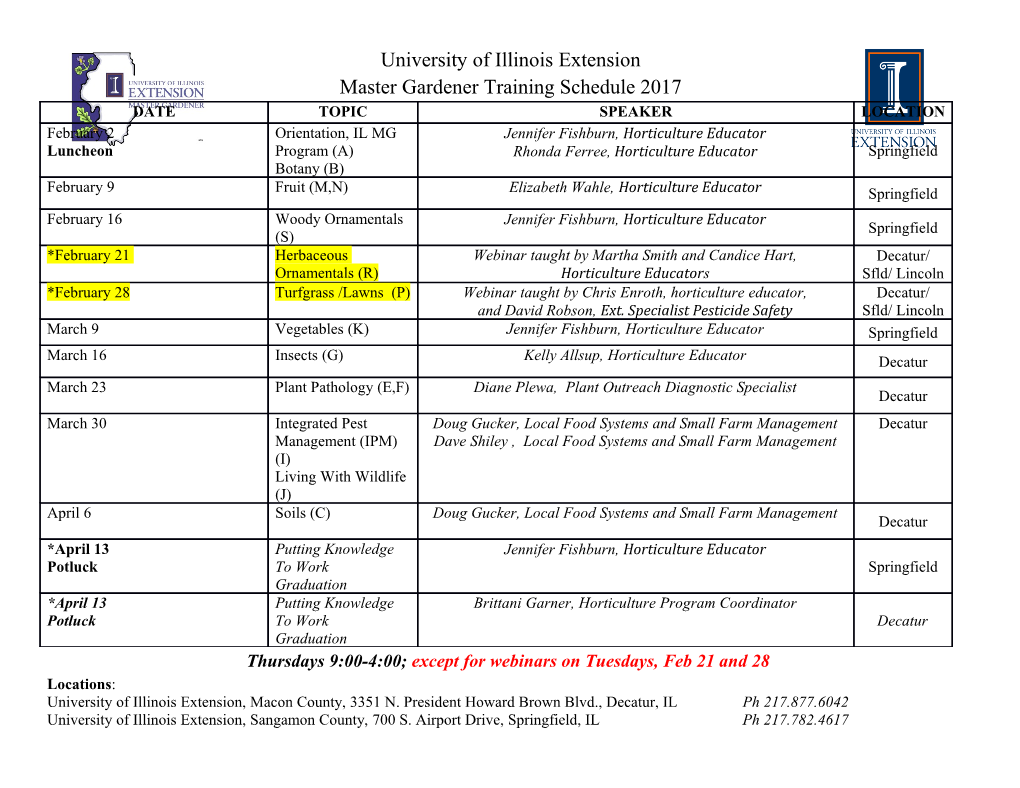
Modelling primary production dynamics in the Arctic under changing sea ice conditions Alice Stuart-Lee University of Utrecht Supervisors : Jack Middelburg - University of Utrecht Karline Soetaert - Royal Netherlands Institute for Sea Research © 2017, A. E. Stuart-Lee Department of Earth Sciences Faculty of Geosciences Utrecht University Abstract A dramatic decline in sea ice is underway in the Arctic and we have reason to anticipate complete summer melting within this half of the century. With our current understanding of the ecological effects of this change limited, and Earth system models not yet accounting for sympagic (sea ice ecosystem) primary production, this study has been motivated to contribute towards efforts to effectively model this system. With the aim of investigating the role of sea ice in the primary production dynamics in the Arctic, an existing nutrient-phytoplankton-zooplankton-detritus (NPZD) biogeochemical model has been extended to include a parameterised ice module and sympagic primary production via an ice algae variable. This model revealed a picture of how the modelled ice algae, phytoplankton and zooplankton entities fared under varying levels of ice cover, providing a potential progression of the basic bloom dynamics in pelagic-sympagic ecosystems in which total productivity increases with reduced ice cover. Sensitivity analysis has been conducted, with considerations offered for further tests, means of model refinements and potential future developments. Acknowledgements Many thanks to my marvellous supervisors Jack Middelburg and Karline Soetaert. I am very grateful for your time and insight. i Contents List of Figures iv List of Tables iv 1 Introduction 1 2 Biological Background 3 2.1 Sea ice habitat . 3 2.2 Ice algae . 5 2.3 Phytoplankton . 7 2.4 Bloom dynamics . 8 2.5 Benthos . 9 3 Physical Processes 11 3.1 Light . 11 3.2 Temperature . 12 3.3 Salinity . 12 3.4 Mixing . 13 4 Sympagic-Pelagic Ecosystem Models 14 4.1 Example 1: Production on the Canadian Beaufort Sea shelf . 14 4.2 Example 2: Production in the Hudson Bay . 15 4.3 Example 3: Production on the west coast of Greenland . 15 5 Model Design 16 5.1 Physical setup . 16 5.2 Biological activity . 18 5.3 Forcing data . 19 6 Results 21 6.1 Simulation descriptions . 21 6.2 Parameter sensitivity . 27 7 Discussion 29 7.1 Scenario analysis . 29 7.2 Model validation . 30 7.3 Further model considerations . 31 ii 8 Conclusions 33 Bibliography 34 Appendices 41 A Annotated model code . 41 B Model scenario output plots . 49 C Total primary production with time . 55 D Annual state variable flows . 56 E Model scenario ice parameterisations . 57 iii List of Figures 2.1 An observed sympagic-pelagic-benthic ecosystem . 4 2.2 The strand-forming diatom Melosira arctica ....................... 6 2.3 Phytoplankton bloom in the Barents Sea . 9 5.1 Conceptual model diagram . 18 6.1 Summary of scenario 2: seasonal ice . 22 6.2 Summary of scenario 3: multiyear ice . 24 6.3 Scenario 1: model currency flows in a year . 25 6.4 Scenario 2: model currency flows in a year . 26 6.5 Scenario 3: model currency flows in a year . 26 6.6 Total column integrated biomass for one year . 27 B.1 Scenario 1: Icethickness, Light, SedimentOrgN, Mixingcoefficient, NH4br, NO3br . 49 B.2 Scenario 1: NH4, NO3, Phyto, Zoo, Detritus, IceAlgae . 50 B.3 Scenario 2: Icethickness, Light, SedimentOrgN, Mixingcoefficient, NH4br, NO3br . 51 B.4 Scenario 2: NH4, NO3, Phyto, Zoo, Detritus, IceAlgae . 52 B.5 Scenario 3: Icethickness, Light, SedimentOrgN, Mixingcoefficient, NH4br, NO3br . 53 B.6 Scenario 3: NH4, NO3, Phyto, Zoo, Detritus, IceAlgae . 54 C.1 Column integrated biomass with time . 55 List of Tables 5.1 Model parameters . 17 5.2 Mass balance equations . 19 5.3 Rate expressions . 20 6.1 Parameters ranked by sensitivity value . 27 D.1 Total model currency flows between state variables . 56 E.1 Ice parameterisation for scenario 1: ice free . 57 E.2 Ice parameterisation for scenario 2: seasonal ice . 57 E.3 Ice parameterisation for scenario 3: multiyear ice . 57 iv Chapter 1 Introduction Winter satellite coverage of the Arctic between 1979 and 2011 records strongly declining sea ice with respect to both extent (defined as ocean with a minimum ice concentration of 15%) and area. The most rapid rates of decline have been recorded for the thick multiyear component of the ice (-15.6% in extent and -17.5% in area per decade), which has increasingly been replaced by first year ice and thinner ice cover (Comiso, 2011). Further satellite observations from the National Snow and Ice Data Center (NSIDC) show that a new record low was reached in September 2012 for both minimum sea ice extent and sea ice area. These major changes to the Arctic are expected to continue over the coming decades. Complete summer melting is predicted for this half of the century, with projections varying from 2020 with trend extrapolation, to 2040, with alternative methods (Overland and Wang, 2013). These changes to the sea ice have numerous physical feedbacks that are resulting in Arctic ampli- fication, a regional temperature increase greater than the Earth's average. Serreze et al. (2011) outline the most prominent causes of Arctic amplification, which include the ice albedo positive feedback, changing ocean-atmosphere heat fluxes, changing cloud and water vapour cover affecting the longwave radiation flux, and additional soot and other dark aerosols increasing heat absorption. The implication is that not only will the Arctic experience rapid change, but that the rate of change will also continue to rise, with far reaching consequences (Serreze et al., 2011). For ecosystems, these include the loss of habitats for a wide range of life, from the microalgae in the ice to the ringed seals and polar bears using the environment to hunt, forage and reproduce. At the former end of the scale, both past and future changes related to ice algal and pelagic phytoplankton primary productivity are not fully understood. This is in part due to difficulty in quantifying productivity, with a lack of physical research trips due to inhospitable conditions as well as inadequacies with remote sensing techniques. Furthermore, Tedesco et al. (2012) note that regions with changing light conditions over short time periods are not suited to the use of constant Chl:C ratios for the calculation of biomass, and thus that existing estimates for the Arctic region may be biased. One quantification of changing marine primary productivity was made by Arrigo et al. (2008), using an Arctic-specific algorithm with satellite measurements of sea ice, sea surface temperature (SST) and chlorophyll. The result was that annual Arctic primary production increased by a yearly average of 27.5 Tg C yr-1 between 2003 and 2006, and then by 35 Tg C yr-1 1 between 2006 and 2007, mostly as a result of increased open water area and extended phytoplankton growth seasons. Looking to the future, Tedesco et al. (2012) predict that in the areas that maintain seasonal ice, algae may thrive, but that as ice melts earlier, phytoplankton blooms may coincide with lower light conditions and become less productive, and that overall production in these areas may start decreasing at the end of this century. Although it is not clear exactly how Arctic marine production will change over the coming decades, ecosystem disruption appears inevitable. Changes have already been seen to cause mismatches in bloom timings that were previously strongly coupled for efficiency between the ice algae, under-ice phytoplankton and grazing zooplankton, with consequences for the rest of the food web, such as Arctic cod, sea birds, seals and polar bears (Søreide et al., 2010). Motivated by the need for a better understanding of what to expect from primary production dynamics, this project starts with an examination of the biology of the Arctic sea ice and ocean in chapter 2. Existing knowledge of sea ice as a habitat for microorganisms is reviewed, and ice algal life is addressed with a particular emphasis on their adaptation to the particularities of their Arctic environment and the controls on their growth. Their bloom dynamics are explored alongside those of phytoplankton and of zooplankton. Examination of the tight coupling of this ecosystem proceeds by looking into how the surface production affects the benthos. Key processes that control light availability, temperature, salinity and mixing are reviewed in chapter 3 and the primary factors for ecological modelling are investigated. Chapter 4 provides concise and comparative summaries of the biological structure of three existing biogeochemical sympagic-pelagic ecosystem models of varying complexity, where sympagic refers to the ice-based ecosystem. These serve as key examples for the adaption of a biogeochemical model to incorporate ice dynamics and ice algal production, described in chapter 5. Chapters 6 and 7 detail and discuss the output of the model, and conclusions are presented in chapter 8. 2 Chapter 2 Biological Background Although known for its extremes of temperature, salinity and light availability, Arctic ice lends itself to a plethora of life. Not only does the ice provide a habitat for microorganisms and their grazers, the seasonal melting releases this organic matter into the water column below, fuelling further productivity and life in the water and benthos. These sea ice communities and their nearby pelagic and benthic counterparts are reviewed here, with an overview of the factors affecting their growth, bloom and export dynamics. 2.1 Sea ice habitat As ice forms, a large proportion of its salt content is rejected at the ice-water interface, and a vertical convective process arises from this brine rejection whereby the dense water migrates towards the primary drainage channels, with less dense water taking its place (Lake and Lewis, 1970).
Details
-
File Typepdf
-
Upload Time-
-
Content LanguagesEnglish
-
Upload UserAnonymous/Not logged-in
-
File Pages63 Page
-
File Size-