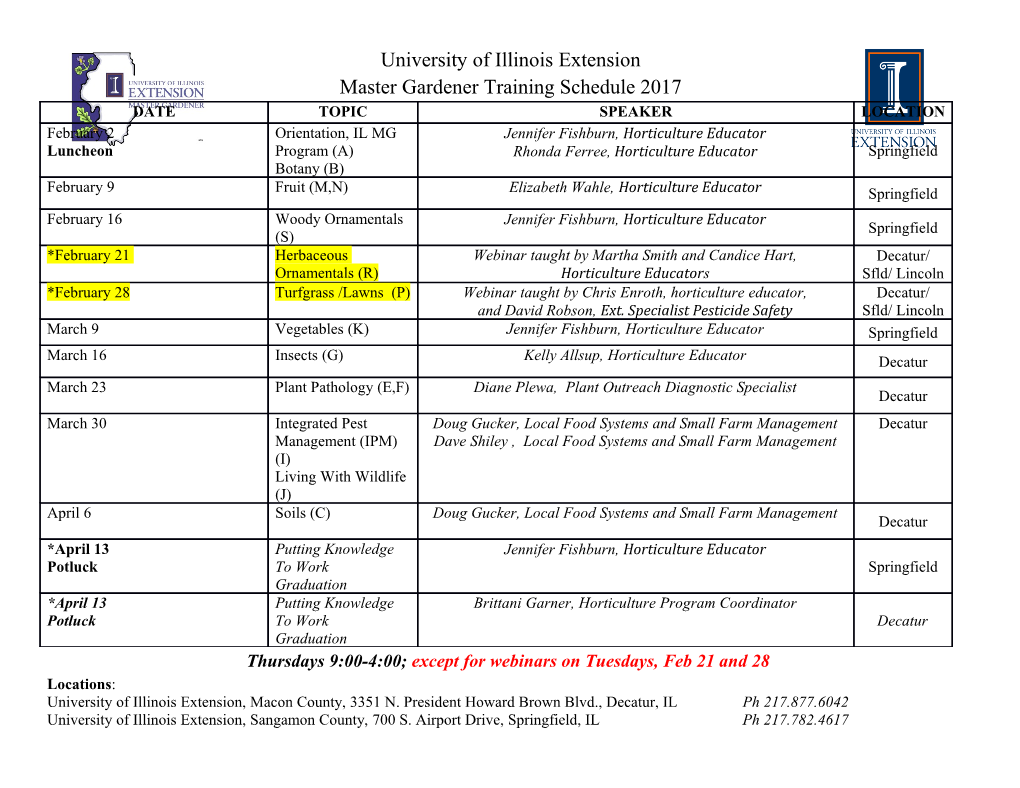
Lecture 3: Minimaxity and admissibility Consider estimators of a real-valued J = g(q) based on a sample X from Pq , q 2 Θ, under loss L and risk RT (q) = E[L(T (X);q)]. Minimax estimator An estimator d is minimax if supq Rd (q) = infall T supq RT (q) Discussion A minimax estimator can be very conservative and unsatisfactory. It tries to do as well as possible in the worst case. A unique minimax estimator is admissible, since any estimator better than a minimax estimator is also minimax. We should find an admissible minimax estimator. Different for UMVUE: if a UMVUE is inadmissible, it is dominated by a biased estimator) If a minimax estimator has some other good properties (e.g., it is a Bayes estimator), then it is often a reasonable estimator. beamer-tu-logo UW-Madison (Statistics) Stat 710 Lecture 3 Jan 2019 1 / 15 Minimax estimator The following result shows when a Bayes estimator is minimax. Theorem 4.11 (minimaxity of a Bayes estimator) Let Π be a proper prior on Θ and d be a Bayes estimator of J w.r.t. Π. Suppose d has constant risk on ΘΠ. If Π(ΘΠ) = 1, then d is minimax. If, in addition, d is the unique Bayes estimator w.r.t. Π, then it is the unique minimax estimator. Proof Let T be any other estimator of J. Then Z Z sup RT (q) ≥ RT (q)dΠ ≥ Rd (q)dΠ = sup Rd (q): q2Θ ΘΠ ΘΠ q2Θ If d is the unique Bayes estimator, then the second inequality in the previous expression should be replaced by > and, therefore, d is the unique minimax estimator. beamer-tu-logo UW-Madison (Statistics) Stat 710 Lecture 3 Jan 2019 2 / 15 Example 4.18 Let X1;:::;Xn be i.i.d. binary random variables with P(X1 = 1) = p. Consider the estimation of p under the squared error loss. The UMVUE X¯ has risk p(1 − p)=n which is not constant. In fact, X¯ is not minimax (Exercise 67). To find a minimax estimator by applying Theorem 4.11, we consider the Bayes estimator w.r.t. the beta distribution B(a;b) with known a and b (Exercise 1): d(X) = (a + nX¯ )(a + b + n): 2 2 Rd (p) = [np(1 − p) + (a − ap − bp) ] (a + b + n) : To apply Theorem 4.11, we need to find values of a > 0 and b > 0 such that Rd (p) is constant. p It can be shown that Rd (p) is constant if and only if a = b = n=2, which leads to the unique minimax estimator p p T (X) = (nX¯ + n=2)(n + n): p beamer-tu-logo 2 The risk of T is RT = 1=[4(1 + n) ]. UW-Madison (Statistics) Stat 710 Lecture 3 Jan 2019 3 / 15 Example 4.18 (continued) Note that T is a Bayes estimator and has some good properties. Comparing the risk of T with that of X¯ , we find that T has smaller risk if and only if q q p 2 1 − 1 1 − np ; 1 + 1 1 − np : 2 2 (1+ n)2 2 2 (1+ n)2 Thus, for a small n, T is better (and can be much better) than X¯ for most of the range of p (Figure 4.1). 1 When n ! ¥, the above interval shrinks toward 2 . Hence, for a large (and even moderate) n, X¯ is better than T for most of the range of p (Figure 4.1). The limit of the asymptotic relative efficiency of T w.r.t. X¯ is 4p(1 − p), 1 1 which is always smaller than 1 when p 6= 2 and equals 1 when p = 2 . Minimaxity depends strongly on the loss function. Under the loss function L(p;a) = (a − p)2=[p(1 − p)], X¯ has constant risk and is the unique Bayes estimator w.r.t. the uniform prior on (0;1). By Theorem 4.11, X¯ is the unique minimax estimator. p beamer-tu-logo The risk, however, of T is 1=[4(1 + n)2p(1 − p)], which is unbounded. UW-Madison (Statistics) Stat 710 Lecture 3 Jan 2019 4 / 15 Figure 4.1. mse’s of X¯ (curve) and T (X) (straight line) in Example 4.18 n=1 n=4 mse 0.0 0.10 0.20 0.30 n=9 n=16 mse beamer-tu-logo 0.0 0.10 0.20 0.30 0.0 0.25 0.5 0.75 1.0 0.0 0.25 0.5 0.75 1.0 UW-Madison (Statistics) p Stat 710 Lecture 3 p Jan 2019 5 / 15 How to find a minimax estimator? Candidates for minimax: estimators having constant risks. Theorem 4.11 (minimaxity of a Bayes estimator) A limit of Bayes estimators In many cases a constant risk estimator is not a Bayes estimator (e.g., an unbiased estimator under the squared error loss), but a limit of Bayes estimators w.r.t. a sequence of priors. The next result may be used to find a minimax estimator. Theorem 4.12 Let Πj , j = 1;2;:::, be a sequence of priors and rj be the Bayes risk of a Bayes estimator of J w.r.t. Πj . Let T be a constant risk estimator of J. If liminfj rj ≥ RT , then T is minimax. Although Theorem 4.12 is more general than Theorem 4.11 in finding minimax estimators, it does not provide uniqueness of the minimaxbeamer-tu-logo estimator even when there is a unique Bayes estimator w.r.t. each Πj . UW-Madison (Statistics) Stat 710 Lecture 3 Jan 2019 6 / 15 Example 2.25 2 Let X1;:::;Xn be i.i.d. components having the N(m;s ) distribution with an unknown m = q 2 R and a known s 2. 2 If the prior is N(m0;s0 ), then the posterior of q given X = x is 2 N(m∗(x);c ) with s 2 ns 2 s 2s 2 m (x) = m + 0 x¯ and c2 = 0 ∗ 2 2 0 2 2 2 2 ns0 + s ns0 + s ns0 + s We now show that X¯ is minimax under the squared error loss. For any decision rule T , Z Z sup RT (q) ≥ RT (q)dΠ(q) ≥ Rm∗ (q)dΠ(q) q2R R R n 2o n 2 o 2 2 = E [~q − m∗(X)] = E Ef[~q − m∗(X)] jXg = E(c ) = c : 2 2 2 2 Since this result is true for any s0 > 0 and c ! s =n as s0 ! ¥, s 2 sup RT (q) ≥ = sup RX¯ (q); beamer-tu-logo q2R n q2R UW-Madison (Statistics) Stat 710 Lecture 3 Jan 2019 7 / 15 Example 2.25 (continued) where the equality holds because the risk of X¯ under the squared error loss is s 2=n and independent of q = m. Thus, X¯ is minimax. To discuss the minimaxity of X¯ in the case where s 2 is unknown, we need the following lemma. Lemma 4.3 Let Θ0 be a subset of Θ and T be a minimax estimator of J when Θ0 is the parameter space. Then T is a minimax estimator if sup RT (q) = sup RT (q): q2Θ q2Θ0 Proof If there is an estimator T0 with supq2Θ RT0 (q) < supq2Θ RT (q), then sup RT0 (q) ≤ sup RT0 (q) < sup RT (q) = sup RT (q); q2Θ0 q2Θ q2Θ q2Θ0 which contradicts the minimaxity of T when Θ0 is the parameter space.beamer-tu-logo Hence, T is minimax when Θ is the parameter space. UW-Madison (Statistics) Stat 710 Lecture 3 Jan 2019 8 / 15 Example 4.19 2 2 Let X1;:::;Xn be i.i.d. from N(m;s ) with unknown q = (m;s ). Consider the estimation of m under the squared error loss. Suppose first that Θ = R × (0;c] with a constant c > 0. Let Θ0 = R × fcg. From Example 2.25, X¯ is a minimax estimator of m when the parameter space is Θ0. By Lemma 4.3, X¯ is also minimax when the parameter space is Θ. Although s 2 is assumed to be bounded by c, the minimax estimator X¯ does not depend on c. Consider next the case where Θ = R × (0;¥), i.e., s 2 is unbounded. Let T be any estimator of m. For any fixed s 2, s 2 ≤ sup RT (q); n m2R since s 2=n is the risk of X¯ that is minimax when s 2 is known. 2 Letting s ! ¥, we obtain that supq RT (q) = ¥ for any estimator T . Thus, minimaxity is meaningless (any estimator is minimax). beamer-tu-logo UW-Madison (Statistics) Stat 710 Lecture 3 Jan 2019 9 / 15 Admissibility The following is another result to show admissibility. Theorem 4.14 (Admissibility in one-parameter exponential families) Suppose that X has the p.d.f. c(q)eqT (x) w.r.t. a s-finite measure n, where T (x) is real-valued and q 2 (q−;q+) ⊂ R. Consider the estimation of J = E[T (X)] under the squared error loss. Let l ≥ 0 and g be known constants and let Tl;g (X) = (T + gl)=(1 + l): Then a sufficient condition for the admissibility of Tl;g is that − − Z q+ e glq Z q0 e glq dq = dq = ¥; l l q0 [c(q)] q− [c(q)] beamer-tu-logo where q0 2 (q−;q+). UW-Madison (Statistics) Stat 710 Lecture 3 Jan 2019 10 / 15 Remarks Theorem 4.14 provides a class of admissible estimators.
Details
-
File Typepdf
-
Upload Time-
-
Content LanguagesEnglish
-
Upload UserAnonymous/Not logged-in
-
File Pages15 Page
-
File Size-