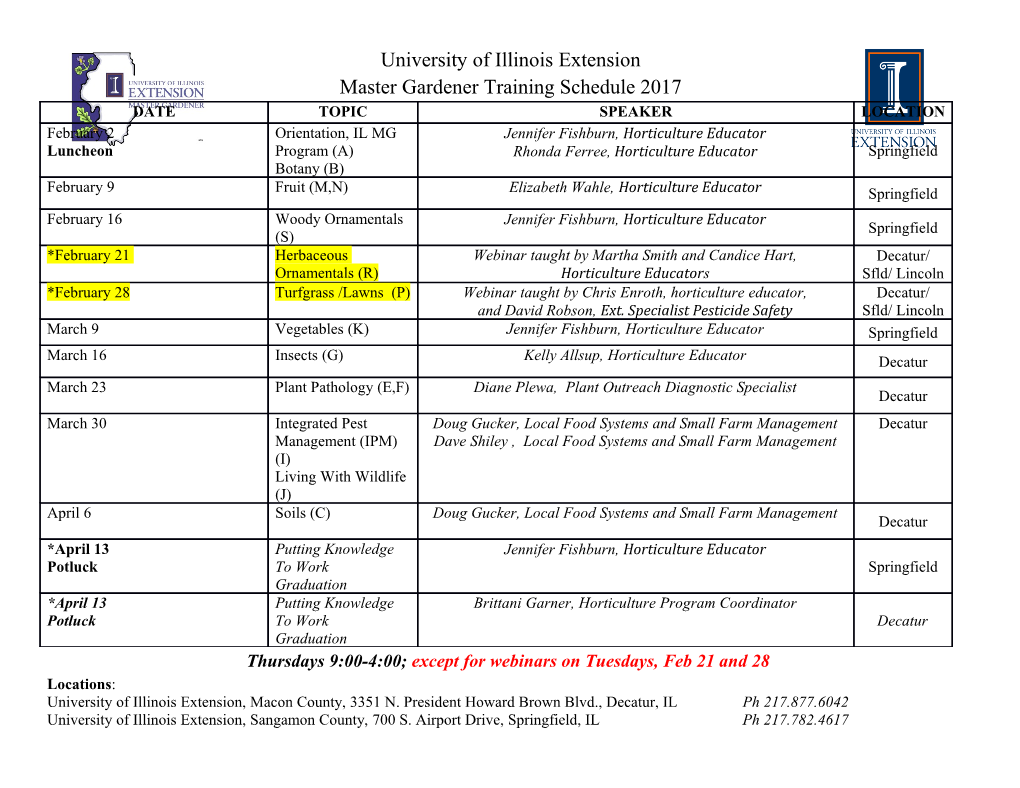
Fast Deduplication Data Transmission Scheme on a Big Data Real-Time Platform Sheng-Tzong Cheng, Jian-Ting Chen and Yin-Chun Chen Department of Computer Science and Information Engineering, National Cheng Kung University, Taiwan {stevecheng1688, eytu0233, darkerduck}@gmail.com Keywords: Big Data, Deduplication, In-Memory Computing, Spark. Abstract: In this information era, it is difficult to exploit and compute high-amount data efficiently. Today, it is inadequate to use MapReduce to handle more data in less time let alone real time. Hence, In-memory Computing (IMC) was introduced to solve the problem of Hadoop MapReduce. IMC, as its literal meaning, exploits computing in memory to tackle the cost problem which Hadoop undue access data to disk caused and can be distributed to perform iterative operations. However, IMC distributed computing still cannot get rid of a bottleneck, that is, network bandwidth. It restricts the speed of receiving the information from the source and dispersing information to each node. According to observation, some data from sensor devices might be duplicate due to time or space dependence. Therefore, deduplication technology would be a good solution. The technique for eliminating duplicated data is capable of improving data utilization. This study presents a distributed real-time IMC platform -- “Spark Streaming” optimization. It uses deduplication technology to eliminate the possible duplicate blocks from source. It is expected to reduce redundant data transmission and improve the throughput of Spark Streaming. 1 INTRODUCTION Combined these overhead costs, it make some algorithms that require fast steps unacceptably slow. In recent years, with the development of Internet and For example, many machine-learning algorithms prevalence of mobile devices, a very huge amount of were required to work iteratively. Algorithms like data was generated daily. To be able to carry out some training a recommendation engine or neural networks operations on larger and more complex data now, and finding natural clusters in data are typically techniques for Big Data were presented. In 2004, iterative algorithms. In addition, if you want to get a Google released a programming model MapReduce real-time result from the trained model or wish to (Dean, 2008) for processing and generating large data monitor program logs to detect failures in seconds, sets with a parallel, distributed algorithm. Packages you will need for computation streaming models that have been developed and widely used nowadays. simplify MapReduce offline processing. Obviously, They can make big-data analysis more efficient. For you want the steps in these kinds of algorithms to be instance, one of the mostly used packages is Hadoop as fast and lightweight as possible. (Shvachko, 2010). It provides an interface to To implement iterative, interactive and streaming implement MapReduce that allows people use it more computing, a parallel in-memory computing platform, easily. Spark (Zaharia, 2010), was presented. Spark is built Hadoop MapReduce adapts coarse-grained tasks on a powerful core of fine-grained, lightweight, and to do its work. These tasks are very heavyweight for abstract operations by which the developers iterative algorithms. Another problem is that previously had to write themselves. Spark is MapReduce has no awareness of the total pipeline of lightweight and easy to build iterative algorithms with Map plus Reduce steps. Therefore, it cannot cache good performance as scale. The flexibility and intermediate data in memory for faster performance. support for iterations also allow Spark to handle event This is because it uses a small circular buffer (default stream processing in a clever way. Originally, Spark 100MB) to cache intermediate data, and it flushes was designed to become a batch mode tool, like intermediate data to disk between each step and when MapReduce. However, its fine-grained nature makes 80% of the circular buffer space is occupied. possible that it can process very small batches of data. 155 Cheng S., Chen Y. and Chen J. 155 Fast Deduplication Data Transmission Scheme on a Big Data Real-Time Platform. DOI: 10.5220/0006528400000000 In Proceedings of the Seventh International Symposium on Business Modeling and Software Design (BMSD 2017), pages 155-164 ISBN: 978-989-758-238-7 Copyright c 2017 by SCITEPRESS – Science and Technology Publications, Lda. All rights reserved Seventh International Symposium on Business Modeling and Software Design BMSD 2017 - Seventh International Symposium on Business Modeling and Software Design Therefore, Spark developed a streaming model to gateways and computing platform to exchange more handle data in short time windows and compute each efficient utilization of network bandwidth. In brief, of them as “mini-batch”. we applied data deduplication technique completely Network bandwidth is another bottleneck that we to improve the data re-use rate on distributed wish to resolve. Bandwidth shortage is not from its computing system like Spark. architecture but from the gateway between sensors and computing platform (Akyildiz, 2002). The bridge that collects data from sensors and transmits data to 2 DATA DEDUPLICATION server is performed by one or more gateways. Their bandwidth is often low because of the wireless TRANSMISSION SCHEME network environment. Our proposal is to utilize these transmitted data fully for low-latency processing In this section, we elaborate on the details of our applications. In order to maintain or even improve the system design. We first clarify our problem in Section throughput of computing platform, we adopt the real- 2.1 and then the implementations and the parameter time parallel computing platform based on data definition are listed in the following sections. In deduplication technology. It allows the efficient Section 2.2, we outline our system overview and utilization of network resources to improve provide a series steps explanation then formulate our throughput. bandwidth saving model. In Section 2.3, we describe Data deduplication is a specialized data how to choose block fingerprint and give a compression technique for eliminating duplicated benchmark for hash functions to compare to select the data. This technique is used to improve storage option. In Section 2.4, we give some concept to guide utilization and can also be applied to network data users how to implement the data chunk preprocess transmission to reduce the amount of bytes that must model. be sent. One of the most common forms of data deduplication implementation works by comparing 2.1 Problem Description chunks of data to detect duplicates. Block deduplication looks within a file and saves distant The main problem we want to resolve is to reduce the blocks. Each chunk of data is processed using a hash duplicated data delivery so that it can send more data algorithm such as MD5 (Rivest, 1992) or SHA-1 in limited time. This problem can be divided into (Eastlake, 2001). This process generates a unique several sub-problems. The first one is that how to number for each piece which is then stored in an index. chunk data so that we can make the set of data blocks If a file is updated, only the changed data is saved. smaller. In other words, when the repetition rate of For instance, Dropbox and Google Drive are also data blocks is higher, the bandwidth saving becomes cloud file synchronization software. Both of them use more. However, if remote does not have similar data, data deduplication technique to reduce the cost of these chunking methods would not effective. storage and transmission between client and The second problem is that how sender decides server. However, unlike those cloud storages, there is whether this data block has received or not. With no similar file between gateway and computing server. Rsync algorithm (Tridgell, 1998), it uses a pair of Hence, we propose a data structure to keep those weak and strong checksums for a data block to enable duplicated part of data and reuse them. This is the part sender to check whether the blocks have not been where our work is different from those cloud storages. modified or not. This gives a good inspiration to solve In our work, the data stream from sensors can be it. In order to find the same data block, Rsync uses regarded as an extension of a file. In other words, the strong checksum to achieve it. So, hash function is the data stream is also divided into blocks to identify solution that is able to digest block into a fingerprint. which blocks are redundant. So data deduplication Block fingerprint can represent the contents of the has quite potentials to resolve the problem of block and utilize less space, this is we want. However, bandwidth inadequate. MD5 used in Rsync is not the best choice for our work. In this study, we propose that the deduplication This will be analyzed in Section 2.3. scheme reduces the requirement of bandwidth and improves throughput on real-time parallel computing 2.2 Scheme Overview platform. Interestingly, the data from sensors has quite duplicated part that can be eliminated. This is Before describing solutions of these sub-problems, the tradeoff between processing speed and network we assemble these notions into a data block bandwidth. We sacrifice some CPU efficacy of deduplication scheme. We believe this scheme helps 156 156 Fast Deduplication Data Transmission Scheme on a Big Data Real-Time Platform Fast Deduplication Data Transmission Scheme on a Big Data Real-Time Platform us to reduce bandwidth utilization between gateway this process needs Block Fingerprint Generator to and computing platform. Figure 1 shows the scheme generate hash value for each block, the detail overview that illustrates how we implement it. implementation is showed in Section 2.3. Here we explain the meaning of control flow and Step 4: The Matches Decision Maker will data flow. In Figure 1, the two biggest dotted boxes exchange metadata with Fingerprint Matcher in arrow represent a remote data source (i.e.
Details
-
File Typepdf
-
Upload Time-
-
Content LanguagesEnglish
-
Upload UserAnonymous/Not logged-in
-
File Pages10 Page
-
File Size-