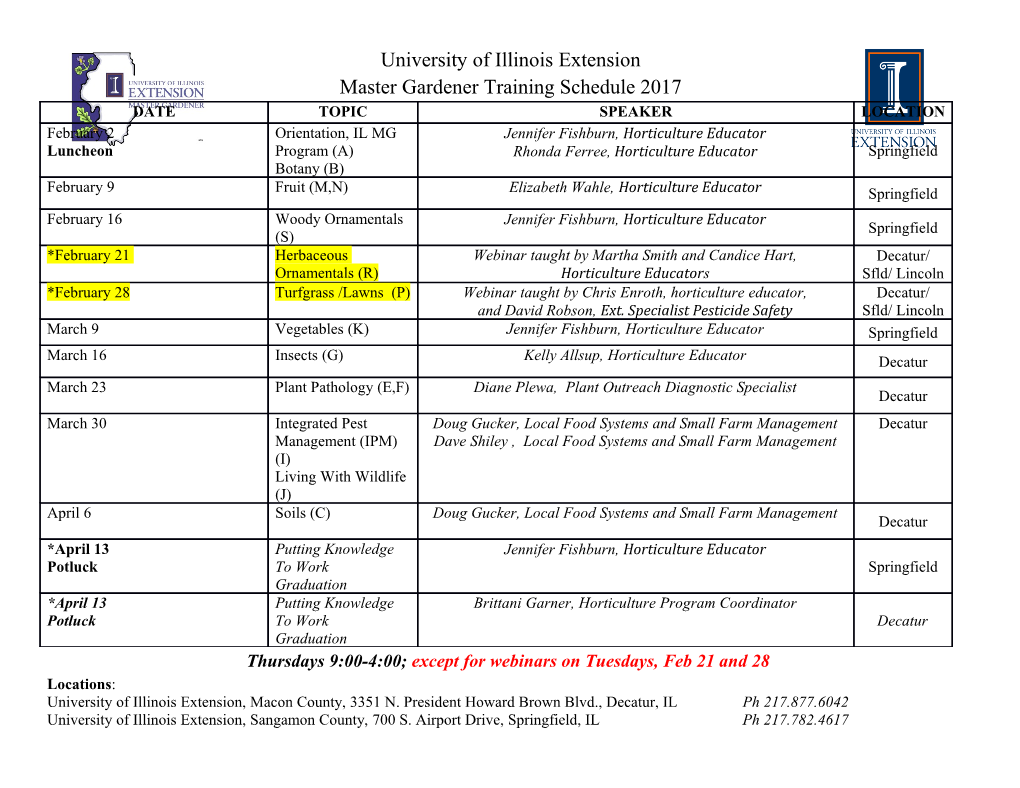
Intro to SDEs with with Examples Introduction to the Numerical Simulation of Stochastic Differential Equations with Examples Prof. Michael Mascagni Department of Computer Science Department of Mathematics Department of Scientific Computing Florida State University, Tallahassee, FL 32306 USA E-mail: [email protected] or [email protected] URL: http://www.cs.fsu.edu/∼mascagni Intro to SDEs with with Examples Introduction Stochastic Differential Equations Brownian Motion Itô Calculus Numerical Solution of SDEs Types of Solutions to SDEs Examples Higher-Order Methods Some Applications Stability Weak Solutions Higher-Order Schemes Examples Numerical Examples Bibliography Intro to SDEs with with Examples Introduction Stochastic Differential Equations Brownian Motion Itô Calculus Numerical Solution of SDEs Types of Solutions to SDEs Examples Higher-Order Methods Some Applications Stability Weak Solutions Higher-Order Schemes Examples Numerical Examples Bibliography Intro to SDEs with with Examples Introduction Stochastic Differential Equations Brownian Motion Itô Calculus Numerical Solution of SDEs Types of Solutions to SDEs Examples Higher-Order Methods Some Applications Stability Weak Solutions Higher-Order Schemes Examples Numerical Examples Bibliography Intro to SDEs with with Examples Stochastic Differential Equations Stochastic Differential Equations Stoke’s law for a particle in fluid dv(t)=−γ v(t) dt where π γ = 6 r η, m η = viscosity coefficient. Langevin’s eq. For very small particles bounced around by molecular movement, dv(t)=−γ v(t) dt + σ dw(t), w(t) is a Brownian motion, γ = Stoke’s coefficient. σ =Diffusion coefficient. Intro to SDEs with with Examples Stochastic Differential Equations Brownian Motion 1-D Brownian Motion Figure: 1-D Brownian motion Intro to SDEs with with Examples Stochastic Differential Equations Brownian Motion 2-D, or Complex Brownian Motion Figure: 2-D Brownian motion Intro to SDEs with with Examples Stochastic Differential Equations Brownian Motion Brownian Motion w(t)=Brownian motion. Einstein’s relation gives diffusion coefficient γ σ = 2kT . m and probability density function for Brownian motion satisfies heat equation: ∂ ( , ) ∂2 ( , ) p w t = 1 p w t ∂t 2 ∂w 2 Formal solution to LE is called an Ornstein-Uhlenbeck process t −γt −γt γs v(t)=v0e + σe e dw(s) 0 Intro to SDEs with with Examples Stochastic Differential Equations Brownian Motion A Simple Stochastic Differential Equation What does dw(t) mean? w(t)=Δw1 +Δw2 + ···+Δwn each increment is independent, and E{Δwi Δwj } = δij Δt or infinitesimal version Edw(t)=0 E{dw(t) dw(s)} = δ(t − s) dt ds Intro to SDEs with with Examples Stochastic Differential Equations Brownian Motion The Langevin Equation Solution to LE has properties t −γt −γt γs Ev(t)=v0e + σe e Edw(s) 0 −γt = v0e and e2γt − 1 E(v(t))2 =(v )2e−2γt + σ2e−2γt 0 2γ σ2 → as t →∞ 2γ Something familiar about this? m m σ2 1 E(v)2 = = kT 2 2 2γ 2 Intro to SDEs with with Examples Stochastic Differential Equations Itô Calculus Itô Calculus Itô calculus for multi-dimensional version 2 dw(t) ≡ dt or dwi (t)dwj (t) ≡ δij dt In non-isotropic case, system dz = b(z) dt + σ(z) dw(t) (SDE) is shorthand for t t z(t)=z0 + b(zs) ds + σ(zs) dws. 0 0 Itô rule for Stochastic integral: t E{ σ(zs)dws} = 0, 0 and t t 2 T E{ σ(zs)dws} = σσ (zs)ds. 0 0 These integrals are martingales. Intro to SDEs with with Examples Stochastic Differential Equations Itô Calculus A Standing Martingale Figure: A standing martingale Intro to SDEs with with Examples Stochastic Differential Equations Numerical Solution of SDEs Numerical Solution of SDEs Simulation? First, 1 N Ef (z(t)) ≈ f (z[i](t)) N i=1 for sample of N paths z(t). Paths {z[1], z[2], ..., z[N]} integrated by some rule, e.g. Euler Two criteria two versions of solution z˜(t), z(t) are equivalent (z˜(t) ≡ z(t)) for 0 ≤ t ≤ T , strong criteria: P( sup |z˜(t) − z(t)| > 0)=0 0≤t≤T weak:forany sufficiently smooth f (x), |Ef (z˜(T )) − Ef (z(T ))| = 0 Intro to SDEs with with Examples Stochastic Differential Equations Types of Solutions to SDEs Weak Solutions Example: weak simulation (m ≥ 0): dx = −x|x|m−1dt + dw(t) has solution whose distribution law satisfies Kolmogorov equation ∂p(x, t) ∂ 1 ∂ = + x|x|m−1 p(x, t) → 0 ∂t ∂x 2 ∂x when t →∞. That is, x(t) becomes stationary. p(x, t →∞), properly normalized, is 2 m+1 − + |x| p(x, ∞)=Nme m 1 . Two examples p(x, ∞)=e−2|x| for m = 0 ( , ∞)=√1 −|x|2 = p x π e for m 1 Intro to SDEs with with Examples Stochastic Differential Equations Types of Solutions to SDEs Strong Solutions Example: a strong test, dx = −λxdt + μxdw(t) having formal solution μ2 x(t)=x exp (−(λ + )t + μw(t)). (1) 0 2 Notice x(t) → 0ast →∞. Many authors (Mitsui et al, Higham, ...) have studied stability regions, λ, μ, for asymptotic stability x(tn) → 0, when tn = h1 + h2 + ...+ hn →∞ may have varying stepsizes. Cases t = T1 = n · h, and h → h/2m = h, m t = Tm = n2 · h Intro to SDEs with with Examples Stochastic Differential Equations Types of Solutions to SDEs Strong Solutions allow pathwise comparisons when = = = · tn T1 Tm √n h Δ ( + )= ξ w Tm h √h 1 √ Δw(Tm + h + h )=h ξ1 + h ξ2 ... √ Δ ( + )=m ξ w Tm h k=1 h k Δw(T1 + h)=Δw(Tm + h) Here, one follows the pathwise convergence as m is changed. See Kloeden and Platen, chapt. 9, p. 309. One compares "exact" solution, equation (1), with simulation values at points T1 = Tm. Intro to SDEs with with Examples Stochastic Differential Equations Types of Solutions to SDEs Strong Solutions Numerical criteria similar: discrete times tk = kh, h = step size, T = Mh, and zk = numerical approx., strong order β: 2 1/2 β (E max |zk − z(tk )| ) ≤ K1h 0≤k≤M weak order β:forf (z) ∈ C2β, β |Ef (zM ) − Ef (z(T ))|≤K2h Intro to SDEs with with Examples Stochastic Differential Equations Examples Examples Example methods: Euler-Maruyama zk+1 = zk + b(zk )h + σ(zk )ΔW is strong order β = 1/2, weak order 1. Milstein zk+1 =zk + b(zk )h + σ(zk )ΔW 1 + σ(z )σ(z )(ΔW 2 − h) 2 k k is strong order β = 1, weak order 1 Intro to SDEs with with Examples Stochastic Differential Equations Higher-Order Methods Higher-Order Methods Higher order weak methods require modeling h h Iij = wi dwj Ii0 = wi (s)ds 0 0 h h = = 2 Iijk wi wj dwk Iii0 wi ds 0 0 For example, for Runge-Kutta type methods 1 h I ≈ ξ ξ + Ξ , ij 2 i j 2 ij h I ≈ ξ , i0 2 i h I ≈ δ ξ ijk 2 ij k h I ≈ ξ2 ii0 2 i Ξij is a model for wi dwj − wj dwi . Intro to SDEs with with Examples Stochastic Differential Equations Higher-Order Methods Examples Δw = ξ is approximately gaussian Eξ = 0, Eξ2 = h, Eξ3 = 0, Eξ4 = 3h2. Do N sample paths per time-step - one for each z[i]. A simple Δw is √ ξ = 1 √3h with probability 6 , = − 1 3h with probability 6 , = 2 . 0 with probability 3 Important facts about these bounded increments: √ they introduce Fourier spectra with wave vectors = k 3h, where k ∈ Zd . in d > 1 dimensions, Δw is not isotropic. Intro to SDEs with with Examples Stochastic Differential Equations Higher-Order Methods Examples Δw = ξ is approximately gaussian Eξ = 0, Eξ2 = h, Eξ3 = 0, Eξ4 = 3h2. Do N sample paths per time-step - one for each z[i]. A simple Δw is √ ξ = 1 √3h with probability 6 , = − 1 3h with probability 6 , = 2 . 0 with probability 3 Important facts about these bounded increments: √ they introduce Fourier spectra with wave vectors = k 3h, where k ∈ Zd . in d > 1 dimensions, Δw is not isotropic. Intro to SDEs with with Examples Stochastic Differential Equations Higher-Order Methods Examples of Bounded Increments Figure: 3-D distribution of bounded increments Intro to SDEs with with Examples Stochastic Differential Equations Some Applications Some Applications Some applications: Black-Scholes model for asset volatility Langevin dynamics shearing of light in inhomogeneous universes Intro to SDEs with with Examples Stochastic Differential Equations Some Applications Some Applications Some applications: Black-Scholes model for asset volatility Langevin dynamics shearing of light in inhomogeneous universes Intro to SDEs with with Examples Stochastic Differential Equations Some Applications Some Applications Some applications: Black-Scholes model for asset volatility Langevin dynamics shearing of light in inhomogeneous universes Intro to SDEs with with Examples Stochastic Differential Equations Some Applications Black-Scholes Black-Scholes model. Let S = asset price, r = interest rate. Without volatility, dS = rSdt. With efficient market hypothesis, fluctuations(S) ∝ S: dS = rS dt + σSdw. σ is called the volatility. Solution to SDE (r− 1 σ2)t+σw(t) S(t)=S0 e 2 . Intro to SDEs with with Examples Stochastic Differential Equations Some Applications Langevin Dynamics Langevin dynamics: we want some physical quantity e−S(x)f (x)d nx Ef = p(x)f (x)dn x = . e−S(x)d nx To find a covering distribution q(x),αq(x) ≥ p(x),butα ≥ 1 is not large - difficult if n large. Alternative is Langevin dynamics: 1 ∂S dx(t)=− dt + dw(t), 2 ∂x and use 1 T Ef = lim f (x(t))dt. →∞ T T 0 The following is sufficient for convergence: if |x| big, ∂S x · > 1 ∂x Intro to SDEs with with Examples Stochastic Differential Equations Some Applications A Simple Example A simple example: dx = −sign(x)dt + dw, whose p.d.f as t →∞is p(x, ∞)=e−2|x|.
Details
-
File Typepdf
-
Upload Time-
-
Content LanguagesEnglish
-
Upload UserAnonymous/Not logged-in
-
File Pages51 Page
-
File Size-