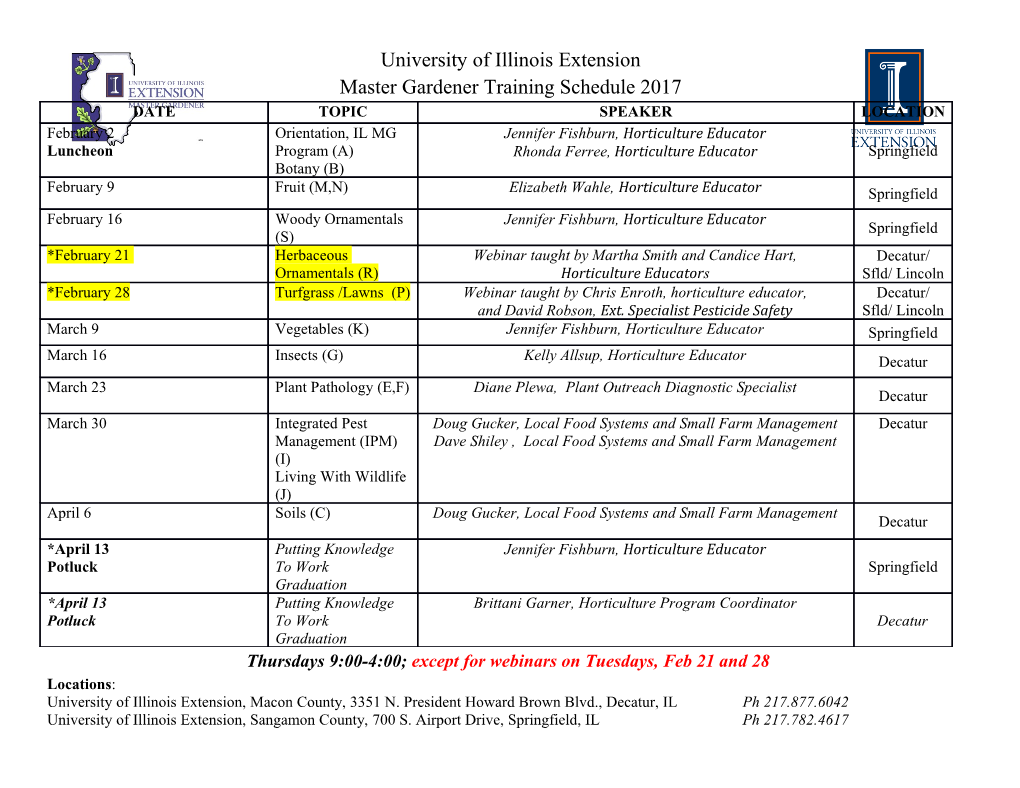
Hydrol. Earth Syst. Sci. Discuss., https://doi.org/10.5194/hess-2017-322 Manuscript under review for journal Hydrol. Earth Syst. Sci. Discussion started: 7 June 2017 c Author(s) 2017. CC BY 3.0 License. How can expert knowledge increase the realism of conceptual hydrological models? A case study in the Swiss Pre-Alps Manuel Antonetti1,2, Massimiliano Zappa1 1 Swiss Federal Research Institute WSL, Birmensdorf, 8903, Switzerland 5 2 Department of Geography, University of Zurich, Zurich, 8057, Switzerland Correspondence to: Manuel Antonetti ([email protected]) Abstract. Both modellers and experimentalists agree that using expert knowledge can improve the realism of conceptual hydrological models. However, their use of expert knowledge differs for each step in the modelling procedure, which involves hydrologically mapping the dominant runoff processes (DRPs) occurring on a given catchment, parameterising 10 these processes within a model, and allocating its parameters. Modellers generally use very simplified mapping approaches, applying their knowledge in constraining the model by defining parameter and process relational rules. In contrast, experimentalists usually prefer to invest all their detailed and qualitative knowledge about processes in obtaining as realistic spatial distribution of DRPs as possible, and in defining narrow value ranges for each model parameter. Runoff simulations are affected by equifinality and numerous other uncertainty sources, which challenge the assumption that 15 the more expert knowledge is used, the better will be the results obtained. To test to which extent expert knowledge can improve simulation results under uncertainty, we therefore applied a total of 60 modelling chain combinations forced by five rainfall datasets of increasing accuracy to four nested catchments in the Swiss Pre-Alps. These datasets include hourly precipitation data from automatic stations interpolated with Thiessen polygons and with the Inverse Distance Weighting (IDW) method, as well as different spatial aggregations of Combiprecip, a combination between ground measurements and 20 radar quantitative estimations of precipitation. To map the spatial distribution of the DRPs, three mapping approaches with different levels of involvement of expert knowledge were used to derive so-called process maps. Finally, both a typical modellers’ top-down setup relying on parameter and process constraints, and an experimentalists’ setup based on bottom-up thinking and on field expertise were implemented using a newly developed process-based runoff generation module (RGM- PRO). To quantify the uncertainty originating from forcing data, process maps, model parameterisation, and parameter 25 allocation strategy, an analysis of variance (ANOVA) was performed. The simulation results showed that: (i) the modelling chains based on the most complex process maps performed slightly better than those based on less expert knowledge; (ii) the bottom-up setup performed better than the top-down one when simulating short-duration events, but similarly to the top-down setup when simulating long-duration events; (iii) the differences in performance arising from the different forcing data were due to compensation effects; and (iv) the bottom-up 30 setup can help identify uncertainty sources, but is prone to overconfidence problems, whereas the top-down setup seems to accommodate uncertainties in the input data best. Overall, modellers’ and experimentalists’ concept of “model realism” 1 Hydrol. Earth Syst. Sci. Discuss., https://doi.org/10.5194/hess-2017-322 Manuscript under review for journal Hydrol. Earth Syst. Sci. Discussion started: 7 June 2017 c Author(s) 2017. CC BY 3.0 License. differ. This means that the level of detail a model should have to accurately reproduce the DRPs expected must be agreed in advance. 1 Introduction Applying expert knowledge in hydrology, as in any other natural science, is crucial for linking observations and laws 5 governing a given system, such as a catchment. It usually involves formulating and testing hypotheses about how the system functions (Savenije, 2009). At the root of this scientific reasoning, two opposing philosophies can be identified: the top- down and the bottom-up approaches. The first can be traced back to the Greek philosopher Plato (428 – 348 BC), who was trying to link general theories about the functioning of complex systems to measurable observations. A “bottom-up” approach involves extrapolating general theories from given observations, and can be attributed to Plato’s student Aristotle 10 (384 – 322 BC). These two approaches have been applied in nearly all scientific disciplines, e.g. in mathematics (Cellucci, 2013), economics (Böhringer and Rutherford, 2008) and neuroscience (Gilbert and Li, 2013), as well as hydrology. Thus one type of hydrological scientist, the experimentalist or “wet” hydrologist, tries to understand catchment functioning through extended field investigations, whereas the modeller or “dry” hydrologist tends to develop theories at the catchment scale and successively tries to validate them against measurements (Seibert and McDonnell, 2002). 15 Both modellers and experimentalists agree on the importance of expert knowledge for improving the realism of hydrological models, e.g. by forcing the model to reproduce the processes observed in the catchment. In recent years, several process- oriented approaches have been developed, of which the concept of dominant runoff process (DRP, for list of abbreviations see Table A1) is one (Blöschl, 2001). It relies on the hypothesis that, among the different runoff generation mechanisms that may occur at a given location (Hortonian overland flow HOF, saturation overland flow SOF, subsurface flow SSF, and deep 20 percolation DP), one, the DRP, will be dominant over the others. Based on this concept, the following process-based modelling chain has been proposed (Clark et al., 2015): (i) reading the landscape, identifying and classifying the processes, (ii) developing a proper parameterisation to reflect our perceptions of the processes observed and (iii) allocating the parameter values of these parameterisations (Fig. 1). Wet and dry hydrologists disagree, however, on how to implement their expert knowledge in each of these steps. For 25 example, Schmocker-Fackel et al. (2007) applied the two philosophies to hydrological classifications using DRPs and claimed: “[…] These top-down approaches try to identify homogeneous landscape units. The assumption is that the hydrological response will also be homogeneous. By contrast, in bottom-up approaches, runoff formation is investigated on the plot scale and then units with the same runoff forming process are identified” (Schmocker-Fackel et al., 2007). Examples of such bottom-up mapping approaches can be found in Markart et al. (2004); Smoorenburg (2015), Scherrer AG (2006), 30 Scherrer and Naef (2003) and Tilch et al. (2006), and of top-down mapping approaches in Gao et al. (2014), Gharari et al. (2011) and Fenicia et al. (2016). 2 Hydrol. Earth Syst. Sci. Discuss., https://doi.org/10.5194/hess-2017-322 Manuscript under review for journal Hydrol. Earth Syst. Sci. Discussion started: 7 June 2017 c Author(s) 2017. CC BY 3.0 License. Different interpretations of the two philosophies have been applied in hydrological modelling. For example, Hrachowitz and Clark (2017) maintain bottom-up models correspond to physically-based models, where the conservations laws on mass, momentum and energy are solved. In contrast, top-down models are conceptual models. With regard to the level of modelling detail, Nalbantis et al. (2011) linked monometric approaches, where some components are examined in detail and 5 other ones are only roughly described, to the bottom-up philosophy and the holistic approach, when all components are modelled with the same degree of detail, to the top-down one. Sivapalan et al. (2003), in contrast, classify approaches according to the scale considered: if the modelling is performed first at the small scale of e.g. HRU, or hillslopes, and then the results are scaled up to the catchment scale, it can be defined as bottom-up, whereas lumped models developed directly at the catchment scale can be defined as top-down. The definition of Sivalapan et al. (2003) also works well with the concepts 10 of model parameterisation and parameter allocation. For example, in a classical bottom-up exercise, parameter ranges are narrowed and/or model parameterisations are proposed based on catchments properties, expert knowledge and possibly inferences from measurements. By following a top-down approach, expert knowledge can be used instead to define relational rules between the parameters and fluxes of different landscape classes. In this way, the model is forced to behave according to the modeller’s perception of the catchment functioning and the parameter space can be reduced so that no 15 calibration is necessary (Bahremand, 2016; Gharari et al., 2014). Both approaches have strengths and weaknesses when implementing expert knowledge in process-based hydrological modelling. Bottom-up mapping approaches are often considered to require much data (Hümann and Müller, 2013; Müller et al., 2009), whereas top-down classification approaches are considered too coarse to detect the spatial distribution of processes with enough accuracy (Antonetti et al., 2016). Top-down models and parameterisations may be too simplistic and, 20 therefore, require calibration (e.g. Fatichi et al., 2016), whereas physically-based
Details
-
File Typepdf
-
Upload Time-
-
Content LanguagesEnglish
-
Upload UserAnonymous/Not logged-in
-
File Pages42 Page
-
File Size-