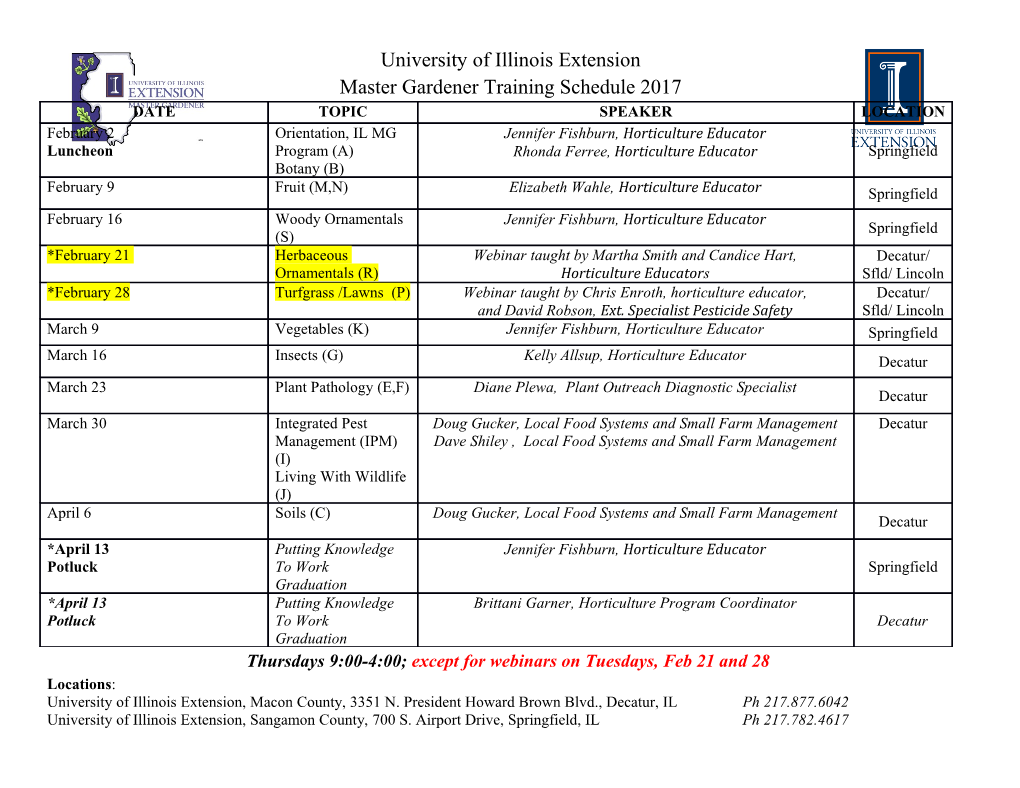
FEEG6017 lecture: Time series analysis, autocorrelation [email protected] Time series analysis • The basic idea of time series analysis is simple: given an observed sequence, how can we build a model that can predict what comes next? • Obvious applications in finance, business, ecology, agriculture, demography, etc. What's different about time series? • In most of the contexts we've seen so far, there's an implicit assumption that observations are independent of each other. • In other words, the fact that subject 27 is 165cm tall and terrible at basketball says nothing at all about what will happen with subject 28. What's different about time series? • In time series data, this is not true. • We're hoping for exactly the opposite: that what happens at time t contains information about what will happen at time t+1. • Observations are treated as both outcome and then predictor variables as we move forward in time. Ways of dealing with time series • Despite (or perhaps because of) the practical uses of time series, there is no single universal technique for handling them. • Lots of different ways to proceed depending on the implicit theory of data generation we're proposing. • Easiest to illustrate with examples... Example 1: Lake Huron data • Our first example data set is a series of annual measurements of the level of Lake Huron, in feet, from 1875 to 1972. • It's a built-in data set in R. So we only need data(LakeHuron) to access it. • R already "knows" that this is a time series. Example 1: Lake Huron data Ex. 2: Australian beer production • Our second example is data on monthly Australian beer production, in millions of litres. • The time series runs from January 1956 to August 1995. • The data is available in beer.csv. Ex. 2: Australian beer production • R doesn't yet know that this is a time series: the data comes in as a list of numbers. • We use the ts function to specify that something should be interpreted as a time series, optionally specifying the seasonal period. • beer = ts(beer[,1],start=1956,freq=12) Ex. 2: Australian beer production Two goals in time series modelling • We assume there's some structure in the time series data, obscured by random noise. • Structure = trends + seasonal variation + noise • The Lake Huron data has no obvious repetitive structure, but possibly a downward trend. The beer data shows clear seasonality and a trend. • Structure = Trend + Cycle + Season + Error Models of data generation • The most basic of data generation is to suppose that there is no structure in the time series at all, and that each observation is an independent random variate. • An example: white noise. • In this case, the best we can do is simply predict the mean value of the data set. Lake Huron: prediction if observations were independent Beer production: prediction if observations were independent Producing these graphs in R png("BeerMeanPredict.png",width=800,height=400) plot(beer,xlim=c(1956,2000),lw=2,col="blue") lines(predict(nullBeer,n.ahead=50)$pred, lw=2,col="red") lines(predict(nullBeer,n.ahead=50)$pred +1.96*predict(nullBeer,n.ahead=50)$se, lw=2,lty="dotted",col="red") lines(predict(nullBeer,n.ahead=50)$pred -1.96*predict(nullBeer,n.ahead=50)$se, lw=2,lty="dotted",col="red") graphics.off() Simple approach to trends • We could ignore the seasonal variation and the random noise and simply fit a linear or polynomial model to the data. • Make predictors: tb = seq(1956,1995.8,length=length(beer)) • Linear: linearBeer = lm(beer ~ tb) • Polynomial: polyBeer = lm(beer ~ tb + tb^2) Polynomial fit of lake level on time Polynomial fit of beer production on time Regression on time a good idea? • This is an OK start: it gives us some sense of what the trend line is. • But we probably don't believe that beer production or lake level is a function of the calendar date. • More likely these things are a function of their own history, and we need methods that can capture that. Autoregression • A better approach is to ask whether the next value in the time series can be predicted as some function of its previous values. • This is called autoregression. • We want to build a regression model of the current value fitted on one or more previous values (lagged values). But how many? Autocorrelation and partial autocorrelation • We can look directly at the time series and ask how much information there is in previous values that helps predict the current value. • The acf function looks at the correlation between now and various points in the past. • Partial autocorrelation(pacf) does the same, but "partials out" the other effects to get the unique contribution of each time-lag. ACF & PACF, Lake Huron data ACF & PACF, beer data ACF & PACF plots • ACF shows a correlation that fades as we take longer lagged values in the Lake Huron time series. • ACF shows periodic structure in the beer time series reflecting its seasonal nature. ACF & PACF plots • But if t[0] is correlated with t[-1], and t[-1] is correlated with t[-2], then t[0] will necessarily be correlated with t[-2] also. • So we need to look at the PACF values. • We find that only the most recent value is really useful in building an autoregression model for the Lake Huron data, for example. Autoregression models • With the ar command we can fit autoregression models and ask R to use AIC to decide how many lagged values should be included in the model. • For example: arb = ar(beer) • The Lake Huron model includes only one lagged value; the beer model includes 24. Autoregression model, lake data, 1 lagged term Autoregression model, beer data, 24 lagged terms Automatically separating trends, seasonal effects, and noise • The stl procedure uses locally weighted regression to separate out a trend line, and parcels out the seasonal effect. • For example: plot(stl(beer,s.window="periodic"), col="blue",lw=2) • If things go well, there should be no autocorrelation structure left in the residuals. Exponential smoothing • A reasonable guess about the next value in a series is that it would be an average of previous values, with the most recent values weighted more strongly. • This assumption constitutes exponential smoothing: 2 t0 = α t-1 + α(1-α)t-2 + α(1-α) t-3 ... Holt-Winters procedure • The logic can be applied to the basic level of the prediction, to the trend term, and to the seasonal term. • The Holt-Winters procedure automatically does this for all three; for example: HWB = HoltWinters(beer) Holt-Winters analysis on beer data Holt-Winters analysis on lake data • The process seems to work well with the seasonal beer data. • For the lake data, we have not specified a seasonal period, and we might also drop the trend term, thus: • beta = trend • gamma = season HWLake = HoltWinters(LakeHuron,gamma=FALSE,beta=FALSE) Holt-Winters analysis on lake data Holt-Winters analysis on lake data • The fitted alpha value is close to 1 (i.e., a very short memory) so the prediction is that the process will stay where it was. • What if we put the trend term back in? • Implicitly beta = trend =TRUE • gamma=seasonal=FALSE HWLake = HoltWinters(LakeHuron,gamma=FALSE) Holt-Winters analysis on lake data • Trend is overdoing it (beta = 0.17)? Differencing • Some time series techniques (e.g., ARIMA) are based on the assumption that the series is stationary, i.e., that it has constant mean, variance, and autocorrelation values over time. • If we want to use these techniques we may need to work with the differenced values rather than the raw values. Differencing • This just means transforming t[1] into t[1] - t[0], etc. • We can use the diff command to make this easy. • To plot the beer data as a differenced series: plot(diff(beer),lw=2,col="green") Differencing Some housekeeping in R • To get access to some relevant ARIMA model fitting functions, we need to download the "forecast" package. • install.packages("forecast") library(forecast) Auto-regressive integrated moving- average models (ARIMA) • ARIMA is a method for putting together all of the techniques we've seen so far. • A non-seasonal ARIMA model is specified with p, d, and q parameters. • p: no. of autoregression terms. d: no. of difference levels. q: no. of moving-average (smoothing) terms. Auto-regressive integrated moving- average models (ARIMA) • ARIMA(0,0,0) is simply predicting the mean of the overall time series, i.e., no structure. • ARIMA(0,1,0) works with differences, not raw values, and predicts the next value without any autoregression or smoothing. This is therefore a random walk. • ARIMA(1,0,0) and ARIMA(24,0,0) are the models we originally fitted to the lake and beer data. Auto-regressive integrated moving- average models (ARIMA) • We can also have seasonal ARIMA models: three more terms apply to the seasonal effects. • The "forecast" library includes a very convenient auto.arima function that uses AIC to find the most parsimonious model in the space of possible models. ARIMA(1,1,2) model of lake data ARIMA(2,1,2)(2,0,0)[12] model of beer data Fourier transforms • No time to discuss Fourier transforms... • But they're useful when you suspect there are seasonal or cyclic components in the data, but you don't yet know the period of these components. • In the beer example, we already knew the seasonal period was 12, of course. Additional material • The beer.csv data set. • The R script used to do the analyses.
Details
-
File Typepdf
-
Upload Time-
-
Content LanguagesEnglish
-
Upload UserAnonymous/Not logged-in
-
File Pages48 Page
-
File Size-