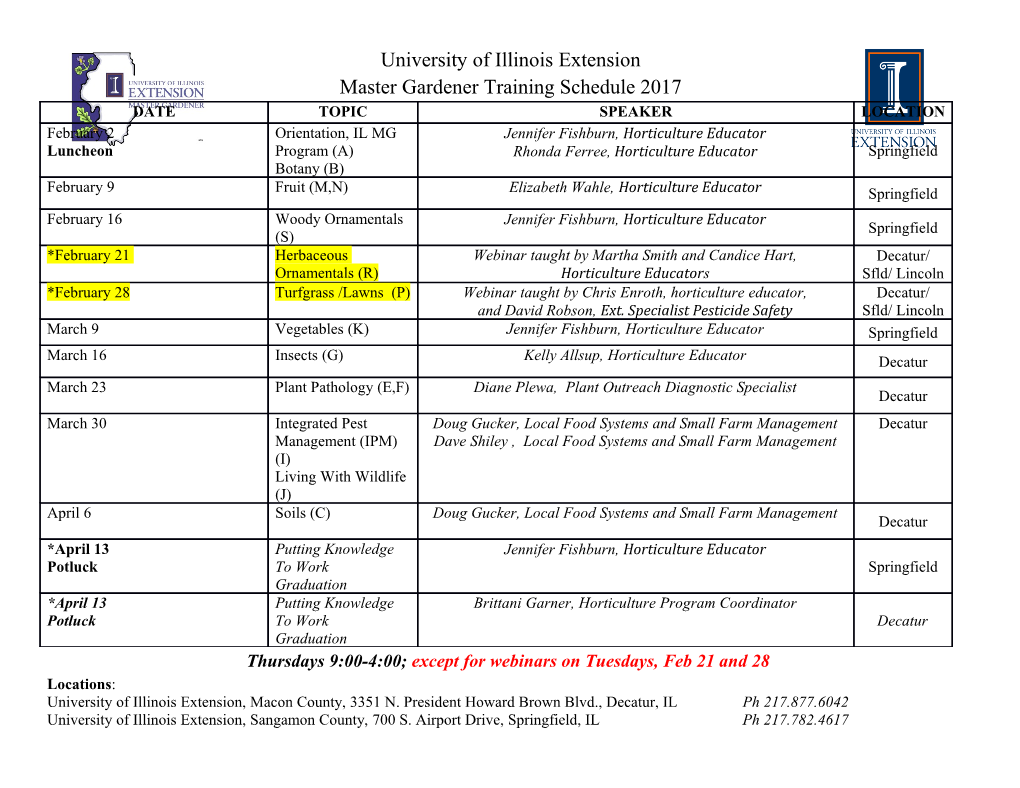
Journal of Neuroscience Methods 184 (2009) 152–160 Contents lists available at ScienceDirect Journal of Neuroscience Methods journal homepage: www.elsevier.com/locate/jneumeth Confounding effects of indirect connections on causality estimation Vasily A. Vakorin a,∗, Olga A. Krakovska b, Anthony R. McIntosh a,c a Rotman Research Institute of Baycrest, Toronto, Canada b Department of Chemistry, York University, Toronto, Canada c Department of Psychology, University of Toronto, Toronto, Canada article info abstract Article history: Addressing the issue of effective connectivity, this study focuses on effects of indirect connections on Received 30 March 2009 inferring stable causal relations: partial transfer entropy. We introduce a Granger causality measure based Received in revised form 9 July 2009 on a multivariate version of transfer entropy. The statistic takes into account the influence of the rest of Accepted 9 July 2009 the network (environment) on observed coupling between two given nodes. This formalism allows us to quantify, for a specific pathway, the total amount of indirect coupling mediated by the environment. Keywords: We show that partial transfer entropy is a more sensitive technique to identify robust causal relations Causality than its bivariate equivalent. In addition, we demonstrate the confounding effects of the variation in Partial transfer entropy Brain connectivity indirect coupling on the detectability of robust causal links. Finally, we consider the problem of model Indirect effects misspecification and its effect on the robustness of the observed connectivity patterns, showing that Model misspecification misspecifying the model may be an issue even for model-free information-theoretic approach. © 2009 Elsevier B.V. All rights reserved. 1. Introduction et al. (1999) are attempts at constructing non-linear versions of the Granger test, remaining within the framefork of linear models. Based on the principles of functional specialization and func- Earlier attempts to address multivariate causality in neuroscience tional integration (Cohen and Tong, 2001), recent studies in trace back to confirmatory methods based on modeling neural neuroscience suggest that behavioral and cognitive functions are activity through autoregressive models. Typically the analysis was implemented in the brain as the specialized and distributed net- performed under the hypothetico-deductive view: how well the works of brain regions (Bressler, 1995). Functional integration in predictions deduced from a hypothesis about causal relations are in turn can be studied under the account of functional and effective accordance with the observed data. Structural equation modeling brain connectivity (Jirsa and McIntosh, 2007; Friston, 1994). Ques- (SEM) is an example of modeling multivariate linear effective tions on how brain anatomy relates to and constrains brain function connectivity within the a priori defined networks (McIntosh and are at the core of functional connectivity, understood as statistical Gonzalez-Lima, 1994). A number of studies elaborated on SEM, dependencies between nodes of a network. However, it is impor- both in the time (Valds-Sosa et al., 2005) and frequency (Blinowska tant not only to reveal the existence of synchronized or temporally et al., 2004) domain. Under this approach, a crucial issue is the correlated systems but also to detect causal pathways. Effective model specification associated with proper selection of covariates, connectivity conceptualized as coherence between spatially remote raising the question how extra variables added to the regression events is an attempt to understand how elements of the interacting can bias the results. At the extreme case, this is related to Simpson’s network are organized dynamically. Inferring causal relationships paradox indicating that statistical relations between variables can is the essential part of the effective connectivity analysis. be reversed by including additional factors into the analysis (Pearl, The dominant position on causality reduces causal relations 2000). to other concepts not involving causal notions. A commonly used Model misspecification is one of the key issues in model- strategy is to reduce causality to predictability. Following the idea ing effective connectivity, linear or non-linear. Schreiber (2000) of one time series predicting another, the Granger test exploits the proposed a non-parametric measure to quantify causal transfer asymmetry in mutual interdependencies between coupled systems entropy, designed as deviation of transition probabilities from the (Granger, 1969). A number of studies analyzed the linear influence Markov property. In essence, introducing transfer entropy was a between brain sites. Locally linear models such as that by Freiwald step towards inferring directionality of coupling between two sys- tems within an information-theoretic approach. This can be viewed as an example of a model-free approach in a sense that no model ∗ Corresponding author. of interactions is assumed to describe given signals. Palus et al. E-mail address: [email protected] (V.A. Vakorin). (2001) proposed to study synchronization phenomena using the 0165-0270/$ – see front matter © 2009 Elsevier B.V. All rights reserved. doi:10.1016/j.jneumeth.2009.07.014 V.A. Vakorin et al. / Journal of Neuroscience Methods 184 (2009) 152–160 153 notion of exchange of information between experimental bivari- for this lies in the approach typical in neurophysiological studies. ate time series. A model-free causality statistic can be defined in Concerning normative research, these studies report the results terms of the conditional mutual information between the past of that are associated with the effects stably expressed across many one process and the future of another process, given the knowl- participants involved in a study. edge about the past of the latter. It can be shown that, under proper The paper is organized as follows. First, we introduce a data- conditions, transfer entropy is equivalent to the conditional mutual driven approach for estimating the prevailing directionality of information (Palus and Vejmelka, 2007). coupling between two nodes of a network. Then, we expand this Analytic tools provided by information theory seem to be a way measure to the multivariate case, which allows us to differenti- of advocating the inductive discovery of causal relations, when the ate the pure coupling between two given nodes from the coupling strength of causal coupling is induced directly from the observed mediated by the rest of the network. Finally, we study the effects of data. Under the information-theoretic approach, we do not need to indirect coupling on assessing significance of the observed causal- specify an a priori model of interactions. This attractive property ity in a group. In particular, we consider the problem of model of information-based statistics has lead to numerous applications misspecification and its effects on the robustness of the obtained in neuroscience. Palus et al. (2001) applied their techniques in a results. case study of electroencephalogram (EEG) recording of an epileptic patient. Hinrichs et al. (2006) estimated the direction of effective 2. Causality connectivity between neural activations in the brain damaged by stroke, using functional magnetic resonance imaging (fMRI). The 2.1. Transfer entropy work of Chavez et al. (2003) is an attempt to reveal active abnormal couplings in the epileptogenic networks with intracranial EEG. In this section we briefly describe a test to quantify data-driven It should be noted that typically non-linear causality is inferred i non-linear Granger causal relations between two signals. Let xt and in the case of bivariate time series. Not many attempts are made j x be realizations of two interacting processes, and , respec- to expand the application of information-theoretic measures for t i j xj xi j → i quantifying causality in a multivariate case. Gourvitch et al. (2006) tively. Intuitively, t is a non-linear cause of t , denoted as , tested the multivariate version of non-parametric Granger causality if past and present values of j contain information about future test based on using the correlation integral. The bivariate version values of i, provided that information about past and present of of this test was proposed by Baek and Brock (1992) and modified i itself is excluded. Next, we assume that the dynamics of the by Hiemstra and Jonathan (1994). It should be noted that informa- underlying multidimensional system can be reconstructed from tion is expressed in terms of individual and joint entropies, which the observed time series, using time delay embedding. The ratio- can be estimated through the corresponding correlation integrals nale for this is that many complex biophysiological phenomena (Prichard and Theiler, 1995). The test by Hiemstra and Jonathan are due to non-linear effects. Recently there has been an increas- (1994) is closely related to the causality test based on estimating ing interest in studying complex neural networks of the brain, the conditional mutual information, which was introduced by Palus applying concepts and time series analysis techniques derived from et al. (2001). However, there is no one-to-one mapping between non-linear dynamics (see Stam, 2005 for a comprehensive review). them. One approach to non-linear analysis consists of reconstructing the In the present study, to address questions on brain connectivity underlying dynamical systems underlying EEG or MEG
Details
-
File Typepdf
-
Upload Time-
-
Content LanguagesEnglish
-
Upload UserAnonymous/Not logged-in
-
File Pages9 Page
-
File Size-