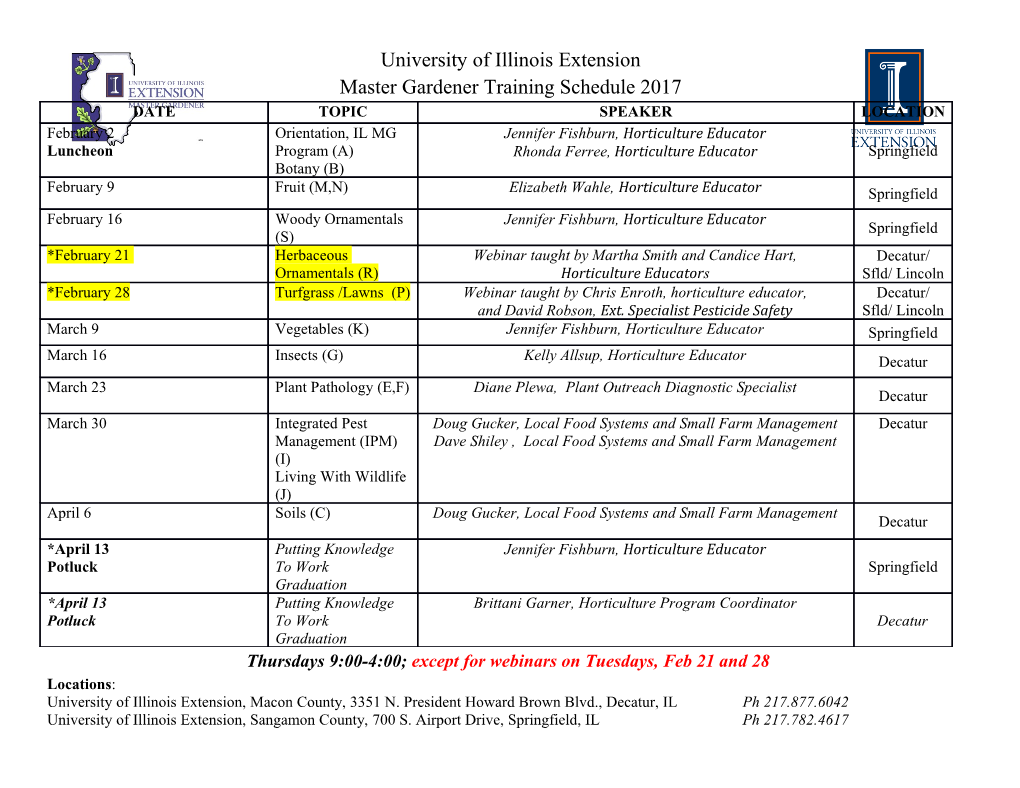
1430 Cook · UP WITH ODDS RATIOS! SPECIAL CONTRIBUTIONS Advanced Statistics: Up with Odds Ratios! A Case for Odds Ratios When Outcomes Are Common Thomas D. Cook, PhD Abstract Treatment comparisons from clinical studies involving cause odds ratios differ from risk ratios and are fre- dichotomous outcomes are often summarized using risk quently interpreted incorrectly as risk ratios. In this ar- ratios. Risk ratios are typically used because the under- ticle, the author contends that risk ratios can be easily lying statistical model is often consistent with the un- misinterpreted and that, in many cases, odds ratios derlying biological mechanism of the treatment and they should be preferred, especially in studies in which out- are easily interpretable. The use of odds ratios to sum- comes are common. Key words: odds ratios; risk ratios; marize treatment effects has been discouraged, especially statistics; differences; outcomes. ACADEMIC EMER- in studies in which outcomes are common, largely be- GENCY MEDICINE 2002; 9:1430±1434. In a 1999 article, Schwartz and colleagues1 com- covariate-adjustedÐwas reported using ORs.) ment on the reporting of a study of the effect of Overall mortality in patients receiving DCLHb (see gender and race on physicians' recommendations the ®rst two columns of Table 1) was 46% (24/52) for cardiac catheterization.2 They contend that, as and mortality in patients receiving normal saline reported, the differences between African Ameri- (control) was 17% (8/46). The risk ratio (RR) is the cans and whites, and between men and women, in ratio of these mortality rates, RR = 2.65 = 46%/17%. rates of referral for cardiac catheterization study Conversely, the odds of mortality is the ratio of the were overstated (Schwartz et al. indicate other rea- mortality rate to the survival rate, or equivalently, sons why this ®nding may be misleading, but they the ratio of the number of deaths to the number of are not relevant to this discussion. As the authors survivors. In the DCLHb study, the odds are 0.857 suggest, formal comparisons of rates in this setting = 24/28 and 0.211 = 8/38 in the DCLHb and control 1 may not even be meaningful). Schwartz et al. groups, respectively. The odds ratio is the ratio of blame this overstatement, in part, on the use of odds in the two groups, OR = 4.07 = 0.857/0.211. odds ratios (ORs) to summarize the results and ar- These measures are numerically quite different and, gue against such use. Additionally, a number of hence, must be interpreted differently. As discussed other articles have appeared in recent years dis- below, each requires careful consideration and each couraging the use of ORs for reporting the results can be easily misinterpreted. 3±9 of medical studies, especially when outcomes are Criticisms of ORs fall principally into two cate- common. Very little has been published since then gories: 1) ORs are not as intuitive as RRs and, there- 10 to refute this recommendation. As is argued be- fore, are dif®cult to understand and easily misin- low, in the study cited above and when properly terpreted and misapplied, and 2) ORs often differ interpreted, ORs may be the most meaningful sum- signi®cantly from RRs. Arguments of the ®rst cat- mary measures of the differences observed. egory are important, but they suffer from a major As an illustration, consider the results of a recent ¯aw. Risk ratios may seem intuitive and easily ap- study of diaspirin cross-linked hemoglobin plied; however, they are easily misapplied and the (DCLHb) in patients suffering from severe trau- conclusions drawn from their use may be inappro- matic hemorrhagic shock.11 (For this study, the ef- priate. An intuitive, easily understood summary fect of treatmentÐoverall, within subgroups, and measure is worthwhile only to the extent that it re- sults in valid conclusions. From the Department of Biostatistics and Medical Informatics, Arguments in the second category appear to be University of Wisconsin, Madison, WI (TDC). Received October 8, 2001; revision received May 7, 2002; ac- based implicitly on two assumptions. The ®rst is cepted July 17, 2002. that the most appropriate summary of differences Series editor: Roger J. Lewis, MD, PhD, Department of Emer- between groups is the RR and that this measure gency Medicine, Harbor±UCLA Medical Center, Torrance, CA. should be reported whenever possible. Second, Address for correspondence and reprints: Thomas D. Cook, PhD, 209 WARF Building, 610 Walnut Street, Madison, WI since ORs, especially when the underlying risk is 53705. Fax: 608-263-0415; e-mail: [email protected]. high, are more extreme than RRs (larger than RR ACAD EMERG MED · December 2002, Vol. 9, No. 12 · www.aemj.org 1431 TABLE 1. Mortality in the Diaspirin Cross-linked Hemoglobin (DCLHb) Study11 Overall and by Baseline Predicted Probability of Death Using the TRISS Method TRISS-predicted Probability of Survival Overall 80%±100% 20%±80% 0%±20% DCLHb Control DCLHb Control DCLHb Control DCLHb Control Dead 24 8 5 1 5 1 12 6 (Mortality) (46.2%) (17.4%) (21.7%) (4.5%) (38.5%) (8.3%) (92.3%) (60.0%) Alive 28 38 18 21 8 11 1 4 Total5246232213121310 Note that ®ve patients had insuf®cient baseline data upon which to compute a TRISS score. when RR > 1 and smaller than RR when RR < 1), the average risk does not necessarily represent the and they overstate the differences between treat- risk for any particular individual, the RR calculated ment groups. Again, a case can be made that pre- using the average risk may not represent the RR for cisely the opposite is true: when they differ, RRs any particular individual. Assuming that the two actually understate treatment differences. groups are balanced with respect to underlying pa- The purpose of this article is to argue that in tient risk (no confounding), the aggregate unad- many cases the OR is a more appropriate summary justed RR will apply to individuals only if there is measure that can be applied to a broader popula- a common RR over the population (homogeneous tion of patients than the RR. In such cases ORs RR assumption). Understanding this fact is critical should be preferred, especially when ORs and RRs to the correct application of RRs in practice. Con- differ, i.e., when outcomes are common. Notwith- sidering Table 2, the overall observed RR of 2.65 standing the errors in the interpretation of the re- likely does not represent the RR for any of the sub- sults reported by Schulman et. al.,2 there is no evi- groups, especially the high-risk group (it is outside dence that in practice, errors resulting from the the 95% con®dence interval for the RR for this misinterpretation of ORs are more frequent than er- group). It is also well below the observed RR in the rors resulting from the misinterpretation of RRs. We other two groups (although well within the corre- suggest, and illustrate below, that practitioners who sponding con®dence intervals). These differences are likely to misinterpret or misuse ORs are also suggest that the homogeneity of RR assumption likely to misinterpret or misuse RRs. does not hold (This example is primarily for pur- poses of illustration and no attempt at statistical RISK RATIOS VERSUS ODDS RATIOS inference is intended. Because of the relatively small numbers of patients in this study, observed Given an outcome of interest (considered a failure), differences among groups, here and in what fol- the risk of failure is the probability that a patient lows, may not reach statistical signi®cance. This will experience failure. For a given population, the fact should have no bearing on the principles being risk is usually estimated by the proportion of the illustrated). population observed to fail. It is important to keep in mind, however, that there is likely to be variation TABLE 2. Risk Ratios (RRs) and Odds Ratios (ORs) in risk within the population. The observed popu- in the Diaspirin Cross-linked Hemoglobin 11 lation risk is actually an average of the risks for the (DCLHb) Study Overall and by Baseline individuals in the population, and therefore, the av- Predicted Probability of Death Using the erage risk may not necessarily apply to individuals TRISS Method within the population. Again, this can be illustrated TRISS-predicted by considering data from the DCLHb study shown Probability of in Table 1. Three subpopulations are de®ned by the Survival RR 95% CI OR 95% CI probability of survival using the TRISS method.12 Overall 2.65 (1.32, 5.32) 4.07 (1.59, 10.4) We consider a low-risk group (45 patients), a mid- 80%±100% 4.78 (0.61, 37.7) 5.83 (0.62, 54.7) dle-risk group (25 patients), and a high-risk group 20%±80% 4.62 (0.63, 34.1) 6.88 (0.67, 70.8) (23 patients). Note that ®ve patients had insuf®cient 0%±20% 1.54 (0.91, 2.61) 8.00 (0.73, 88.2) baseline data to compute the TRISS score. Now, given two groups of patients, for example, TRISS-adjusted 2.07 (1.22, 4.50) 7.15 (2.18, 23.5) treated and control, the (unadjusted) RR is the ratio For the TRISS-adjusted RR, the con®dence interval (CI) was of the risks in the two groups. As above, because computed using a bootstrap method. 1432 Cook · UP WITH ODDS RATIOS! Conversely, the odds of failure is the ratio of the For these patients it is nonsensical to suggest that failure probability to the success probability. In a they would have risk of more than 100% (38% ϫ population, the odds can usually be estimated by 2.65 = 101%) if given DCLHb.
Details
-
File Typepdf
-
Upload Time-
-
Content LanguagesEnglish
-
Upload UserAnonymous/Not logged-in
-
File Pages5 Page
-
File Size-