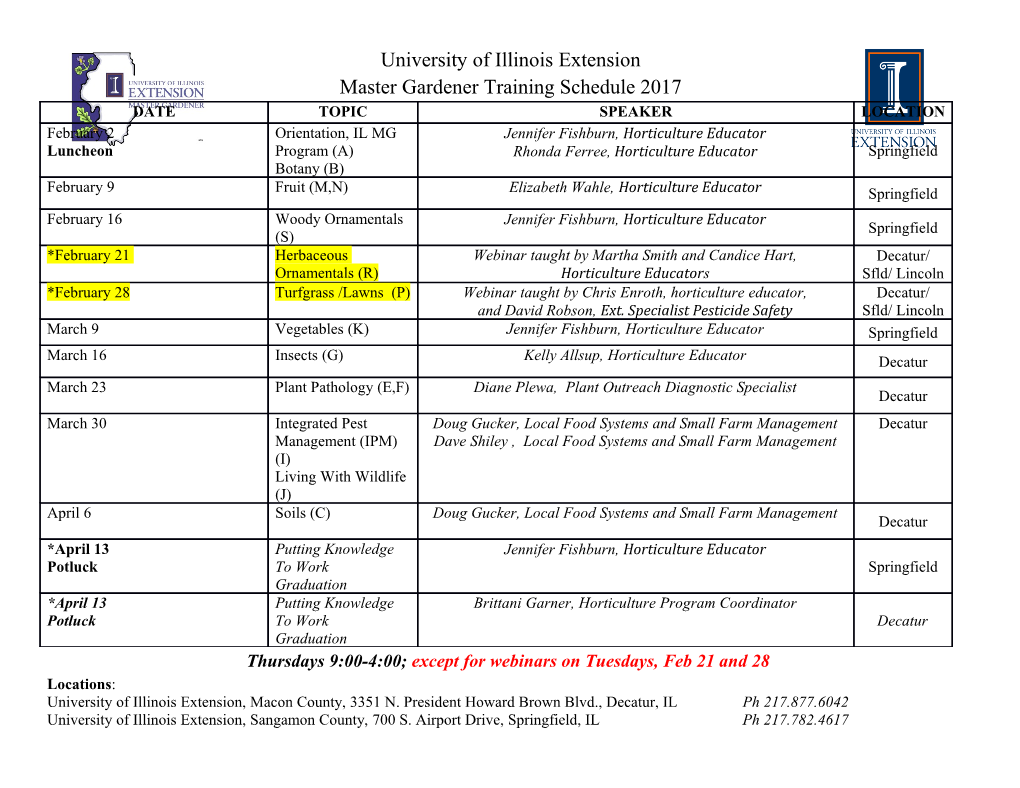
Cancer Genome and Epigenome Research Sex Differences in Cancer Driver Genes and Biomarkers Constance H. Li1,2, Syed Haider1, Yu-Jia Shiah1,2, Kevin Thai1, and Paul C. Boutros1,2,3 Abstract Cancer differs significantly between men and women; even and BAP1. Sex influenced biomarkers of patient outcome, after adjusting for known epidemiologic risk factors, the sexes where different genes were associated with tumor aggression differ in incidence, outcome, and response to therapy. These in each sex. These data call for increased study and consider- differences occur in many but not all tumor types, and their ation of the molecular role of sex in cancer etiology, progres- origins remain largely unknown. Here, we compare somatic sion, treatment, and personalized therapy. mutation profiles between tumors arising in men and in women. We discovered large differences in mutation density Significance: This study provides a comprehensive cata- and sex biases in the frequency of mutation of specific genes; log of sex differences in somatic alterations, including in these differences may be associated with sex biases in DNA cancer driver genes, which influence prognostic biomarkers mismatch repair genes or microsatellite instability. Sex-biased that predict patient outcome after definitive local therapy. genes include well-known drivers of cancer such as b-catenin Cancer Res; 78(19); 5527–37. Ó2018 AACR. Introduction advantage declines and disappears during menopause (11). Some of these differences in treatment response may be attributed to Sex differences in cancer have been known at least since 1949 differences in driver mutations between the sexes, and others to (1), with repeated demonstration that males have higher cancer differences in epigenetics or chromatin conformation. risk both in studies using North American (e.g., SEER; ref. 2) and The origins and mechanisms of these sex differences remain a international databases (e.g., IARC; ref. 3). Most, but not all tumor majorunresolvedquestionincancerbiology.Theymaybe types show increased incidence in men: thyroid cancer occurs caused by differences in the expression of genes on the sex 2.5 times more frequently in women. These differences remain chromosomes, in hormone levels, in developmental biology, after controlling for known epidemiologic risk factors (3). At most or in lifestyle features not reflected in current epidemiologic tumor sites, cancers arising in men induce higher mortality (4); for studies. Likely, a mixture of all these components contributes to example, there is a 3-fold increase in lethality from urinary sex differences in patient outcomes. We hypothesized that, bladder carcinomas in men relative to women (4). Further, there independent of their mechanism, sex differences in cancer are significant differences in response to treatment: female would be reflected by differences in somatic mutation profiles. patients with non–small cell lung cancer respond better to both That is, male and female tumors would acquire mutations at surgery (5, 6) and chemotherapy (7, 8), even after accounting for different rates and of different types. Recent intriguing data differences in variables such as subtype. Female patients with on missense mutations in melanoma support this hypothesis colorectal cancer respond better to surgery, and this difference is (12). We, therefore, undertook a systematic evaluation of sex- driven by improved female survival in the rectal cancer subgroup associated biases in mutations in cancer across a broad range (9). Similarly, female patients with colorectal also respond better of tumor types. Our study provides a comprehensive pan- to chemotherapy, which is partially attributed to differences in cancer catalog of sex-biased mutations and a perspective on tumor site and microsatellite instability (10). Finally, a propen- sex-specific prognostic biomarkers. sity-matched study of nasopharyngeal carcinoma found that females have a survival advantage regardless of tumor stage, Materials and Methods radiation technique, and chemotherapy regimen, but that this Data acquisition and processing mRNA abundance, DNA genome-wide somatic copy-number 1Computational Biology Program, Ontario Institute for Cancer Research, Tor- and somatic mutation profiles for the Cancer Genome Atlas 2 onto, Ontario, Canada. Department of Medical Biophysics, University of Tor- (TCGA) datasets were downloaded from Broad GDAC 3 onto, Toronto, Ontario, Canada. Department of Pharmacology and Toxicology, Firehose (https://gdac.broadinstitute.org/), release 2016-01-28. University of Toronto, Toronto, Ontario, Canada. For mRNA abundance, Illumina HiSeq rnaseqv2 level 3 RSEM- Note: Supplementary data for this article are available at Cancer Research normalized profiles were used. Genes with >75% of samples Online (http://cancerres.aacrjournals.org/). having zero reads were removed from the respective data set. Corresponding Author: Paul C. Boutros, Ontario Institute for Cancer Research, GISTIC v2 (13) level 4 data were used for somatic copy-number Toronto, ON M5G0A3, Canada. Phone: 647-258-4321; E-mail: analysis. mRNA abundance data were converted to log2 scale for [email protected] subsequent analyses. Mutational profiles were based on TCGA- doi: 10.1158/0008-5472.CAN-18-0362 reported MutSig v2.0 calls. All preprocessing was performed in R Ó2018 American Association for Cancer Research. statistical environment (v3.1.3). www.aacrjournals.org 5527 Downloaded from cancerres.aacrjournals.org on September 24, 2021. © 2018 American Association for Cancer Research. Li et al. Patients younger than 18, older than 85 or lacking sex anno- used to reduce false positives that may arise from unbalanced tation were excluded from analysis, resulting in a sample size of tumor type subsets of the pan-cancer data. Multivariate logistic 7,131 across all tumor types for copy-number alterations (CNA; regression (MLR) was used to adjust ternary CNA data for sex, age, 1.5% excluded, Supplementary Table S1) and 6,073 for somatic race, and tumor type. The MLR sex term was tested for significance single-nucleotide variants (SNV; 1.5% excluded; Supplementary and FDR corrected to identify bins with pan-cancer sex biases (q < Table S1). Genes were excluded if they were mutated in fewer than 0.05). 20 patients (for CNAs) or 5% of patients (for SNVs). Gene filters The same approach was applied to each tumor type individ- were applied independently for pan-cancer and per individual ually. Proportions tests were used to select bins for multivariate tumor type data set. All analyses excluded genes on the X and Y analysis (q value < 0.1). MLR was again used to adjust ternary chromosomes. copy-number call for clinical variables. MLR modeling for each tumor type varies based on available clinical data. Tumor type– Mutation load specific models were fit independently per univariately significant Mutation load per patient was calculated as the sum of SNVs bin and variable significance for each bin was extracted from the across all genes on the autosomes. Mutation load was Box–Cox fitted models. FDR correction was used and an FDR threshold of transformed, and transformed values were compared between the 0.05 was used. A description of pan-cancer and tumor type– sexes using unpaired two-sided t tests for both pan-cancer and specific models, along with a breakdown of the data for each tumor type–specific analysis. A linear regression model was used group, can be found in Supplementary Table S1 and results can be to adjust mutation load for tumor type for the pan-cancer com- found in Supplementary Tables S3–S5. parison. Tumor type–specific P values were adjusted using the Benjamini–Hochberg false discovery rate procedure. Tumor types CNA-mRNA functional analysis with q values meeting an FDR threshold of 10% were further Genes in bins altered by sex-biased CNAs after multivariate analyzed using linear regression to adjust for tumor type–specific adjustment for kidney clear cell and kidney papillary cell cancers variables described in Supplementary Table S1. A multivariate were further investigated to determine sex-biased functional q value threshold of 0.05 was then used to determine statistical effects. Available mRNA samples were matched to those used in significance. Full results are in Supplementary Table S2. CNA analysis. For each gene affected by a sex-biased loss, its mRNA abundance was modeled against sex, copy-number loss Genome instability status, and a sex–copy-number loss interaction term. The inter- Genome instability was calculated as the percentage of the action term was used to identify genes with sex-biased mRNA genome affected by copy-number alterations. The number of base changes. FDR-adjusted P values and fold changes were extracted pairs for each CNA segment was summed to obtain a total number for visualization. A q value threshold of 0.05 was used for of base pairs altered per patient. The total number of base pairs statistical significance. For genes affected by sex-biased gains, the was divided by the number of assayed bases excluding the sex same procedure was applied using copy-number gains. chromosomes (7.8 million bp) to obtain the percentage of the genome altered (PGA). Box–Cox transformed PGA was treated as CNA-mRNA survival analysis a continuous variable and compared by sex using two-sided Genes found to have significant or trending (FDR threshold of unpaired t tests for all tumor types combined (pan-cancer) and 10%) sex biases in the CNA-mRNA functional analysis were separately (tumor type–specific). Linear regression models were further analyzed using Cox proportional hazards modeling. That used to adjust PGA for tumor type, age, and race for the pan-cancer is, we focused on genes that were both altered by sex-biased CNAs comparison. Tumor types where univariate testing indicated (MLR q value < 0.05) and showed mRNA abundance differences putative sex biases in PGA (FDR threshold of 10%) were also between the copy-number neutral and loss/gain groups for either adjusted for tumor type–specific variables (Supplementary sex (sex–loss interaction q < 0.1). For each gene, the mRNA Table S1).
Details
-
File Typepdf
-
Upload Time-
-
Content LanguagesEnglish
-
Upload UserAnonymous/Not logged-in
-
File Pages12 Page
-
File Size-