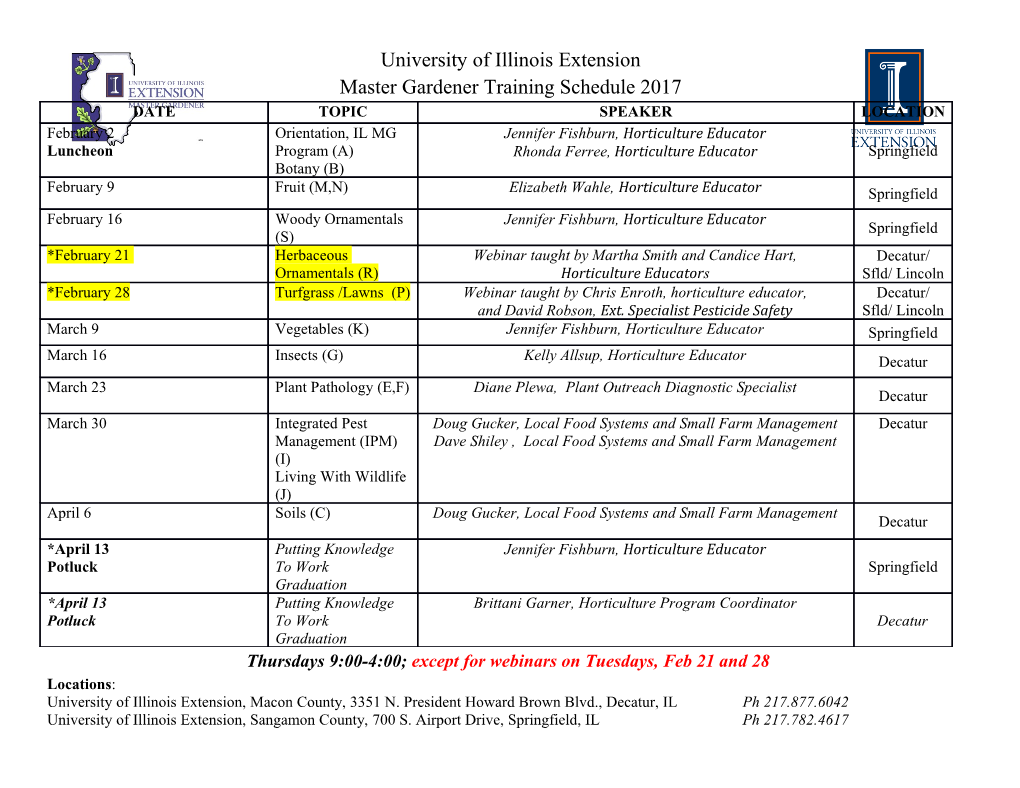
University of Central Florida STARS Electronic Theses and Dissertations, 2020- 2020 Improving Usability of Genetic Algorithms through Self Adaptation on Static and Dynamic Environments Reamonn Norat University of Central Florida Part of the Computer Engineering Commons Find similar works at: https://stars.library.ucf.edu/etd2020 University of Central Florida Libraries http://library.ucf.edu This Masters Thesis (Open Access) is brought to you for free and open access by STARS. It has been accepted for inclusion in Electronic Theses and Dissertations, 2020- by an authorized administrator of STARS. For more information, please contact [email protected]. STARS Citation Norat, Reamonn, "Improving Usability of Genetic Algorithms through Self Adaptation on Static and Dynamic Environments" (2020). Electronic Theses and Dissertations, 2020-. 107. https://stars.library.ucf.edu/etd2020/107 IMPROVING USABILITY OF GENETIC ALGORITHMS THROUGH SELF ADAPTATION ON STATIC AND DYNAMIC ENVIRONMENTS by REAMONN NORAT B.S. Embry-Riddle Aeronautical University, 2013 A thesis submitted in partial fulfilment of the requirements for the degree of Master of Science in the Department of Electrical and Computer Engineering in the College of Engineering and Computer Science at the University of Central Florida Orlando, Florida Spring Term 2020 c 2020 Reamonn Norat ii ABSTRACT We propose a self-adaptive genetic algorithm, called SAGA, for the purposes of improving the us- ability of genetic algorithms on both static and dynamic problems. Self-adaption can improve usability by automating some of the parameter tuning for the algorithm, a difficult and time- consuming process on canonical genetic algorithms. Reducing or simplifying the need for pa- rameter tuning will help towards making genetic algorithms a more attractive tool for those who are not experts in the field of evolutionary algorithms, allowing more people to take advantage of the problem solving capabilities of a genetic algorithm on real-world problems. We test SAGA and analyze its the behavior on a variety of problems. First we test on static test problems, where our focus is on usability improvements as measured by the number of parameter configurations to tune and the number of fitness evaluations conducted. On the static problems, SAGA is compared to a canonical genetic algorithm. Next, we test on dynamic test problems, where the fitness landscape varies over the course of the problem’s execution. The dynamic prob- lems allows us to examine whether self-adaptation can effectively react to ever-changing and un- predictable problems. On the dynamic problems, we compare to a canonical genetic algorithm as well as other genetic algorithm methods that are designed or utilized specifically for dynamic prob- lems. Finally, we test on a real-world problem pertaining to Medicare Fee-For-Service payments in order to validate the real-world usefulness of SAGA. For this real-world problem, we compare SAGA to both a canonical genetic algorithm and logistic regression, the standard method for this problem in the field of healthcare informatics. We find that this self-adaptive genetic algorithm is successful at improving usability through a large reduction of parameter tuning while maintaining equal or superior results on a majority of the problems tested. The large reduction of parameter tuning translates to large time savings for iii users of SAGA. Furthermore, self-adaptation proves to be a very capable mechanisms for dealing with the difficulties of dynamic environment problems as observed by the changes to parameters in response to changes in the fitness landscape of the problem. iv TABLE OF CONTENTS LIST OF FIGURES . xi LIST OF TABLES . xiv CHAPTER 1: INTRODUCTION . 1 CHAPTER 2: LITERATURE REVIEW . 6 CHAPTER 3: METHODOLOGY . 12 3.1 Canonical Genetic Algorithm . 12 3.1.1 Representation and Initialization . 13 3.1.2 Fitness . 13 3.1.3 Parent Selection . 14 3.1.4 Genetic Operators: Crossover and Mutation . 15 3.2 Self-Adaptive Genetic Algorithm . 16 3.2.1 Encoding of Self-adaptive Parameters . 16 3.2.2 Self-Adaption of Parent Selection Pressure . 18 3.2.3 Self-Adaption of Crossover and Mutation Rate . 19 v 3.2.4 Self-Adaption of Crossover and Mutation Operator Selection . 19 3.2.4.1 Encoding of Self-Adaptive Crossover and Mutation Operators . 19 3.2.4.2 Descriptions of Mutation and Crossover Operators . 21 3.2.4.3 SAGA Operator Selection method . 25 CHAPTER 4: STATIC PROBLEMS . 29 4.1 Motivation . 29 4.2 Experimental Setup . 30 4.2.1 Problems . 30 4.2.2 CGA Setup . 34 4.2.3 SAGA Setup . 35 4.3 Tuning For the Best Parameter Configurations . 36 4.3.1 CGA Parameter Tuning . 36 4.3.2 SAGA Parameter Tuning . 39 4.4 Results . 40 4.4.1 Equal Generations . 41 4.4.1.1 Fitness Comparison . 41 4.4.1.2 Number of Fitness Evaluations Comparison . 44 vi 4.4.1.3 Likelihood of a CGA Configuration Finding Superior Fitness Than SAGA . 46 4.4.2 Extended SAGA Generations . 49 4.4.2.1 Fitness Comparison . 50 4.4.2.2 Number of Fitness Evaluations Comparison . 52 4.4.2.3 Likelihood of a CGA Configuration finding superior fitness than SAGA . 54 4.4.3 Summary of Results . 55 4.5 Analysis of the Self-Adaptive Parameters . 56 4.5.1 SAGA Parent Selection Tournament Size . 57 4.5.2 SAGA Operator Rates . 59 4.5.3 SAGA Operator Usage . 62 4.5.3.1 Crossover Operator Usage . 63 4.5.3.2 Mutation Operator Usage . 67 4.6 Conclusions for SAGA on Static Problems . 71 CHAPTER 5: DYNAMIC PROBLEMS . 73 5.1 Motivation . 73 5.2 Experimental Setup . 74 vii 5.2.1 Problems . 74 5.2.2 Algorithms . 78 5.2.2.1 Problem Representation . 79 5.2.2.2 Self Adaptive Genetic Algorithm . 79 5.2.2.3 Canonical Genetic Algorithm . 79 5.2.2.4 Triggered Hypermutation . 80 5.2.2.5 Random immigrants . 81 5.2.2.6 Clearing . 82 5.2.2.7 ACROMUSE . 83 5.2.3 Dynamic Metrics . 84 5.2.3.1 Collective Mean Fitness . 84 5.2.3.2 Moment of Inertia Diversity Measurement . 85 5.3 Results . 86 5.3.1 Summary of Results . 108 5.4 Analysis of the Self-Adaptive Parameters . 109 5.4.1 SAGA Parent Selection Tournament Size . 110 5.4.2 SAGA Operator Rates . 112 viii 5.4.3 SAGA Operator Usage . 116 5.5 Conclusions for SAGA on Dynamic Problems . 121 CHAPTER 6: REAL-WORLD MEDICARE DATA EXPERIMENT . 124 6.1 Motivation . 124 6.2 Experimental Setup . 125 6.2.1 Problem . 126 6.2.2 Logistic Regression . 129 6.2.3 Genetic Algorithm Implementation . 129 6.2.3.1 Fitness Function . 130 6.3 Results . 131 6.4 Analysis of Results . 136 6.4.1 Coefficient Analysis . 137 6.4.2 Input Analysis . 141 6.4.3 Significant Variables Analysis . 143 6.5 Conclusions for SAGA on Real-World Medicare Problem . 150 CHAPTER 7: CONCLUSIONS . 152 ix LIST OF REFERENCES . 156 x LIST OF FIGURES Figure 3.1: Illustration of the two chromosome individual of SAGA. 16 Figure 3.2: Plots of the children created from each crossover operator. 23 Figure 3.3: SAGA crossover operator usages from different operator selection methods. 28 Figure 4.1: Box plots of fitness results from the best configurations of CGA and SAGA at 500 generations. 43 Figure 4.2: Comparison plot of all CGA configurations to the best SAGA configuration. 48 Figure 4.3: Box plots of fitness results from the best configurations of CGA and SAGA with SAGA running for extended generations. 53 Figure 4.4: Mean parent selection tournament size across all runs of the best SAGA configuration on the static problems. 58 Figure 4.5: Mean crossover and mutation rates across all runs of the best SAGA config- uration on the static problems. 60 Figure 4.6: Mean crossover operator usages across all runs of the best SAGA configu- ration on the static problems. 66 Figure 4.7: Mean mutation operator usages across all runs of the best SAGA configura- tion on the static problems. 70 Figure 5.1: Problem 1 fitness, CMF, and MoI plots. 89 xi Figure 5.2: Problem 2 fitness, CMF, and MoI plots. 91 Figure 5.3: Problem 3 fitness, CMF, and MoI plots. 94 Figure 5.4: Problem 4 fitness, CMF, and MoI plots. 96 Figure 5.5: Problem 5 fitness, CMF, and MoI plots. 98 Figure 5.6: Problem 6 fitness, CMF, and MoI plots. ..
Details
-
File Typepdf
-
Upload Time-
-
Content LanguagesEnglish
-
Upload UserAnonymous/Not logged-in
-
File Pages182 Page
-
File Size-