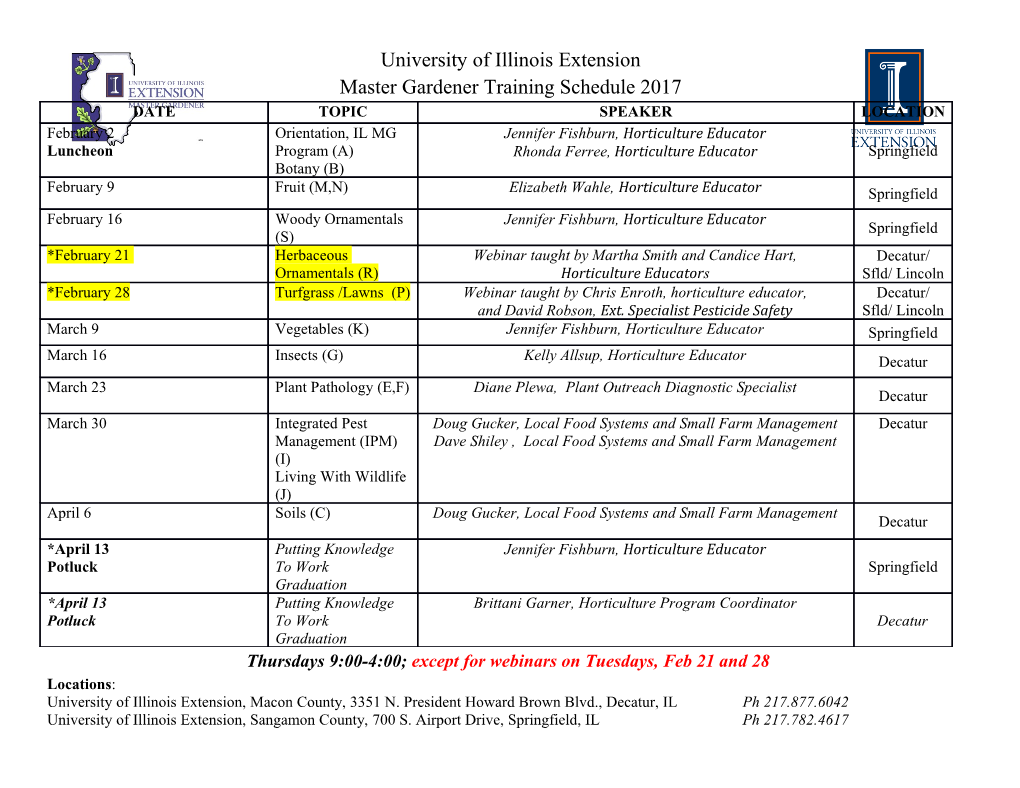
Sferra et al. BMC Bioinformatics (2017) 18:396 DOI 10.1186/s12859-017-1815-5 SOFTWARE Open Access Phylo_dCor: distance correlation as a novel metric for phylogenetic profiling Gabriella Sferra, Federica Fratini, Marta Ponzi and Elisabetta Pizzi* Abstract Background: Elaboration of powerful methods to predict functional and/or physical protein-protein interactions from genome sequence is one of the main tasks in the post-genomic era. Phylogenetic profiling allows the prediction of protein-protein interactions at a whole genome level in both Prokaryotes and Eukaryotes. For this reason it is considered one of the most promising methods. Results: Here, we propose an improvement of phylogenetic profiling that enables handling of large genomic datasets and infer global protein-protein interactions. This method uses the distance correlation as a new measure of phylogenetic profile similarity. We constructed robust reference sets and developed Phylo-dCor, a parallelized version of the algorithm for calculating the distance correlation that makes it applicable to large genomic data. Using Saccharomyces cerevisiae and Escherichia coli genome datasets, we showed that Phylo-dCor outperforms phylogenetic profiling methods previously described based on the mutual information and Pearson’s correlation as measures of profile similarity. Conclusions: In this work, we constructed and assessed robust reference sets and propose the distance correlation as a measure for comparing phylogenetic profiles. To make it applicable to large genomic data, we developed Phylo-dCor, a parallelized version of the algorithm for calculating the distance correlation. Two R scripts that can be run on a wide range of machines are available upon request. Keywords: Phylogenetic profiling, Distance correlation, Protein-protein interaction Background to gain insights on the function of uncharacterized pro- In the last two decades, several computational ap- teins [see for example [6–8]. These methods are based proaches have been proposed to infer both functional on the detection of orthologs either from sequence simi- and physical protein-protein interactions (PPIs). These larity score or from tree-based algorithms (for a recent methods includes the identification of gene fusion events implementation see [9]). [1, 2], conservation of gene neighborhood [3] or phylo- In general, phylogenetic profiling is based on the as- genetic profiling [4, 5]. Recently, the increasing number sumption that proteins involved in the same biological of fully sequenced genomes led to a renewed interest in pathway or in the same protein complex co-evolve [for a these approaches. Among them, the phylogenetic profil- review see [10]. In a first implementation [4], the phylo- ing is one of the most promising in that it allows to pre- genetic profile of a protein was defined as a binary vec- dict protein-protein interactions at a whole genome tor that describes the occurrence pattern of orthologs in level, while gene fusion and gene neighborhood are rela- a set of fully sequenced genomes, and the Hamming dis- tively rare events found typically in prokaryotic tance was used to score the similarity between profile genomes. pairs. Subsequently, to evaluate different degrees of se- Well implemented methods, based on phylogenetic quence divergence, phylogenetic profiles were recon- profiling, have been developed and successfully applied structed using probabilities derived by the expectation for understanding relationships between proteins and/or values obtained aligning the proteins under study with a genome reference set [5]. Among measures proposed to * Correspondence: [email protected] score the phylogenetic profile similarities [for a review Dipartimento di Malattie Infettive, Parassitarie e Immunomediate, Istituto Superiore di Sanità, Viale Regina Elena 299, 00161 Rome, Italy see [11], the Mutual Information (MI) was demonstrated © The Author(s). 2017 Open Access This article is distributed under the terms of the Creative Commons Attribution 4.0 International License (http://creativecommons.org/licenses/by/4.0/), which permits unrestricted use, distribution, and reproduction in any medium, provided you give appropriate credit to the original author(s) and the source, provide a link to the Creative Commons license, and indicate if changes were made. The Creative Commons Public Domain Dedication waiver (http://creativecommons.org/publicdomain/zero/1.0/) applies to the data made available in this article, unless otherwise stated. Sferra et al. BMC Bioinformatics (2017) 18:396 Page 2 of 7 to correlate well in accuracy with genome-wide yeast −∑∑p(a, b)lnp(a, b)represents the summation of the two-hybrid screens or mass spectrometry interaction as- relative entropies of the joint probability distribution says [5]. Although it was largely adopted as a measure of p(a, b)of co-occurrence of gene A and B across the phylogenetic profile similarity, Simon and Tibshirani re- set of reference genomes, in the intervals of the prob- cently debated about the lower power of MI in detecting ability distribution. The mutual information was cal- dependency between two variables compared with cor- culated by using the mutualInfo function available in relation measures [12]. bioDist R package [16] after binning the data into 0.1 In this work, we propose the distance correlation intervals. (dCor) as a novel metric to score phylogenetic profile We calculated dCor according to Szekely and collabo- similarity. dCor measures any dependence between two rators [13, 14]. The original implementation (available in variables, ranges between 0 and 1, and it satisfies all re- the energy package of Bioconductor) allows the calcula- quirements of a distance [13, 14]. tion only between two arrays of data. For this reason, we In order to apply this measure to large genomic data, developed two novel scripts that make possible to per- we developed a novel parallel version of the original al- form dCor NxN phylogenetic profile comparison, where gorithm. Furthermore, we adopted a new strategy of N is the number of genes in a given genome. In genome selection to obtain unbiased and large reference principle, the method is applicable also to binary phylo- sets of genomes. We applied this methodology to con- genetic profiles. struct phylogenetic profiles of two model organisms, First, the matrix of the Euclidean distances was ob- Escherichia coli and Saccharomyces cerevisiae and con- tained calculating the difference between thek-th firmed that correlation measures (dCor and Pearson’s element and thel-th element of the phylogenetic pro- correlation) have a more robust predictive perform- file as ance than the MI. In particular we showed that dCor performs better than Pearson’s correlation (PC) and D ¼ ⌊dkl⌋ MI especially in predicting physical protein-protein interactions. where. dkl =|ak − al|r as the distance between the r-th pairs of Implementation elements of the profiles. d D Phylogenetic profiling Second, each distance kl of the matrix was then da Phylogenetic profiles were obtained as arrays of pro- converted into an element kl of the matrix of the cen- DA bability values according to tered distances , calculated as ¼ − = ðÞ dakl ¼ dkl−dk −dl þ dkl P 1 log10 E − For E-values higher than 10 1, the probability value is where. Pn set to 1, as proposed in [5]. 1 dk ¼ n dkl is the average calculated on the rows of Where E are the E-values obtained from the align- k¼1 ments of S. cerevisiae and E. coli protein sequences the distance matrix; Pn against the four reference sets. To do this, we applied 1 dl ¼ n dkl is the average calculated on the columns the Smith-Watermann alignment algorithm [15]. The l¼1 FASTA package version 36 was implemented as a of the distance matrix; Pn stand-alone software on two Work Stations both dual d ¼ 1 d kl n2 kl is the average calculated on all the ele- core, the first with 12 CPU and the second with 8 k;l¼1 CPU. ments of the distance matrix; where k = l = 1,.…, n =1,…, j. Similarity measures The distance correlation between the profilesApandAq One of the method usually used to establish similarity was calculated as between phylogenetic profiles is the mutual information that is calculated according to CovðDAp; DAqÞ dCorpq ¼ qffiffiffiffiffiffiffiffiffiffiffiffiffiffiffiffiffiffiffiffiffiffiffiffiffiffiffiffiffiffiffiffiffiffiffiffiffiffiffiffiffiffiÀÁÀÁ MIðÞ¼ A; B HAðÞþHBðÞ−HAðÞ; B Var DAp Var DAq where H(A)= −∑p(a)lnp(a) is the summation of the where Cov and Varrepresent the covariance and the marginal entropies, calculated over the intervals of variance of the matrices of the centered distances and p probability distribution p(a), of the gene A to occur = q =1, … , i. among the organisms in the reference set. H(A, B)= Pearson’s correlation was calculated according to Sferra et al. BMC Bioinformatics (2017) 18:396 Page 3 of 7 P n ðÞx −x ðÞy −y 1133 manually selected genomes were collected and PC ¼ qffiffiffiffiffiffiffiffiffiffiffiffiffiffiffiffiffiffiffiffiffiffiffiffiffiffiffiffiffiffiffiffiffiffiffiffiffiffiffiffiffiffiffiffiffiffiffiffiffiffiffiffiffiffii¼1 i i P pPffiffiffiffiffiffiffiffiffiffiffiffiffiffiffiffiffiffiffiffi classified as “core” (high quality genomes) and “per- n ðÞx −x n y −yÞ i¼1 i 2 i¼1 i 2 ipheral” (genomes not completely validated) on the Where n is the size of the two arrays x and y, and x y basis of genome coverage, status of gene annotation and are the corresponding means. and gene completeness. The first reference set (RS1) excluded all the strains of Gold standards and predictive performance assessment the same species classified as “peripheral” genomes. A On the basis of KEGG database [17], we considered pro- second reference set (RS2) was generated from RS1 ex- teins belonging to the same metabolic pathway as func- cluding the eukaryotic genomes with a “peripheral” attri- tional related and hence to be included in the True bute till having 45 eukaryotic genomes in a such way to Positive data set (TP-fun). To derive the True Negative pass from a ratio 5:1 to a ratio 13:1. To construct the data set (TN-fun), we developed a graph-based algo- third reference set (RS3), we progressively excluded “per- rithm to identify non-interacting proteins.
Details
-
File Typepdf
-
Upload Time-
-
Content LanguagesEnglish
-
Upload UserAnonymous/Not logged-in
-
File Pages7 Page
-
File Size-