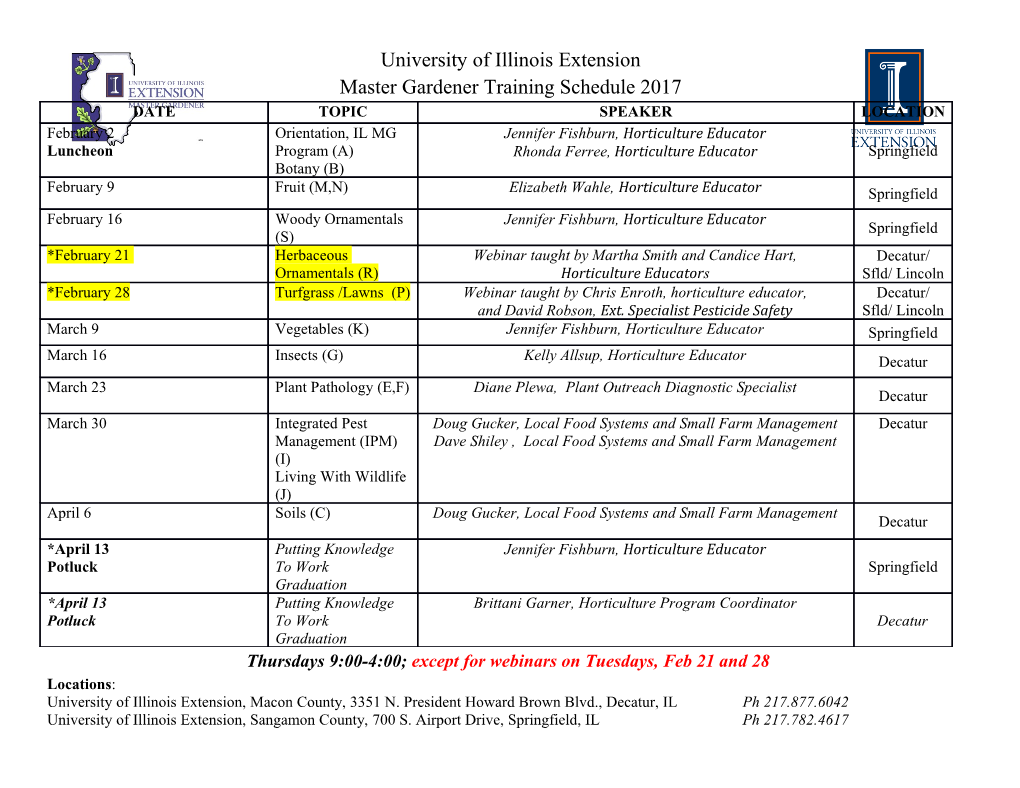
Santander Meteorology Group A multidisciplinary approach for weatherhttp://www.meteo.unican.es & climate Introduction to Model Output Statistics (MOS) and Bias Correction Methods Sixto Herrera García [email protected] Dept. Applied Mathematics and Computer Sciences, University of Cantabria, Spain www.meteo.unican.es SPECS Workshop on Seasonal Forecasting, 8-12 Sept. 2014, Santander Santander Meteorology Group A multidisciplinary approach for weather & climate Outline ! SD Classification ! Model Output Statistics (MOS) ! Bias Correction – Assumptions. – Classification. – Shortcomings. ! Multi-Variate Methods: ISI-MIP Santander Meteorology Group Statistical A multidisciplinary approach for weather & climate Downscaling Predicciones Escenarios de emisión globales RCM A2 B2 Downscaling Dinámico: basado en Modelos Regionales del Clima (RCMs) A2 Y = f (X;θ) Registros históricos Los parámetros Downscaling de los modelos A2 basado en son ajustados con Estadístico: métodos estadísticos que los datos relacionan las ocurrencias observados y locales con las simulados en simulaciones globales. clima presente. Rejilla interpolada (20 km) Statistical Santander Meteorology Group Downscaling A multidisciplinary approach for weather & climate Approach Statistical downscaling methods rely on local observations and allow to locally calibrate GCM outputs. Navacerrada (1900 m) and Madrid (700 m) are 40 km away (same GCM gridbox), but exhibit very different climate. Statistical Santander Meteorology Group Downscaling A multidisciplinary approach for weather & climate Approach 20 Statistical downscaling methods 15 rely on local observations and 10 allow to locally calibrate GCM 5 outputs. 0 OBS Max Temp (ºC) MPEH5 Navacerrada (1900 m). -5 Optim -10 SD 61-70 81-90 01-10 21-30 41-50 61-70 81-90 71-80 91-00 11-20 31-40 51-60 71-80 91-00 SD Methods Santander Meteorology Group A multidisciplinary approach for weather & climate Classification http://www.specs-fp7.eu/?q=node/45 Statistical Downscaling Santander Meteorology Group A multidisciplinary approach for weather & climate Approaches Perfect Prognosis (PP): Calibrated in the training phase using observational data for both the predictands and predictors (reanalysis). Since different GCMs are used in the training and downscaled phases, large-scale circulation variables well- resolved by the models are typically chosen as predictors in this approach. Variables directly influenced by the models’ parameterizations and orography (e.g. precipitation, instability indices, etc.) are not suitable predictors in this approach. Model Output Statistics (MOS): Predictors are taken from the global (or regional) model for both training and downscaling phases. These methods typically work directly with the variable of interest as single predictor. For instance, in MOS approaches, local precipitation is typically downscaled from the direct model precipitation forecasts. Statistical Downscaling Santander Meteorology Group A multidisciplinary approach for weather & climate Techniques General classes of downscaling Local climate = f (larger scale predictors) + locally forced variance Dynamical Empirical-statistical Perturbed observed Climate change projections: How Two approaches Three main classes far can we go for Tanzania? RCM Hi-res GCM 1. New downscaling Weather Generators Transfer Functions Index / analogues Trained on long term Trained on time series Requires long term data time series and that spans range of sets and uses weather atmospheric re-analysis variability, and typing or historical data atmospheric re-analysis analogues data Source: Conditioned by GCM Residual local scale Bruce Hewitson parameters to capture variance added low frequency variance stochastically Santander Meteorology Group VALUE A multidisciplinary approach for weather & climate inventory Reference Country Affiliation Source model(s) Approach1 Technique2 Var Region TAO3 Landman and Tennant S. Africa S. Africa Weather Bureau COLA GCM MOS-E G-D (CCA) P South Africa M SPECS D52.1 (2000) Robertson et al. (2004) USA Columbia University ECHAM 4.5 MOS-D G-S (HMM) P Brazil D D´ıez et al. (2005) Spain National Institute of Meteo- DEMETER (2 models) PP-E (ERA-40) NG-D (Analogs) P Spain D rology Pavan et al. (2005)Santander Italy Meteorology ARPA-SIMC Group DEMETER (6 models) PP-E (ERA-40) G-D (MLR) T, P Italy M Gutierrez´ et al. (2005) A multidisciplinary Spain University approach of Cantabria for weather DEMETER & climate (4 models) PP-E (ERA-40) NG-D (Analogs) P Northern Peru S Fr´ıas et al. (2005) Spain University of Cantabria DEMETER (7 models) PP-E (ERA-40, NNR) G-D (CCA) T Iberian Peninsula M Feddersen and Andersen Denmark Danish Meteorological In- DEMETER MOS-E G/NG-D (SVD+WT) P, T Europe, N America, S (2005) stitute Australia Chu et al. (2008) Taiwan National Taiwan Normal SMIP (6 GCMs) MOS-E G-D (SVD) P N Taiwan S University Sordo et al. (2008) Spain University of Cantabria ECMWF’s System2 PP-E (ERA-40) NG-D (Analogs) P Spain D Landman et al. (2009) S. Africa South African Weather Ser- ECHAM4.5 MOS-E G-D (CCA) P S Africa S vice Juneng et al. (2010) Malaysia Universiti Kebangsaan APCC-MME (7 models) MOS-E G-D (CCA) P Malaysia M Fr´ıas et al. (2010) Spain University of Cantabria DEMETER PP-E (ERA-40) NG-D (Analogs) P, T Spain D Min et al. (2011) S. Korea APEC Climate Center APCC MME (6 models) MOS-E G-D (LR) P, T S Korea S Wu et al. (2012) USA NCAR CFS MOS-E NG-D (Analogs-KNN) P SE Mediterranean M Sun and Chen (2012) China Institute of Atmospheric DEMETER (7 models ) MOS-E G-D (LR) P Global (CRU data) S Physics Kryzhov (2012) Russia Hydrometeorological Re- SLAV GCM MOS-E G-D (LR) T N Eurasia M search Center Robertson et al. (2012) USA International Research Insti- RegCM3 / ECHAM4.5 MOS-E G-D (PC-LR) P Philippines D Tech. Notes Santander Meteorology Group (CSIC-UC): tute for Climate and Society Ying and Ke (2012) China Institute of Atmospheric DEMETER (3 models) PP-E (ERA-40) G-D (LR) P SE China S Physics Johnson (2012) USA Florida State University DEMETER (and others) MOS-E G-D (LR) P S America M Tian and Martinez (2012) USA University of Florida GFS / DOE PP-E (NARR) NG-D (Analogs) ET0 Florida D Shao and Li (2013) Australia CSIRO POAMA PP-E (NNR) NG-D (Analogs) P SE Australia D Sinha et al. (2013) India Indian Institute of Technol- In-GLM1 (NCMRWF) PP-E (NNR) G-D (CCA) P India S ogy de Castro et al. (2013) Brazil Federal University of Ceara RSM / ECHAM4.5 MOS-E G-D (ANN) P Brazil M Charles et al. (2013) Australia Bureau of Meteorology POAMA MOS-E NG-D (Analogs) P SE Australia D Sohn et al. (2013) Korea APCC APCC MME (10 models) MOS-E G-D (LR) P S Korea M Silva and Mendes (2013) Brazil University Federal of Rio CFS MOS-E G-D (ANN) P NE Brazil M Grande do Norte Tung et al. (2013) China City University of Hong APCC MME MOS-E G-D (SVD) P S China S Kong Pavan and Doblas-Reyes Italy ARPA-SIMC ENSEMBLES (5 models) MOS-E G-D (MLR) T Italy M GMS:03.2013;1–12 (2013) Table 2: Summary table of previous studies applying any form of statistical downscaling to seasonal forecast products. Note that the country and affiliation fields are for the corresponding author. The name of a RCM followed by a “/ ” indicates the RCM/GCM coupling (e.g. RegCM3/ECHAM4.5). 1Type of approach according to the classification of Table 1 [MOS=model output statistics, PP=perfect-prog and subtypes: E=eventwise, D=distribution]. For the PP methods, the reanalysis used is indicated in parenthesis. 2Type of technique according to the classification of 3 Table 1 [G=generative, NG=non-generative and subtypes: S=stochastic, D=deterministic]. The particular technique used is indicated in parenthesis. TAO = Temporal aggregation of the output 6 [D=daily, M=monthly, S=seasonal]. Sources: ISI Web of Knowledge, SCOPUS and google scholar. The acronyms used in this table are described in Sec. 3.1. SPECS D52.1 5 2.3 Classification Framework SPECS D52.1 5 Building upon the above classification scheme according to the technique used and the approach followed in the training phase,2.3 Classification we propose the conceptual Framework classification framework shown in Table 1, for the different downscaling variants. In particular, besides considering the downscaling approach, we explicitly differentiate those eventwise circulation-driven SD methods, which are calibrated at a time-series level (i.e. preserving the temporal match- ing —e.g.Building day–to–day upon the correspondence— above classification scheme between according the global to the technique predictorsused and and the the correspondingapproach followed local in the downscaled training phase, we propose the conceptual classification framework shown in Table 1, for the different downscaling predictands),variants. from In particular, those climatology-oriented besides considering the SD downscaling methods, approach, calibrated we explicitly at a PDF differentiate (or CDF) those level,eventwise which do not take intocirculation-driven account the temporal SD methods, correspondence which are calibrated between at a time-seriespredictors level and (i.e. predictands. preserving the To temporal this aim, match- we consider a sub-classificationing —e.g. day–to–day of the above correspondence— approaches betweenas distribution the global (labelled predictors as andDistribution the corresponding) and local time-series downscaled (labelled as eventwisepredictands),) calibrated from ones. those climatology-oriented SD methods, calibrated at a PDF (or CDF) level, which do not take into account the temporal correspondence between predictors and predictands. To this aim, we consider a sub-classification of the above approaches as distribution (labelled as Distribution) and time-series (labelled as Moreover,eventwise regarding) calibrated the ones. two types of downscaling techniques (generative and non-generative), can be either deterministic or stochastic. For the generative case, the stochastic methods have the advantage of intrinsically rep- resenting theMoreover, unexplained regarding local the variance two types ofwhich downscaling is usually techniques under-represented (generative and bynon-generative the deterministic), can be eithermodels.
Details
-
File Typepdf
-
Upload Time-
-
Content LanguagesEnglish
-
Upload UserAnonymous/Not logged-in
-
File Pages34 Page
-
File Size-