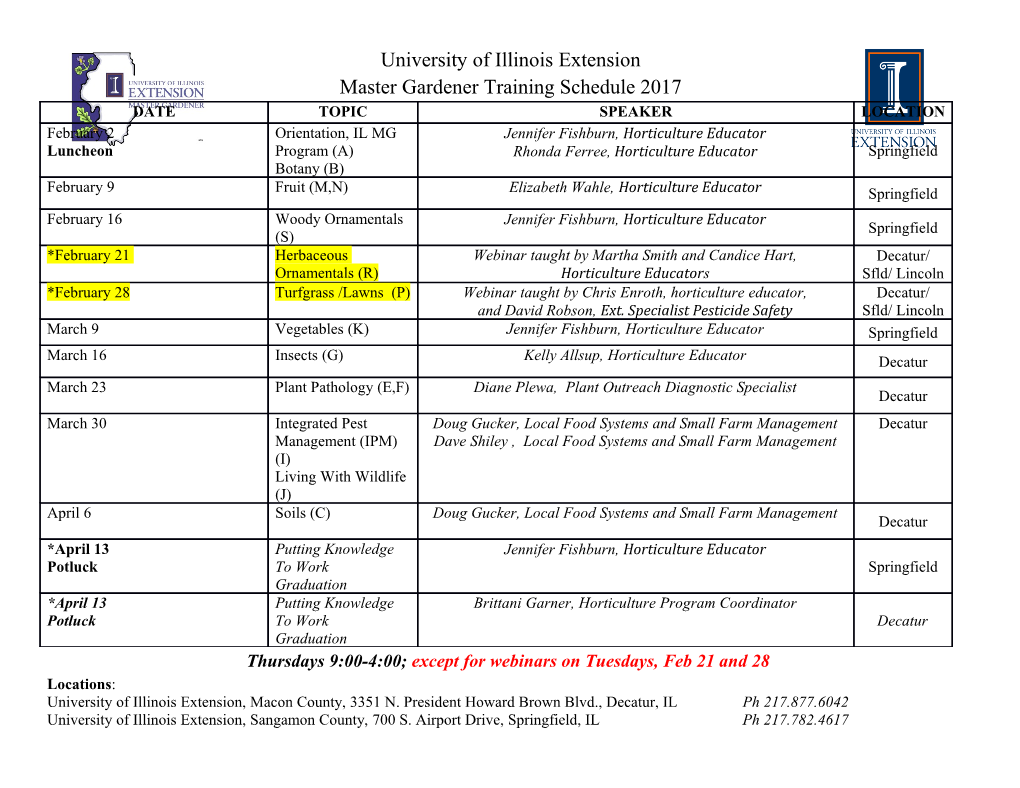
Adam Berenzweig,* BethLogan, † Daniel ALarge-Scale Evaluation P.W. Ellis,* and BrianWhitman †‡ *LabROSA of Acoustic and Columbia University NewYork, NewYork 10027 USA [email protected] Subjective Music- [email protected] †HP Labs Similarity Measures OneCambridge Center Cambridge,Massachusetts 02142– 1612 USA [email protected] ‡Music,Mind &MachineGroup MIT MediaLab Cambridge,Massachusetts 02139– 4307 USA [email protected] Avaluablegoal in the eld of MusicInformation gained.In our previous work (Ellis etal.2002), we Retrieval(MIR) is to devisean automatic measure haveexamined several sources of humanopinion of thesimilarity betweentwo musicalrecordings aboutmusic similarity, with theimpetus thathu- basedonly on ananalysisof theiraudio content. manopinion must bethe nalarbiter of music Sucha tool—aquantitative measure of similarity— similarity, becauseit is asubjectiveconcept. How- canbe usedto build classication, retrieval, brows- ever,as expected,there are as many opinions about ing,and recommendation systems. To develop musicsimilarity asthereare people to beasked, sucha measure,however, presupposes some andso thesecond question is how to unify the groundtruth, a singleunderlying similarity that various sourcesof opinion into asingleground constitutesthe desired output of themeasure. Mu- truth.As we shall see,it turnsout thatperhaps sicsimilarity is anelusiveconcept— wholly subjec- this is thewrong way to look atthings, and so we tive,multifaceted, and a moving target—but one develop theconcept of a‘‘consensustruth’ ’ rather thatmust bepursued in support of applications to thana singleground truth. provide automaticorganization of largemusic col- Finally, armedwith theseevaluation techniques, lections. weprovide anexampleof across-siteevaluation of In this article,we explore music-similarity mea- severalacoustic- and subjective-based similarity suresin severalways, motivated by different types measures.We addressseveral main researchques- of questions.We are rstmotivated by thedesire tions. Regardingthe acoustic measures, which fea- to improve automatic,acoustic-based similarity turespaces and which modeling andcomparison measures.Researchers from severalgroups have re- methods arebest? Regarding the subjective mea- centlytried manyvariations of afewbasic ideas, sures,which provides thebest single ground truth, but it remainsunclear which are best-suited for a thatis, which agrees best on averagewith theother givenapplication. Few authors perform compari- sources? sonsacross multiple techniques,and it is impossi- In theprocess of answeringthese questions, we ble to compareresults from different authors, addresssome of thelogistical difculties peculiar becausethey do not sharethe required common to our eld,such as the legal obstacles to sharing ground:a common databaseand a common evalua- musicbetween research sites. We believethis is tion method. oneof the rstand largest cross-site evaluations in Of course,to improve anymeasure, we needan MIR. Ourwork wasconducted in threeindepen- evaluationmethodology, ascientic wayof deter- dentlabs (LabROSA at Columbia, MIT, andHP mining whetherone variant is betterthan another. Labsin Cambridge),yet by carefullyspecifying our Otherwise,we are left to intuition, andnothing is evaluationmetrics, and by sharingdata in theform ComputerMusic Journal, 28:2, pp. 63 –76,Summer 2004 of derived features(which presents little threatto q 2004Massachusetts Institute ofTechnology. copyright holders),we were able to make nedis- Berenzweig,Logan, Ellis, and Whitman 63 tinctions betweenalgorithms runningat each site. out of necessityto build usefulapplications using Weseethis asapowerful paradigmthat we would currenttechniques. Before proceeding, however, it like toencourageother researchers to use. is worthwhile to examinein more detail someof Finally, anoteabout the terminology usedin this theproblems thatbeset the idea of acoherent article.To date,we have worked primarily with quantitativemeasure of musicsimilarity. popular music,and our vocabularyis thusslanted. Unlessnoted otherwise, when we refer to ‘‘artists’’ or‘‘musicians’’ wearereferring to theperformer, Individual Variation not thecomposer (which frequently arethe same anyway).Also, whenwe referto a‘‘song,’’ wemean Thatpeople have individual tastesand preferences asinglerecording of aperformanceof apieceof is centralto thevery ideaof musicand humanity. music,not anabstractcomposition, andalso not By thesame token, subjective judgments of the necessarilyvocal music. similarity betweenspeci c pairsof artistsare not This articleis organizedas follows. First weex- consistentbetween listeners and may vary with an aminethe concept of musicsimilarity andreview individual’s mood or evolveover time.In particu- prior work.We thendescribe the various algo- lar,music that holds no interestfor agivensubject rithms anddata sources used in this article.Next, veryfrequently ‘‘soundsthe same.’ ’ wedescribeour evaluationmethodologies in detail anddiscuss issues with performing amulti-site evaluation.Then we discussour experimentsand Multiple Dimensions results.Finally, wepresent conclusions and sugges- tions for futuredirections. Thequestion of thesimilarity betweentwo artists canbe answered from multiple perspectives.Music may besimilar or distinct in termsof genre,mel- Music Similarity ody, rhythm, tempo,geographical origin, instru- mentation,lyric content,historical timeframe— Theconcept of similarity hasbeen studied many virtually anyproperty thatcan be used to describe times in eldsincluding psychology,information music.Although thesedimensions arenot indepen- retrieval,and epistemology. Perhaps the most fa- dent,it is clearthat different emphaseswill result mous similarity researcheris Amos Tversky,a cog- in different artists.The fact that both PaulAnka nitive psychologist who formalized andstudied andAlanis Morissetteare from Canadamight beof similarity, perception,and categorization. Tversky paramountsigni cance to aCanadiancultural na- wasquick to notethat human judgments of simi- tionalist, althoughanother person might not nd larity do not satisfy thede nition of aEuclidean theirmusic at all similar. metric,as discussed below (Tversky1977). Healso studied thecontext-dependent nature of similarity andnoted the interplay betweensimilarity andcat- Not a Metric egorization.Other notable work includesGold- stone,Medin, and Gentner (1991) andthe music Asdiscussed in Tversky (1977) andelsewhere, sub- psychology literature—e.g., Deutsch (1999) andthe jectivesimilarity often violates thede nition of a study of melodic similarity in Cambouropoulos metric,in particularthe properties of symmetry (2001). andthe triangle inequality. For example,we might In this article,we are essentially trying to pin a saythat the 1990s LosAngeles pop musicianJason single,quantitative measure to aconceptthat fun- Falkneris similar to theBeatles, but wewould be damentallyresists such de nition. Later,we partly lesslikely to saythat the Beatles are similar to Ja- justify this approachwith theidea of aconsensus sonFalkner, because the more celebratedband truth,but in reality weare forced into thesituation servesas aprototype againstwhich to measure. 64 Computer MusicJournal Thetriangle inequality canbe violated becauseof successhas been achieved for pitch trackingof ar- themultifaceted nature of similarity: for example, bitrary polyphonic music. MichaelJackson is similar to theJackson Five, his Acousticapproaches analyze the music content Motown roots,and also to Madonna.Both arehuge directly andthus can be applied to anymusic for pop starsof the1980s, but Madonnaand the Jack- whichone has the audio. Blum etal.(1999) present son Five do not otherwisehave much in common. anindexing systembased on matchingfeatures suchas pitch,loudness, or Mel-FrequencyCepstral Coefcients (MFCCs; these are a compactrepresen- Variability andSpan tation of thefrequency spectrum, typically com- putedover short time windows).Foote (1997) has Fewartists are truly asingle‘ ‘point’’ in anyimagi- designeda musicindexing systembased on histo- nablestylistic spacebut undergochanges through- gramsof MFCC featuresderived from adiscrimina- out theircareers and may consciouslyspan tively trainedvector quantizer. Tzanetakis (2002) multiple styleswithin asinglealbum, or evena extractsa varietyof featuresrepresenting the spec- singlesong. Trying to dene a singledistance be- trum, rhythm, andchord changes and concatenates tweenany artist and widely ranging,long-lived mu- theminto asinglevector to determinesimilarity. sicianssuch as David Bowieor Sting seems Loganand Salomon (2001) andAucouturier and unlikely to yield satisfactoryresults. Pachet(2002) model songsusing local clustering of Despiteall of thesedif culties, techniques to au- MFCC features,then determine similarity by com- tomatically determinemusic similarity haveat- paringthe models. Berenzweig,Ellis, andLawrence tractedmuch attention in recentyears (Ghias et al. (2003) usea suiteof patternclassi ers to map 1995; Foote 1997; Tzanetakis2002; Loganand Salo- MFCCs into an anchorspace ,in whichprobability mon 2001; Aucouturierand Pachet 2002; Ellis etal. models are tandcompared. 2002). Similarity lies atthe core of theclassi ca- With thegrowth of theWorld Wide Web,several tion andranking algorithms neededto organizeand techniqueshave emerged that are based on public recommendmusic. Such algorithms could beused dataderived from subjectivehuman opinion (Co- in futuresystems to index vastaudio repositories, henand Fan 2000; Ellis etal.2002). Theseuse
Details
-
File Typepdf
-
Upload Time-
-
Content LanguagesEnglish
-
Upload UserAnonymous/Not logged-in
-
File Pages14 Page
-
File Size-