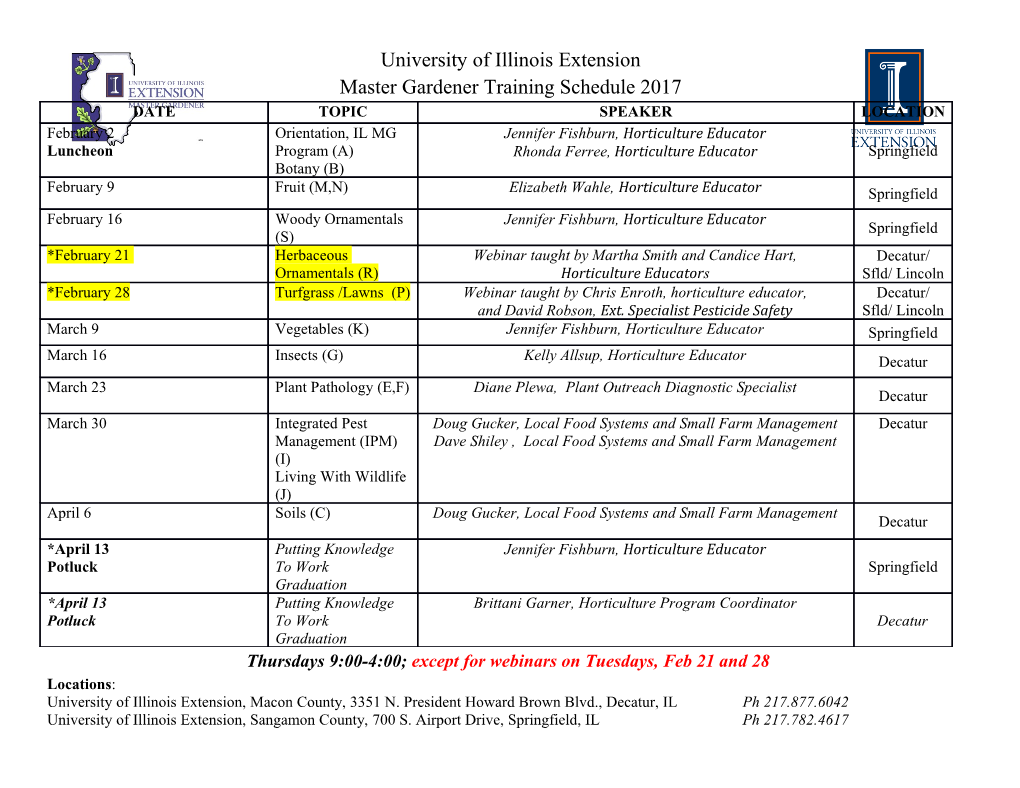
J. Japan Statist. Soc. Vol. 21 No. 1 1991 1-11 LEVERAGE POINTS IN NONLINEAR REGRESSION MODELS Yasuto Yoshizoe* We give a general definition of the leverage point in the general nonlinear regression model, including the linear regression model. In particular, the definition can be applied to binary regression models, such as the logit and the probit models. This approach makes clear when and why the ordinary definition of leverage points based on the diagonal element of the hat matrix makes sense. Key words: leverage point, influence function, empirical influence function, nonlinear regression, binary regression. 1. Introduction Consider the linear regression model y=Xβ+u, where y and u are the n-dimensional vectors of the response and error terms, X is an n×p matrix of the explanatory variables or carriers, and β is a p-dimensional vector of unknown parameters. We further assume that each component of as is independently distributed as N(0, σ2). The maximum likelihood method (which is equivalent to the least-squares method in this case) gives the estimate of β,β=(XT X)-IXTy, from which the fitted y is defined by y=Xβ=Hy, where (1.1) H=(hij)=X(XT X)-1XT is the hat matrix H (so called because it adds a hat onto y). As is well known, H is sym metric and idempotent; its diagonal element hss, which is often written as hi , satisfies O〓hi〓1; and the trace of H is given by (1.2) We first review the notion of leverage points in the linear regression model given by Hoaglin and Welsch (1978), who are the first to refer to the phrase leverage point in the literature: For the data analyst, the element hsi of H has a direct interpretation as the amount of leverage or influence exerted on yi by yj (regardless of the actual value of yj , since H depends only on X). Thus a look at the hat matrix can reveal sensitive points in the design, points at which the value of y has a large impact on the fit (Huber 1975) . In using the word “design" here, we have in mind both the standard regression or ANOVA situation, in which the values of X1, …, Xp are sampled together. The simple designs, such as two-way analysis of variance, give good control over leverage (as we shall see in Section 3); and with fixed X one can examine, and perhaps modify, Received February, 1989. Revised July, 1990. Accepted September, 1990. * Rissho University, Faculty of Economics. The author wishes to express his thanks to Kci Takeuchi, Peter Huber, John Pratt, and David Hoaglin for their valuable comments . This research was supported in part by Nihon Kcicai Kenkyuu Zaidan. 2 J. JAPAN STATIST. SOC. Vol. 21 No. 1 1991 the experimental conditions in advance. When the carriers are sampled, one can at least determine whether the observed X contains sensitive points and consider omit ting them if the corresponding y value seems discrepant. Thus we use the hat matrix to identify •ghigh-leverage points." If this notion is to be really useful, we must make it more precise. They then continue: The influence of the response value yi on the fit is most directly reflected in its lever age on the corresponding fitted value yi, and this is precisely the information con tained in his, the corresponding diagonal element of the hat matrix. The above paragraphs describe how the hat matrix is used to detect the points of leverage. In the first paragraph, the authors point out the following fact: since each component of y can be written as yi=Σjhijyj,we observe that ∂yi/∂yj=hij. That is,hij represents the effect of yf on yi. It is also interesting to observe that this effect is symmetric, in the sense that hij=hji. In the second paragraph, however, the yclaim that (1.3) most directly reflects the influence of yi on the fit. As cited by Hoaglin and Welsch, Huber (1975) first pointed out the importance of measuring the influence of a particular observation in the design matrix on the fit. Later, Huber (1981, p. 153•`155) explains the concept of the leverage point as follows: Regression poses some peculiar and difficult robustness problems. ... The difficulty is of course that a gross error does not necessarily show up through a large residual; by causing an overall increase in the size of other residuals, it can even hide behind a veritable smokescreen. In order to disentangle the issues, we must : (1) Find analytical methods for identifying so-called leverage points, that is, points in the design space where an observation, by virtue of its position, has an overriding influence on the fit and in particular on its own fitted value. ... In addition, he says (p.160), •gPoints with large hi are, by definition, leverage points." Again, we see that the influence of yi on itself is regarded as the measure of leverage. It is not immediately clear when and how this statement is valid. This is one of the ques tions we want to answer in this paper. In the following, we consider a general nonlinear regression model in order to define a measure of the influence of a particular observation on the fit. In this way, we can make clear when and why leverage points are properly defined through the hat matrix in the linear regression. 2. Leverage in Nonlinear Regressions We define our general nonlinear regression model by (2.1) E[y│x]=η(x, β) and var[y│x]=σ2ω(x)-1, where y is a scalar, x is a p-dimensional vector,η and ω are known functions, and where a p-dimensional vector β and σ2 are unknown parameters. We further assume that all y's LEVERAGE POINTS IN NONLINEAR REGRESSION MODELS 3 are independently normally distributed. Then, the maximum-1ikelihood estimate β=β[〓n] (which, again, is equivalent to the least-squaresestimate) is given by Here, is a p-dimensional vector, and 〓n=n-1Σiδ(xi, yi), where δ(x,y) is a point mass at (x,y), is the empiricaljoint measure. We also define the model distribution 〓=(η, G) as foUows: x is distributed as G (known and independent of β, that is, we regard x as an ancillary statistic), and the conditional distribution of y, given x, is normal with mean and variance as described in Eq. (2.1). Then, using the influence function, or influence curve, evaluated at the model 〓 (see Hampel (1971), Huber (1981), or Hampel el al.(1986) for the concept and derivation) is given by (2,2) where β=β[〓] is, by definition, the true value of the parameter; 〓t=(1-t)〓+tδ(x, y); and which is essentially the precision matrix of ƒÀ. In order to detect influential points in the carrier, i. e., the leverage points, we need to study the innuence of the response, rather than just that of β. A natural estimate of the response at z (using the maximum-likelihood estimate β) is (2.3) y(z)=η(z, β)=y(z)[〓n]. Its influence curve evaluated at the model 〓 is then (2.4) 1C[x, y; 〓, y(z)]=ξ(z, β)T IC[x, y;〓, β] =ξ(z, β)TS-1ξ(x, β)ω(x){y-η(x , β)}. The influence curve is a function of x and y; however, it can also be regarded as a function of z. This viewpoint is helpful, as will be seen later. Based on the IC of y(z), we can evaluate the influence of an observation at x as follows. When we have an observation at x, the effect of a small change in the corre- sponding y on y(z) can be measured by (2.5) We call this measure a cross influence. The heuristic interpretation follows. Suppose we get a new observation at (x, y) and then change the value of y there by an infinitesimal 4 J. JAPAN STATIST. SOC. Vol.21 No.1 1991 amount. Then φ(x, z)measures the ratio of the change in y(z) to the change in y at x. Obviously, this concept is analogous to hip in Eq. (1.3). In the general nonlinear regres sion, the explicit form of the cross influece is (2.6) φ(x,z)=ξ(z,β)T S-1ξ(x,β)ω(x). We can interpret the cross innuenceφ(x,z)in another way;namely、 it is equivalent to the ratio whose denominator is the residual from the fit. Although both expressions are meaningful when y is a continuous variable, the first definition naturally agress with the use of the influence function. We further observe that if mi(x)is a constant, then Eq.(2.6)is symmetric in x and z. Hence the cross influence is symmetric only when ω(x)is a constant. This property also holds for the linear regression; we have already seen that hij=hji. The condition thatω is a constant will play an important role later in our argument. A special case is the self influence defined byφ(x, x)=ξ(x,β)TS-1(x,β)ω(x), which is a measure of the influence of an observation at x and is analogous to hi in the linear regression. Uufortunately, this quantity does not always reflect the entire influence. Because of the factor ξ(x,β) in φ(x,x), instead of ξ(z,β)in Eq. (2.6), the self influence can be small even when cross influences are large for some (or most) values of z.
Details
-
File Typepdf
-
Upload Time-
-
Content LanguagesEnglish
-
Upload UserAnonymous/Not logged-in
-
File Pages11 Page
-
File Size-