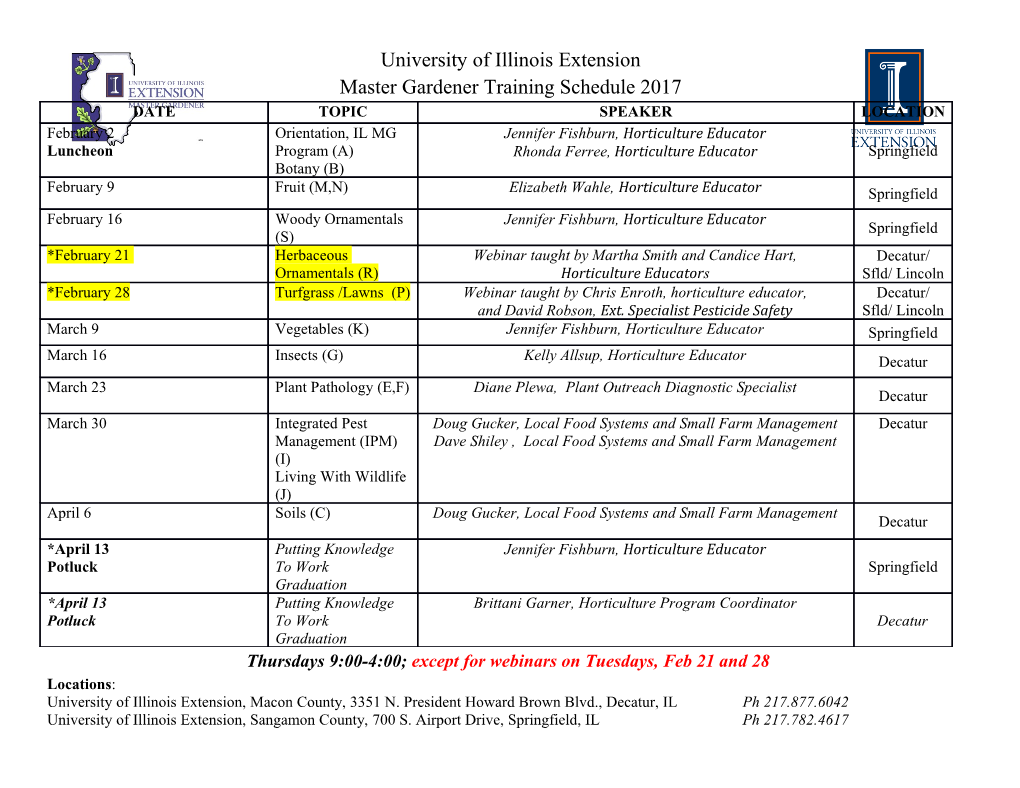
Confidence Distribution (CD) and an unifying framework for BFF inferences (Part I: An Introduction to CD & Part II: CD is an effective inference tool for fusion learning) Min-ge Xie (Part I) Regina Liu (Part II) Department of Statistics & Biostatistics, Rutgers University International FocuStat Workshop on CDs and Related Themes Oslo, Norway 2015 Research supported in part by grants from NSF First peek of Bayesian/Fiducial/Frequentist (BFF) inferences Question: What are in common among the following BFF statistical tools/concepts? Bayesian posterior distribution (B) Fiducial distribution (Fi) Likelihood function or normalized likelihood L(θjdata)= R L(θjdata)dθ – assume the integration exists (Fr/B) Bootstrap distribution (Fr) p-value function (Fr) One-sided H0: θ = t vs H1: θ > t =) p-value p = p(t); varying t in Θ =) p(t) is called a p-value function. Belief/plausible functions (Dempster-Shafer/IMs) (Fi/Fr) ······ A common name for such sample-dependent functions? — Confidence distributions (CDs) In the talks – I A more formal treatment of CD concept; combining information; examples as effective tools; relations to fiducial and Bayes I Stress added values – Provide solutions for problems whose solutions are previously unavailable, unknown or not easily available. First peek of Bayesian/Fiducial/Frequentist (BFF) inferences They all — are sample-dependent (distribution) functions on the parameter space. can often be used to make statistical inference, especially o can be used to construction confidence (credible?) intervals of all levels, exactly or asymptotically. In the talks – I A more formal treatment of CD concept; combining information; examples as effective tools; relations to fiducial and Bayes I Stress added values – Provide solutions for problems whose solutions are previously unavailable, unknown or not easily available. First peek of Bayesian/Fiducial/Frequentist (BFF) inferences They all — are sample-dependent (distribution) functions on the parameter space. can often be used to make statistical inference, especially o can be used to construction confidence (credible?) intervals of all levels, exactly or asymptotically. A common name for such sample-dependent functions? — Confidence distributions (CDs) First peek of Bayesian/Fiducial/Frequentist (BFF) inferences They all — are sample-dependent (distribution) functions on the parameter space. can often be used to make statistical inference, especially o can be used to construction confidence (credible?) intervals of all levels, exactly or asymptotically. A common name for such sample-dependent functions? — Confidence distributions (CDs) In the talks – I A more formal treatment of CD concept; combining information; examples as effective tools; relations to fiducial and Bayes I Stress added values – Provide solutions for problems whose solutions are previously unavailable, unknown or not easily available. Estimation viewpoint – Parameter estimation & confidence distribution (CD) Statistical inference: Point estimate Interval estimate Distribution estimate (e.g., confidence distribution) Example: X1;:::; Xn i.i.d. follows N(µ, 1) ¯ 1 Pn Point estimate: xn = n i=1 xi p p Interval estimate: (x¯n − 1:96= n; x¯n + 1:96= n) ¯ 1 Distribution estimate: N(xn; n ) I The idea of the CD approach is to use a sample-dependent distribution (or density) function to estimate the parameter of interest. One-sided test: K0 : µ = µ0 vs Ka : µ > µ0 p p ¯ p(µ0) = P(X > x¯n) = 1 − Φ( nfx¯n − µ0g) = Φ( nfµ0 − x¯ng): ¯ 1 Varying µ0 2 Θ! =) Cumulative distribution function of N(xn; n )! ? Suppose n = 100 and we observe x¯n = 0:3 mu0 iny -0.1 0 0.1 0.1355 0.2 0.3 0.4645 0.496 P-value 0 .00002 .00135 0.02275 0.05 .15866 0.5 0.95 0.975 -0.1 0 0.2 0.3 0.496 ¯ 1 Example: Many ways to obtain "distribution estimate" N(xn; n ) Method 1 & 2: Bayes & fiducial (omit here ...) Method 3: P-value method ? Suppose n = 100 and we observe x¯n = 0:3 mu0 iny -0.1 0 0.1 0.1355 0.2 0.3 0.4645 0.496 P-value 0 .00002 .00135 0.02275 0.05 .15866 0.5 0.95 0.975 -0.1 0 0.2 0.3 0.496 ¯ 1 Example: Many ways to obtain "distribution estimate" N(xn; n ) Method 1 & 2: Bayes & fiducial (omit here ...) Method 3: P-value method One-sided test: K0 : µ = µ0 vs Ka : µ > µ0 p p ¯ p(µ0) = P(X > x¯n) = 1 − Φ( nfx¯n − µ0g) = Φ( nfµ0 − x¯ng): ¯ 1 Varying µ0 2 Θ! =) Cumulative distribution function of N(xn; n )! mu0 iny -0.1 0 0.1 0.1355 0.2 0.3 0.4645 0.496 P-value 0 .00002 .00135 0.02275 0.05 .15866 0.5 0.95 0.975 -0.1 0 0.2 0.3 0.496 ¯ 1 Example: Many ways to obtain "distribution estimate" N(xn; n ) Method 1 & 2: Bayes & fiducial (omit here ...) Method 3: P-value method One-sided test: K0 : µ = µ0 vs Ka : µ > µ0 p p ¯ p(µ0) = P(X > x¯n) = 1 − Φ( nfx¯n − µ0g) = Φ( nfµ0 − x¯ng): ¯ 1 Varying µ0 2 Θ! =) Cumulative distribution function of N(xn; n )! ? Suppose n = 100 and we observe x¯n = 0:3 ? Suppose n = 4; Observe a sample with mean x¯obs = 2:945795: Area = 1 ¯ 1 Example: Many ways to obtain "distribution estimate" N(xn; n ) Method 4: Normalizing likelihood function Likelihood function 1 P 2 n 2 1 P 2 Y − (xi −µ) − (x¯−µ) − (xi −x¯) L(µjdata) = f (xi jµ) = Ce 2 = Ce 2 2 Normalized with respect to µ L(µjdata) 1 − n (x¯−µ)2 R = e 2 L(µjdata)µ p2π=n ¯ 1 It is the density of N(xn; n )! Area = 1 ¯ 1 Example: Many ways to obtain "distribution estimate" N(xn; n ) Method 4: Normalizing likelihood function Likelihood function 1 P 2 n 2 1 P 2 Y − (xi −µ) − (x¯−µ) − (xi −x¯) L(µjdata) = f (xi jµ) = Ce 2 = Ce 2 2 Normalized with respect to µ L(µjdata) 1 − n (x¯−µ)2 R = e 2 L(µjdata)µ p2π=n ¯ 1 It is the density of N(xn; n )! ? Suppose n = 4; Observe a sample with mean x¯obs = 2:945795: Inference using CD: Point estimators, Intervals, p-values & more b Review on CD (Xie & Singh 2013, Int. Stat. Rev.) Cox (2013, Int. Stat. Rev.): The CD approach is “to provide simple and interpretable summaries of what can reasonably be learned from data (and an assumed model).” Efron (2013, Int. Stat. Rev.): The CD development is “a grounding process” to help solve “perhaps the most important unresolved problem in statistical inference” on “the use of Bayes theorem in the absence of prior information.” Confidence distribution Efron (1998, Statist. Sci.): “... but here is a safe prediction for the 21st century: ... I believe there is a good chance that ... something like fiducial inference will play an important role ... Maybe Fisher’s biggest blunder will become a big hit in the 21st century!” o Bootstrap distributions are “distribution estimators” and “confidence distributions.” Confidence distribution Efron (1998, Statist. Sci.): “... but here is a safe prediction for the 21st century: ... I believe there is a good chance that ... something like fiducial inference will play an important role ... Maybe Fisher’s biggest blunder will become a big hit in the 21st century!” o Bootstrap distributions are “distribution estimators” and “confidence distributions.” Review on CD (Xie & Singh 2013, Int. Stat. Rev.) Cox (2013, Int. Stat. Rev.): The CD approach is “to provide simple and interpretable summaries of what can reasonably be learned from data (and an assumed model).” Efron (2013, Int. Stat. Rev.): The CD development is “a grounding process” to help solve “perhaps the most important unresolved problem in statistical inference” on “the use of Bayes theorem in the absence of prior information.” Confidence distribution has a long history Long history: an uncertainty measure on parameter space without any prior Fraser (2011) suggested the seed idea (alas, fiducial idea) can be traced back to Bayes (1763) and Fisher (1922). The term “confidence distribution” appeared as early as 1937 in a letter from E.S. Pearson to W.S. Gosset (Student); the first use of the term in a formal publication is Cox (1958). No precise yet general definition for a CD in the classical literature Most commonly used approach is via inverting the upper limits of a whole set of lower-side confidence intervals, often using some special examples (e.g., Efron, 1993, Cox, 2006) Little attention on CDs in the past Historic connection to fiducial distribution, which is largely considered as “Fisher’s biggest blunder” (see, Efron, 1998) Not yet seriously looked at the possible utilities of CDs especially in applications. Renewed interest Recent developments Entirely within the frequentist school — Not attempt to derive a new “paradox free” fiducial theory (not possible!) Focus on providing frequentist inference tools for problems whose solutions are previously unavailable or unknown. Renewed interest 21st century developments of confidence distributions & related topics Confidence distributions (Schweder & Hjort 2002, 2015; Singh, Xie & Strawderman 2005, 2007; Xie & Singh 2013) Bayes/objective Bayes (Berger 2006, Dongchu’s book with Jim 2015(?), etc.) Dempster-Shafer calculus (Dempster 2008); Inferential Models (Liu & Martin 2009, 2012, 2014) Generalized inference/generalized fiducial inference (Weerahandi 1989, 1993; Hannig 2009, 2013; Hannig & Lee 2009); (* above only cited some ‘review’ papers and books) Current research focus — while paying attention to new developments of theoretical frameworks, Emphasize on applications — Focus on to provide frequentist inference tools for problems whose solutions are previously unavailable or unknown. Can these all lead to a brighter new future? Now, with the emerging BFF, Can we really unify the foundation of statistical inferences? “Can our desire to find a unification of Bayesian, Frequentist and Fiducial (BFF) perspectives do the same trick, allowing all of us to thrive under one roof as BFFs (Best Friends Forever)?” - Meng (2014, IMS Bulletin) Classical notion: confidence distribution Classical CD function (e.g., Efron 1993, Cox 2006): Let (−∞; ξn(α)] be a 100α% lower side confidence interval for θ, at every level α 2 (0; 1) Assume the ξn(α) = ξn(Xn; α) is continuous and increasing in α.
Details
-
File Typepdf
-
Upload Time-
-
Content LanguagesEnglish
-
Upload UserAnonymous/Not logged-in
-
File Pages55 Page
-
File Size-