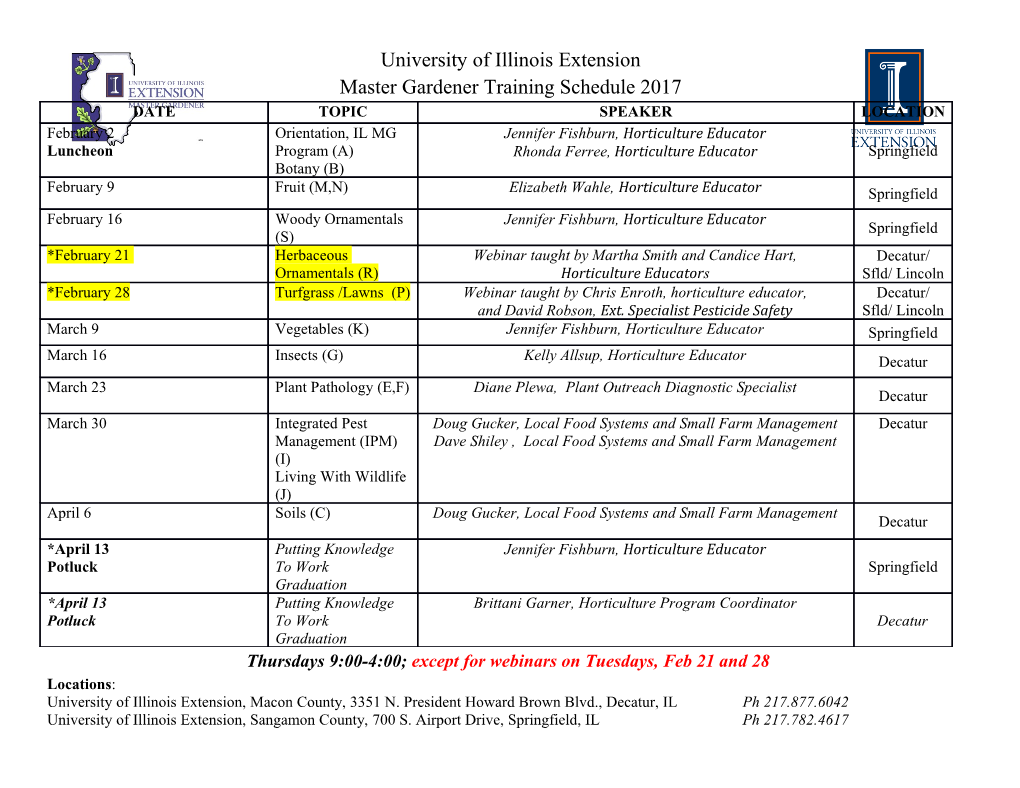
.............................................................................................................................................................................................................................................. PROFESSION SYMPOSIUM .............................................................................................................................................................................................................................................. I Saw You in the Crowd: Credibility, Reproducibility, and Meta-Utility Nate Breznau, University of Bremen .............................................................................................................................................................................................................................................. rowdsourcing is a new word. Google Books’ of scientific value (Berinsky, Huber, and Lenz 2012). Block- first recorded usage of “crowdsourcing” was in chain technology uses decentralized crowd computing. Many 1999.1 It originally referenced Internet users actions that crowds already take (e.g., “Tweeting” and “geo- adding content to websites, the largest example tagging” images) offer free possibilities to test hypotheses of being Wikipedia (Zhao and Zhu 2014). As a social and political relevance (Nagler and Tucker 2015; Cscientific practice, its roots go back to open-engineering com- Salesses, Schechtner, and Hidalgo 2013). Wikipedia had fan- petitions in the 1700s, when the collective ideas of many tastic success, given the strong willingness of publics around overcame otherwise insurmountable problems of the few. the world to add and monitor content. The Wiki model is the Openly tapping into a large population breaks down barriers basis for all types of crowd creations.4 There are the Wikiver- of time, money, and data. The solution is simple. A centralized sity5 crowdsourcing-teaching resources and the Replication- investigator or team poses a question or problem to the crowd. Wiki6 for listing and identifying replications across the social Sometimes tasks are co-creative, such as the programming of sciences (Höffler 2017). In a similar vein, there are efforts to packages by statistical software users and the development of crowdsource open peer review and ideas for what researchers Wiki content. Other times, they are discrete, such as asking should study from potential stakeholders outside of science. individuals to donate computing power,2 respond to a survey, Some scholars might question why many researchers are or perform specific tasks. Using crowdsourced methods, sci- necessary when we have so many preexisting datasets and a entists, citizens, entrepreneurs, and even governments more single researcher can run every possible statistical model con- effectively address societies’ most pressing problems (e.g., figuration. Crowdsourcing researchers’ time and effort may cancer and global warming) (Howe 2008). seem inefficient when machine learning is so advanced. How- Although the public comprises the typical crowd, new ever, if a single scholar or machine throws all possible variables landmark studies involved researchers crowdsourcing other into their models like a “kitchen sink,” it is only efficient at researchers. The human genome project, for example, involved maximizing prediction. There is a subtle but crucial difference major byline contributions from almost 3,000 researchers. In between human research, where if X predicts Y,itmight be a the social sciences, crowdsourcing of researchers is brand new. meaningful association, versus the machine approach, where if In a 2013 study, Silberzahn et al. (2018) convened researchers X predicts Y,itis meaningful (Leamer 1978). When humans or from across disciplines and geographic locations to analyze machines start running every possible model, which is the the same dataset to discover whether football referees issued typical machine-learning strategy, they implicitly test every more red cards to darker-skinned players.3 Klein et al. (2014) possible hypothesis and potentially every possible theory. sourced laboratories across the world to try and reproduce Among all possible models, there will be some in which the several high-profile experimental psychological studies. In the data-generating process is theoretically impossible, such as Crowdsourced Replication Initiative, Breznau, Rinke, and predicting biological sex as an outcome of party affiliation, or Wuttke et al. (2019) demonstrated that crowdsourcing also is right-party votes in 1970 from GDP in 2010. If we want some- useful for replication, structured online deliberation, and thing more than predictive power, human supervision and macro-comparative research. Studies like these are a new causal inference are necessary to rule out impossible realities paradigm within social, cognitive, and behavioral research. that a computer cannot identify on its own (Pearl 2018). In the knowledge business, crowdsourcing is highly cost Crowdsourcing is epistemologically different than machine effective. The average researcher lacks the means to survey or learning. It is not a “kitchen-sink” method. The landmark conduct experiments with samples outside of universities. Silberzahn et al. (2018) study demonstrated that seemingly Crowdsourcing platforms such as Amazon’s Mechanical Turk innocuous decisions in the data-analysis phase of research can (mTurk) changed this by creating access to even-more- change results dramatically. The effect of idiosyncratic fea- convenient convenience samples from all over the world tures of researchers (e.g., prior information and beliefs) are (Palmer and Strickland 2016). Others use these platforms to something Bayesian researchers raised warning signals about crowdsource globally competitive labor to perform paid tasks long ago (Jeffreys 1998 [1939]). This is echoed in more recent © The Author(s), 2020. Published by Cambridge University Press on behalf of the American Political Science Association. This is an Open Access article, distributed under the terms of the Creative Commons Attribution licence (http://creativecommons.org/licenses/by/4.0/), which permits unrestricted re-use, distribution, and reproduction in any medium, provided doi:10.1017/S1049096520000980 the original work is properly cited. PS • April 2021 309 ............................................................................................................................................................................................................................................... .............................Profession Symposium:..................................Opening................................... Political............................. Science ............................................................................................................... discussions about the unreliability and irreproducibility of remains to be seen how valuable the machines are with research (Dewald, Thursby, and Anderson 1986; Gelman and minimal human interference. Loken 2014; Wicherts et al. 2016). However, grappling with At the heart of the SCORE project are questions of cred- this uncertainty is problematic. Identification of informative ibility and reliability. These topics also are at the center of research priors usually involves consulting an expert, such as scandals and conflicts that are infecting science at the moment the researcher doing the work, or using simulations wherein and rapidly increasing researchers and funding agencies’ the user selects parameters that may (or may not) reflect the interests in replication (Eubank 2016; Ishiyama 2014; Laitin Crowdsourcing is epistemologically different than machine learning. It is not a “kitchen-sink” method. reality of the researcher’s decisions. The primary objection is and Reich 2017; Stockemer, Koehler, and Lentz 2018). How- that the distribution of prior beliefs across researchers is ever, there are limits to what replications can offer, and they unknown (Gelman 2008). The Silberzahn et al. (2018) and take on diverse formats and goals (Clemens 2015; Freese and Breznau, Rinke, and Wuttke (2018; 2019) studies provide a real Peterson 2017). Currently, the decision of whether, what, and observation of these priors because all researchers develop how to replicate is entirely a researcher’s prerogative. Few what should be at least a plausible model for testing the engage in any replications, meaning that any given original hypothesis, given the data. This observation extends beyond study is fortunate to have even one replication.8 Journals thus prior studies because the crowdsourced researchers scrutin- far have been hesitant to publish replications, especially of ized the other researchers’ models before seeing the results. their own publications, even if the replications overturn This practice is extremely useful because it is not one expert preposterous-sounding claims (e.g., precognition) (Ritchie, guessing about the relative value of a model but rather poten- Wiseman, and French 2012) or identify major mistakes in tially 100 or more. the methods (Breznau 2015; Gelman 2013). Crowdsourcing The reduction of model uncertainty is good from a Bayes- could change this because it provides the power of meta- ian perspective but it also demands theory from a causal replication in one study. Moreover, crowdsourcing might perspective. Statisticians are well aware that without the appear to journal editors as cutting-edge research rather than correct model
Details
-
File Typepdf
-
Upload Time-
-
Content LanguagesEnglish
-
Upload UserAnonymous/Not logged-in
-
File Pages6 Page
-
File Size-