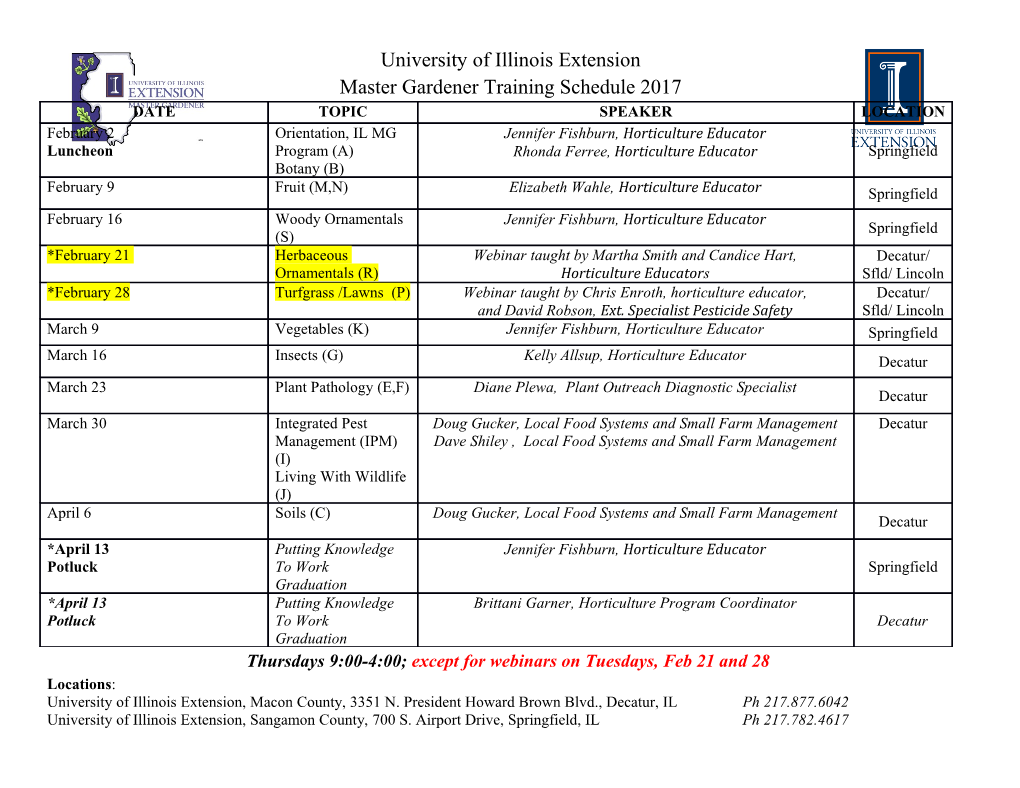
ABSTRACT Title of dissertation: COMPUTATIONAL METHODS TO IMPROVE GENOME ASSEMBLY AND GENE PREDICTION David Kelley, Doctor of Philosophy, 2011 Dissertation directed by: Professor Steven Salzberg Department of Computer Science DNA sequencing is used to read the nucleotides composing the genetic material that forms individual organisms. As 2nd generation sequencing technologies offering high throughput at a feasible cost have matured, sequencing has permeated nearly all areas of biological research. By a combination of large-scale projects led by consortiums and smaller endeavors led by individual labs, the flood of sequencing data will continue, which should provide major insights into how genomes produce physical characteristics, including disease, and evolve. To realize this potential, computer science is required to develop the bioinformatics pipelines to efficiently and accurately process and analyze the data from large and noisy datasets. Here, I focus on two crucial bioinformatics applications: the assembly of a genome from sequencing reads and protein-coding gene prediction. In genome assembly, we form large contiguous genomic sequences from the short sequence fragments generated by current machines. Starting from the raw sequences, we developed software called Quake that corrects sequencing errors more accurately than previous programs by using coverage of k-mers and probabilistic modeling of sequencing errors. My experiments show correcting errors with Quake improves genome assembly and leads to the detection of more polymorphisms in re- sequencing studies. For post-assembly analysis, we designed a method to detect a particular type of mis-assembly where the two copies of each chromosome in diploid genomes diverge. We found thousands of examples in each of the chimpanzee, cow, and chicken public genome assemblies that created false segmental duplications. Shotgun sequencing of environmental DNA (often called metagenomics) has shown tremendous potential to both discover unknown microbes and explore com- plex environments. We developed software called Scimm that clusters metagenomic sequences based on composition in an unsupervised fashion more accurately than previous approaches. Finally, we extended an approach for predicting protein-coding genes on whole genomes to metagenomic sequences by adding new discriminative features and augmenting the task with taxonomic classification and clustering of the sequences. The program, called Glimmer-MG, predicts genes more accurately than all previous methods. By adding a model for sequencing errors that also allows the program to predict insertions and deletions, accuracy significantly improves on error-prone sequences. COMPUTATIONAL METHODS TO IMPROVE GENOME ASSEMBLY AND GENE PREDICTION by David Kelley Dissertation submitted to the Faculty of the Graduate School of the University of Maryland, College Park in partial fulfillment of the requirements for the degree of Doctor of Philosophy 2011 Advisory Committee: Professor Steven Salzberg, Chair/Advisor Professor James Yorke Professor Mihai Pop Professor Carl Kingsford Professor H´ectorCorrado Bravo Acknowledgments First and foremost, I acknowledge my thesis advisor Steven Salzberg. His patience while I learned how to do research in computational biology and support as I developed and pursued my own research ideas have had a significant positive impact on my career. Carl Kingsford, Mihai Pop, and Art Delcher also influenced my development as a computational biologist. I am particularly grateful to Dr. Kingsford for encour- aging me to join his group meetings focused on networks in computational biology, which has expanded my knowledge of the field. My officemates for the majority of my graduate career | Michael Schatz, Adam Phillippy, Cole Trapnell, Saket Navlakha, James White, and Ben Langmead | helped make graduate school incredibly enjoyable. Furthermore, they guided me by their experiences and transformed me from a book smart computer scientist to a more practically skilled one. Many other friends and colleagues at CBCB deserve recognition as well. While pursuing my undergraduate degree at Syracuse University, I reached out for a senior thesis collaboration and stumbled into a wonderful experience working with biology Professors Scott Pitnick and Al Uly. Drs. Pitnick and Uly guided me through my first true research project and convinced me to continue on to graduate school to study biology. But the most long-standing and important influence on my life and career has come from my family. My parents Robert Kelley and Janice Collins pushed me to ii be my best and imparted the value of a technical education. They have been loving, encouraging, supportive, and invaluable at the major decision points in my life. My two brothers and extended family have also been a great influence. And finally, I am grateful to my loving girlfriend Amy Sawin who supports my hard work and brings much needed balance to my life. iii Table of Contents List of Tables vi List of Figures vii 1 Background 1 1.1 Genome assembly . .3 1.2 Gene prediction . .7 1.3 2nd-generation sequencing . .9 1.4 Metagenomics . 12 1.5 Summary . 12 2 Quake: quality-aware detection and correction of sequencing errors 15 2.1 Rationale . 15 2.2 Results and Discussion . 20 2.2.1 Accuracy . 20 2.2.2 Genome assembly . 24 2.2.3 SNP detection . 30 2.2.4 Data quality . 33 2.3 Conclusions . 35 2.4 Methods . 39 2.4.1 Counting k-mers . 40 2.4.2 Coverage cutoff . 42 2.4.3 Localizing errors . 44 2.4.4 Sequencing error probability model . 46 2.4.5 Correction search . 48 2.5 List of abbreviations . 52 2.6 Acknowledgements . 52 3 Detection and correction of false segmental duplications caused by genome mis-assembly 53 3.1 Background . 54 3.2 Results and Discussion . 57 3.2.1 Genomes . 57 3.2.2 Use of the human genome to check duplications . 61 3.2.3 Coverage depth . 62 3.2.4 Genes affected by erroneous duplications . 64 3.2.5 Unplaced contigs . 66 3.2.6 SNPs and indels . 67 3.3 Conclusions . 67 3.4 Methods . 71 3.4.1 Detection of potential haplotype mis-assemblies . 72 3.4.2 Analysis of mate pairs . 73 3.4.3 Unplaced contigs . 78 iv 3.4.4 Haplotype polymorphisms . 79 3.5 List of abbreviations . 83 3.6 Acknowledgements . 84 4 Clustering metagenomic sequences with interpolated Markov models 85 4.1 Background . 86 4.2 Methods . 89 4.2.1 Interpolated Markov models . 90 4.2.2 K-means clustering framework . 92 4.2.3 Scimm ............................... 95 4.2.4 Initial partitioning . 96 4.2.5 Supervised initial partitioning . 98 4.3 Results and Discussion . 99 4.3.1 Simulated reads . 99 4.3.2 FAMeS . 105 4.3.3 In vitro-simulated metagenome . 109 4.4 Conclusions . 111 4.5 Acknowledgements . 114 5 Gene prediction with Glimmer for metagenomic sequences augmented by classification and clustering 115 5.1 Introduction . 116 5.2 Methods . 120 5.2.1 Glimmer . 120 5.2.2 Additional features . 120 5.2.3 Truncated length model . 123 5.2.4 Classification . 127 5.2.5 Clustering . 129 5.2.6 Sequencing errors . 131 5.2.7 Whole genomes . 135 5.2.8 Simulated metagenomes . 136 5.2.9 Accuracy . 138 5.3 Results . 139 5.3.1 Whole genomes . 139 5.3.2 Simulated metagenomes - perfect reads . 140 5.3.3 Simulated metagenomes - error reads . 144 5.3.4 Real metagenomes . 147 5.4 Conclusion . 148 5.5 Acknowledgements . 149 6 Conclusion 150 Bibliography 152 v List of Tables 2.1 E. coli 36bp ............................... 22 2.2 E. coli 124 bp . 23 2.3 Velvet E. coli assembly . 27 2.4 SOAPdenovo bee assembly . 28 2.5 E. coli SNPs . 32 3.1 Erroneous duplications . 59 3.2 Unplaced haplotype variants . 66 3.3 SNP prior comparison . 81 4.1 Varying read length . 102 4.2 Varying error rate . 103 5.1 Accuracy on whole genomes . 140 5.2 Accuracy on simulated metagenomes with perfect reads . 142 5.3 Accuracy on simulated metagenomes with error read . 144 vi List of Figures 1.1 Shotgun sequencing . .2 1.2 Overlap graph . .4 1.3 Assembly graph with repeats . .6 1.4 Sequencing cost . 11 2.1 Alignment difficulty . 25 2.2 Adenine error rate . 34 2.3 K-mer coverage . 43 2.4 Localize errors . 45 2.5 Pseudocode . 49 2.6 Correction search . 50 3.1 Mis-assembled DCC and DOC . 58 3.2 Erroneous duplication lengths . 60 3.3 Chimpanzee Contig412.192 . 61 3.4 SCPEP1 . ..
Details
-
File Typepdf
-
Upload Time-
-
Content LanguagesEnglish
-
Upload UserAnonymous/Not logged-in
-
File Pages177 Page
-
File Size-