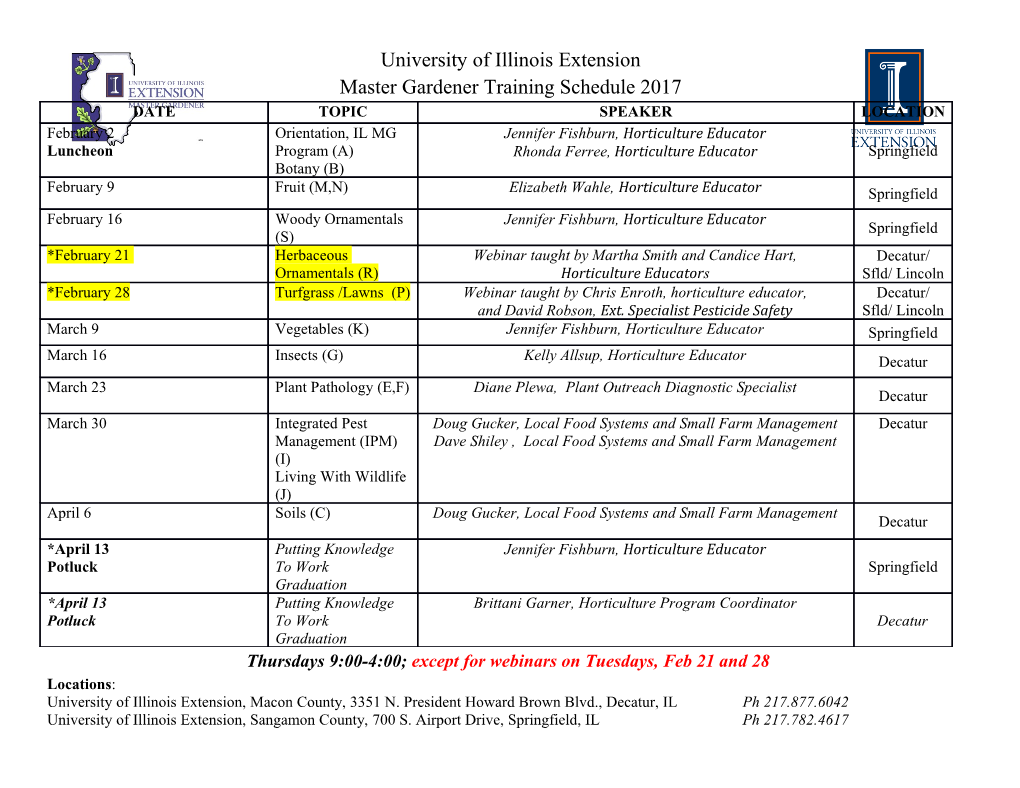
Causal Analysis of Agent Behavior for AI Safety Gregoire´ Deletang´ * 1 Jordi Grau-Moya * 1 Miljan Martic * 1 Tim Genewein 1 Tom McGrath 1 Vladimir Mikulik 1 Markus Kunesch 1 Shane Legg 1 Pedro A. Ortega 1 Abstract allow for investigating and uncovering the causal mecha- nisms that underlie an agent’s behavior. Such methodologies As machine learning systems become more pow- would enable analysts to explain, predict, and preempt fail- erful they also become increasingly unpredictable ure modes (Russell et al., 2015; Amodei et al., 2016; Leike and opaque. Yet, finding human-understandable et al., 2017). explanations of how they work is essential for their safe deployment. This technical report illus- This technical report outlines a methodology for investi- trates a methodology for investigating the causal gating agent behavior from a mechanistic point of view. mechanisms that drive the behaviour of artificial Mechanistic explanations deliver a deeper understanding of agents. Six use cases are covered, each addressing agency because they describe the cause-effect relationships a typical question an analyst might ask about an that govern behavior—they explain why an agent does what agent. In particular, we show that each question it does. Specifically, agent behavior ought to be studied cannot be addressed by pure observation alone, using the tools of causal analysis (Spirtes et al., 2000; Pearl, but instead requires conducting experiments with 2009; Dawid, 2015). In the methodology outlined here, ana- systematically chosen manipulations so as to gen- lysts conduct experiments in order to confirm the existence erate the correct causal evidence. of hypothesized behavioral structures of AI systems. In particular, the methodology encourages proposing simple Keywords: Agent analysis, black-box analysis, causal explanations that refer to high-level concepts (“the causal reasoning, AI safety. agent prefers green over red apples”) that abstract away the low-level (neural) inner workings of an agent. 1. Introduction Using a simulator, analysts can place pre-trained agents into test environments, recording their reactions to various inputs Unlike systems specifically engineered for solving a and interventions under controlled experimental conditions. narrowly-scoped task, machine learning systems such as The simulator provides additional flexibility in that it can, deep reinforcement learning agents are notoriously opaque. among other things, reset the initial state, run a sequence Even though the architecture, algorithms, and training data of interactions forward and backward in time, change the are known to the designers, the complex interplay between seed of the pseudo-random number generator, or spawn a these components gives rise to a black-box behavior that new branch of interactions. The collected data from the sim- is generally intractable to predict. This problem wors- ulator can then be analyzed using a causal reasoning engine ens as the field makes progress and AI agents become where researchers can formally express their assumptions arXiv:2103.03938v1 [cs.AI] 5 Mar 2021 more powerful and general. As illustrated by learning-to- by encoding them as causal probabilistic models and then learn approaches, learning systems can use their experience validate their hypotheses. Although labor-intensive, this to induce algorithms that shape their entire information- human-in-the-loop approach to agent analysis has the ad- processing pipeline, from perception to memorization to vantage of producing human-understandable explanations action (Wang et al., 2016; Andrychowicz et al., 2016). that are mechanistic in nature. Such poorly-understood systems do not come with the nec- essary safety guarantees for deployment. From a safety 2. Methodology perspective, it is therefore paramount to develop black-box methodologies (e.g. suitable for any agent architecture) that We illustrate this methodology through six use cases, se- lected so as to cover a spectrum of prototypical questions *Equal contribution 1AGI Safety Analysis, DeepMind, an agent analyst might ask about the mechanistic drivers London, UK. Correspondence to: Pedro A. Ortega <pe- of behavior. For each use case, we present a minimalis- [email protected]>. tic grid-world example and describe how we performed ©2020 by the authors. our investigation. We limit ourselves to environmental and Causal Analysis of Agent Behavior for AI Safety behavioral manipulations, but direct interventions on the internal state of agents are also possible. The simplicity in a) t t+1 our examples is for the sake of clarity only; conceptually, all solution methods carry over to more complex scenarios under appropriate experimental controls. Our approach uses several components: an agent and an environment, a simulator of interaction trajectories, and a causal reasoning engine. These are described in turn. b) W W 2.1. Agents and environments t t+1 For simplicity, we consider stateful agents and environments that exchange interaction symbols (i.e. actions and obser- At-1 Ot At Ot+1 vations) drawn from finite sets in chronological order at discrete time steps t = 1; 2; 3;::: Typically, the agent is a system that was pre-trained using reinforcement learning Mt-1 Mt Mt+1 and the environment is a partially-observable Markov de- time t cision process, such as in Figure1a. Let mt; wt (agent’s memory state, world state) and a ; o (action, observation) t t Figure 1. Agents and environments. a) The goal of the agent is to denote the internal states and interaction symbols at time t pick up a reward pill without stepping into a lava tile. b) Causal of the agent and the environment respectively. These inter- Bayesian network describing the generative process of agent- actions influence the stochastic evolution of their internal environment interactions. The environmental state Wt and the states according to the following (causal) conditional proba- agent’s memory state Mt evolve through the exchange of action bilities: and observation symbols At and Ot respectively. wt ∼ P (wt j wt−1; at−1) ot ∼ P (ot j wt) (1) system made from coupling an agent and an environment, a m ∼ P (m j m ; o ) a ∼ P (a j m ): (2) t t t−1 t t t t random seed ! ∼ P (!), and a desired length T , it generates These dependencies are illustrated in the causal Bayesian a trace network of Figure1b describing the perception-action loop τ = (!; s1; x1); (!; s2; x2); (!; s3; x3);:::; (!; sT ; xT ) (Tishby & Polani, 2011). of a desired length T , where the s := (! ; m ) and Since we wish to have complete control over the stochastic t t t x := (o ; a ) are the combined state and interaction sym- components of the interaction process (by controlling its t t t bols respectively, and where ! is the random element which random elements), we turn the above into a deterministic has been made explicit. The simulator can also contract system through a re-parameterization1. Namely, we repre- (rewind) or expand the trace to an arbitrary time point sent the above distributions using functions W; M; O; A as T 0 ≥ 1. Note that this works seamlessly as the genera- follows: tive process of the trace is deterministic. wt = W (wt−1; at−1;!) ot = O(wt;!) (3) In addition, the simulator allows for manipulations of the mt = M(mt−1; ot;!) at = A(mt;!) (4) trace. Such an intervention at time t can alter any of the three components of the triple (!; st; xt). For instance, where ! ∼ P (!) is the random seed. This re- changing the random seed in the first time step corresponds parameterization is natural in the case of agents and en- to sampling a new trajectory: vironments implemented as programs. τ = (!; s1; x1); (!; s2; x2);:::; (!; sT ; xT ) 2.2. Simulator # (5) τ 0 = (!0; s0 ; x0 ); (!0; s0 ; x0 );:::; (!0; s0 ; x0 ); The purpose of the simulator is to provide platform for exper- 1 1 2 2 T T imentation. Its primary function is to generate traces (roll- whereas changing the state at time step t = 2 produces a outs) of agent-environment interactions (Figure2). Given a new branch of the process sharing the same root: 1 That is, we describe the system as a structural causal model τ = (!; s1; x1); (!; s2; x2);:::; (!; sT ; xT ) as described in Pearl(2009, chapter 7). Although this parame- terization is chosen for the sake of concreteness, others are also # (6) 0 0 0 0 0 possible. τ = (!; s1; x1); (!; s2; x2);:::; (!; sT ; xT ): Page 2 Causal Analysis of Agent Behavior for AI Safety Rollout 1 change seed Rollout 2 change tiles Rollout 3 Figure 2. Simulating a trace (rollout) and performing interventions, creating new branches. Using these primitives one can generate a wealth of data model for this situation would be the system of equations about the behavior of the system. This is illustrated in Figure2. X = fX (UX ) UX ∼ P (UX ) Y = fY (X; UY ) UY ∼ P (UY ) (7) 2.3. Causal reasoning engine Z = fZ (X; Y; UZ ) UZ ∼ P (UZ ) Finally, in order to gain a mechanistic understanding of the agent’s behavior from the data generated by the simulator, where fX ; fY , and fZ are (deterministic) functions and it is necessary to use a formal system for reasoning about where the (exogenous) variables UX ;UY , UZ encapsulate statistical causality. The purpose of the causal reasoning the stochastic components of the model. Together, they engine is to allow analysts to precisely state and validate induce the conditional probabilities their causal hypotheses using fully automated deductive reasoning algorithms. P (X);P (Y j X); and P (Z j X; Y ): (8) As an illustration of the modeling process, consider an an- These probabilities can be directly supplied by the analyst alyst wanting to understand whether an agent avoids lava (e.g. if they denote prior probabilities over hypotheses) or when trying to reach a goal state. First, the analyst selects estimated from Monte-Carlo samples obtained from the the set of random variables X they want to use to model the simulator (see next subsection).
Details
-
File Typepdf
-
Upload Time-
-
Content LanguagesEnglish
-
Upload UserAnonymous/Not logged-in
-
File Pages16 Page
-
File Size-