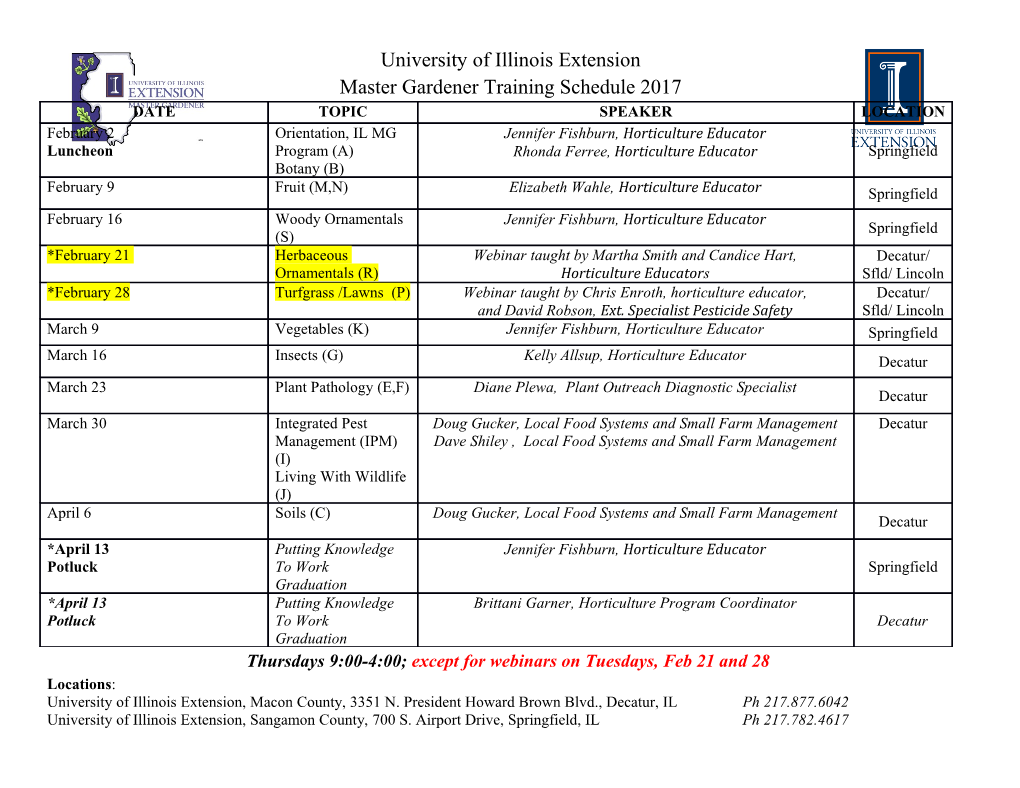
Supplementary Information Supplementary Methods Evaluation of the Affymetrix Rhesus Macaque GeneChip for use on Sooty Mangabey Samples Gene expression in rhesus macaques and sooty mangabeys was monitored using the Affymetrix Rhesus Genome Array which contains probesets for ~47, 000 transcripts (1) and has been tested rigorously by independent primate centers (2) These arrays were designed using rhesus cDNA and genomic rhesus sequences from multiple sources (1). Prior to the availability of rhesus macaque arrays, several groups, including our own, have published microarray studies hybridizing samples from various non-human primates on arrays with probes specific for human sequences, however a central concern has been cross-species specificity of these arrays. At the onset of this study, we were concerned with the ability of the Rhesus Genome Array to accurately hybridize cRNA derived from SMs, and whether data from the arrays would allow meaningful comparison between RM and SM samples. The design of probesets for this array employed an algorithm in which macaque subsequences most orthologous between human and macaque were utilized; to further reduce the effect of polymorphisms, 16 probes per probeset (rather than the customary 11) were used for ~15,000 probesets (1). A recent study comparing 1,180 transcripts between rhesus macaques and humans have estimated the average degree of nucleotide identity to be 97.791.78% in the coding region, and 95.104.15% in the 3’ UTR (3), with less than 1% of genes having lower than 88% homology. To minimize the effect of individual probes with sequence mismatches due to interpecies variation, we 1 used the Robust Multi-Array analysis for normalization and probeset summarization of the dataset. We feel that RMA normalization is the most appropriate method for interspecies comparisons for two reasons: (i) the global background adjustment (as opposed to background correction at the probe level, ie. Perfect Match – Mismatch correction) will reduce the effect of mismatches on expression measurements at the probe level, and (ii) the use of median polish to summarize individual probes into probeset measurements is robust and the effect of individual probes with sequence variation due to interspecies differences is minimized. Lastly, as described in detail below, we limited our analysis to include only genes for which (i) a two-fold change in expression relative to uninfected samples could be detected, and (ii) the gene expression change was determined to be statistically significant. Including the requirement of a two-fold ratio cut-off in our analysis, rather than direct comparison of probeset intensities, would further reduce false-negative comparisons between the species. Based on the high degree of cDNA transcript identity between SMs and RMs, the probe design of the Rhesus GeneChip towards orthologous sequences, and our data analysis approach, we reasoned that the Rhesus GeneChip would be able to accurately detect changes in sooty mangabey transcript levels, and that our analysis would be focused on genes that could be meaningfully compared between species. Nevertheless, to confirm the utililty of the array for our analysis, we employed in silico analyses prior to hybridization and post-analysis validation experiments of our results from the main study. To predict the overall binding ability of the Rhesus GeneChip with SM cDNAs, we performed an in silico analysis of perfect and mismatch binding based on SM sequences in GenBank and individual probes on the array. Due to the variation in 2 annotation of probesets on the Affymetrix RM Array, probesets were matched to genes based on the presence of at least one perfect match within the gene sequence. In some cases multiple genes matched with a single probeset due to the presence of multiple alleles or orthologs of particular genes within the set of sequences. The resultant probesets were then aligned using Clustal to the available sequence and probes within each set were binned based on the number of mismatches and/or indels between the probe and the sequence. Probes with >5 mismatches or containing indels are likely not contained within the query sequence. As detailed in Supplementary Table S2, 54 of the 100 sequences were successfully aligned with probesets on the Affymetrix RM array. These 54 sequences represent 42 unique genes. In almost all cases where probesets aligned with their intended gene (e.g. not a close paralog), the number of perfect match probes outnumbered the probes containing mismatches. Because of the median polish summarization employed by the RMA algorithm, and our estimate that most probesets have a majority of probes that match perfectly with SM sequences, we felt any effect due to interspecies sequence variation on the arrays would be minimal. An independent analysis by (C. Davies, Affymetrix, Inc., pers. communication) verified our findings. To verify that hybridization efficiency was similar between RMs and SMs, we calculated the global statistics of the log10 intensity on all 94 arrays described in this study. The array data was pre-filtered to exclude all probesets representing control sequences, viral genomes or reporter genes. As summarized in Supplementary Table S1, the means, medians, ranges and standard deviations on arrays hybridized with total RNA from SMs (33 arrays) or RMs (61 arrays) were nearly identical, and were very similar to the cumulative statistics from all 94 arrays. The distribution of log10 intensities on each 3 individual array is depicted in Supplementary Figure S1. Similar to the global statistics for each species, the RM arrays have a slightly larger range of expression values compared to those hybridized with SM RNA, and arrays hybridized with SM samples have a moderately higher median intensity; however no gross differences were apparent between species hybridization statistics. Collectively, these data validate our in silico model of hybridization of SM RNA on the Rhesus GeneChips. More importantly, these data indicate that gene expression measurements obtained from hybridizing SM RNA samples to the Rhesus GeneChips is directly comparable to data obtained from RM samples. Microarray data analysis – normalization, principal components analysis, hierarchical clustering Arrays were scanned using the Affymetrix GeneChip Scanner 3000 7G, and visual inspection and evaluation of overall intensity was used to judge the quality of the hybridization; 2 of 96 total arrays were judged to be of low quality and removed from the analysis. .CEL files from the remaining Rhesus GeneChip arrays were imported into Partek Genomics Suite v.6.4 (Partek, Inc. St. Louis, MO) and RMA pre-processing was used to perform global background correction, quantile normalization and median polish probeset summarization. Probeset annotation was based on NetAffx Annotation Release 27 (November 2008, Affymetrix, Inc.) which utilizes the most update-to-date build of the rhesus macaque genome (Unigene Macaca mulatta Build #13, September, 2008). After generation of smaller gene lists (ie. One-way ANOVA to define SIV-inducible genes in SMs), probeset annotations were further updated using the DAVID annotation tool 4 (http://david.abcc.ncifcrf.gov/) (3-4). For probesets with no Unigene rhesus macaque identifier, the Gene Symbol for the human orthologue was used. Similarity between samples was estimated using principal component analysis was used to reduce the log10 intensities on individual arrays and using a covariance dispersion matrix and normalized eigenvector scaling. PC#1 (37.4%), PC#2 (11.7%) and PC#3 (7.8%) accounted for 56.9% of the variance in the dataset. Hierarchical clusters between samples or between genes were assembled using Euclidean metric and average linkage to determine distance between datasets and clusters, respectively. Identification of genes induced by SIV infection and genes with differential SIV regulation between species One-way ANOVA was used to test for differences in the log10 intensities of individual probesets in the sooty mangabey group (33 arrays) after infection, and the P-value was corrected for multiple hypothesis testing using the Benjamini-Hochberg False Discovery Rate method. This corrected P-value was 0.0008342 was used in combination with a second criteria, a two-fold change up or down, in the average gene expression relative to pre-infected samples (log10 time X – Log10 time 0 > 0.301 or < -0.301) to define differentially expressed genes. The same algorithm was used to identify differentially expressed genes in the SIVmac239-infected RM group (36 arrays, corrected P-value = 0.00746). Although fairly stringent by current standards, this approach allowed us to have a high degree of confidence in probesets/genes defined as differential. The utility of our approach in identifying genes with SIV-inducible expression in SMs and RMs was 5 supported by the high degree of overlap between our SM/RM dataset and concurrent, independent, studies detailing SIV infection in AGMs (5) and previously published observations from our group in SHIV89.6P infected cynomolgus macaques (6). Because of the differences in sample sizes between the SIVsmm SM (33 arrays), SIVsmm RM (25 arrays) and SIVmac239 RM (36 arrays) groups, a direct Boolean analysis to determine SIV-regulated genes that differed between species would not be appropriate; exclusion of a gene from one group and not another may be due to differing P-values rather than underlying biology. Instead, we employed a two-layered approach. In the first step, we used a two-way ANOVA to define genes with expression that differed (i) between the three infection groups, and (ii) over time after infection. This gene list was further reduced by a post-hoc filter to include only genes that also exhibited significantly different expression values between SMs and both RM groups. This initial gene list was large (>20,000 probesets) and we reasoned that it included genes with differential expression due to (i) species-specific differences in constitutive expression, (ii) differing hybridization efficiency of genes with sequence disparity, or (iii) distinct patterns of transcription in response to SIV infection.
Details
-
File Typepdf
-
Upload Time-
-
Content LanguagesEnglish
-
Upload UserAnonymous/Not logged-in
-
File Pages61 Page
-
File Size-