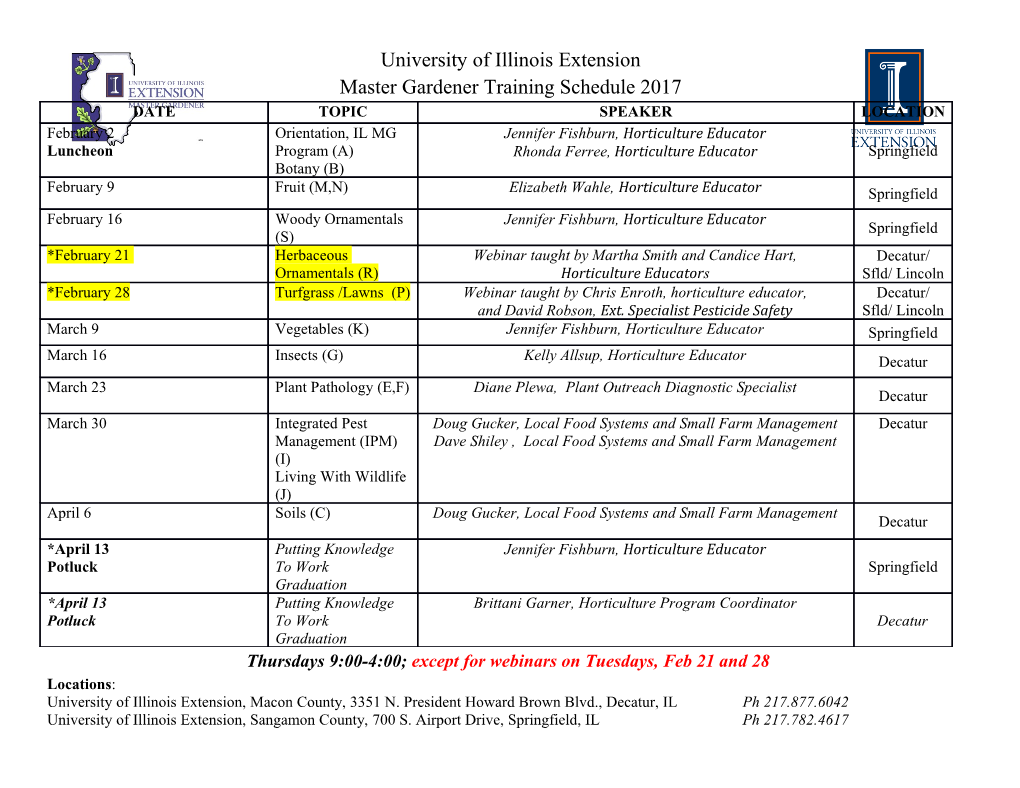
Design under uncertainty E. Nikolaidis Aerospace and Ocean Engineering Department Virginia Tech Acknowledgments Sophie Chen (VT) Harley Cudney (VT) Raphael Haftka (UF) George Hazelrigg (NSF) Raluca Rosca (UF) Outline • Decision making problem • Why we should consider uncertainty in design • Available methods • Objectives and scope • Comparison of probabilistic and fuzzy set methods • Concluding remarks 1. Decision making problem Noise Design 1 level (db) Design 2 Design 3 Initial target Cost ($) Which design is better ? Taxonomy of decision problems (Keney and Raiffa, 1994) Certainty about Uncertainty about outcomes of actions outcomes of actions ONE Type I problems Type II problems ATTRIBUTE IS Approach: Approaches: Utility SUFFICIENT Deterministic theory, fuzzy set FOR optimization theory DESCRIBING AN OUTCOME MULTIPLE Type III problems Type IV problems ATTRIBUTES Approaches: Utility Approaches: Utility ARE NEEDED theory, fuzzy set theory, fuzzy set FOR theory theory DESCRIBING AN OUTCOME Types of uncertainty Irreducible: due to inherent Reducible: due to use Statistical: randomness of imperfect models due to lack in physical to predict of data for phenomena and outcomes of an action processes modeling uncertainty Preferences • An outcome is usually described with one or more attributes • Preferences are defined imprecisely: no clear sharp boundary between success and failure • Need a rational approach to quantify value of an outcome to decision maker – Utility theory – Fuzzy sets 2. Why we should consider uncertainty in design • Design parameters are uncertain -- there is no way to make a perfectly safe design • Ignoring uncertainty and using safety factors usually leads to designs with inconsistent reliability levels 3. Available methods • Safety factor • Worst case scenario-convex models • Taguchi methods • Fuzzy set methods • Probabilistic methods Probabilistic methods • Approach – Model uncertainties using PDF’s – Estimate failure probability – Minimize probability of failure and/or cost • Advantage: account explicitly for probability of failure • Limitations: – Insufficient data – Sensitive to modeling errors (Ben Haim et al., 1990) Fuzzy set based methods • Possibility distributions • Possibility of event = 1-degree of surprise (Shackle, 1969) • Relation to fuzzy sets (Zadeh, 1978): X is about 10: Possibility distribution 1 0.25 Probability distribution 8 10 12 Fuzzy sets in structural design • Uncertainty in mechanical vibration: Chiang et al., 1987, Hasselman et al., 1994 • Vagueness in definition of failure of reinforced plates (Ayyub and Lai, 1992) • Uncertainty and imprecision in preferences in machine design (Wood and Antonsson, 1990) • Relative merits of probabilistic methods and fuzzy sets may depend on: – Amount and type of available information about uncertainty – Type of failure (crisp or vague) – Accuracy of predictive models Important issues • Are fuzzy sets better than probabilities in modeling random uncertainty when little information is available? • How much information is little enough to switch from probabilities to fuzzy sets? • Compare experimentally fuzzy set and probabilistic designs 4. Objectives and scope • Objectives: – Compare theoretical foundations of probabilistic and fuzzy set methods – Demonstrate differences on example problems – Issue guidelines -- amount of information • Scope: – Problems involving uncertainty – Problems involving catastrophic failure Æ clear, sharp boundary between success and failure 5. Comparison of probabilistic and fuzzy set methods • Comparison of theoretical foundations – Axiomatic definitions – Probabilistic and possibility-based models of uncertainty – Risk assessment – Design for maximum safety • Comparison using a design problem Axiomatic definitions Probability measure, P(⋅) Possibility measure, Π(⋅) 1) P(A) ≥ 0 ∀ A∈S 1) Boundary requirements: Π(∅)=0, Π(Ω)=1 2) Boundary requirement: 2) Monotonicity: P(Ω)=1 ∀A, B ∈ S, if A ⊆ B, then Π(A) ≤ Π(B) 3) Probability of union of 3) Possibility of union of a events finite number of events ∀ ∈ Ai , i I, Ai are disjoint ∀Ai , i∈ I, Ai disjoint I I Π = Π P( U Ai ) = ∑ P(Ai ) ( U Ai ) maxi∈I ( (Ai )) i=1 i∈I i=1 Differences in axioms • Probability measure can be assigned to the members of a s-algebra. Possibility can be assigned to any class of sets. • Probability measure is additive with respect to the union of sets. Possibility is subadditive. P(A) + P(AC ) =1 Π(A) + Π(AC ) ≥1 Probability density and possibility distribution functions fX(x) ≠ 1 Area=1 x0 x P(X=x0)=0 ΠX(x) Area≥1 1 x x0 Π(X=x0)≠0 Modeling an uncertain variable when very little information is available Maximum uncertainty principle: use model that maximizes uncertainty and is consistent with data Possibility distribution 1 Probability distribution 0.25 8 10 12 8.5 • Increase range of variation from [8,12] to [7,13]: – Failure probability: 0.13Æ0.08 – Failure possibility: 0.50 Æ0.67 • Design modification that shifts failure zone from [8,8.5] to [7.5,8] – failure probability: 0.13 Æ0 (if range of variation is [8,12]) – failure probability remains 0.08 (if range of variations is [7,13]) • Easy to determine most conservative possibility based model consistent with data • Do not know what modeling assumptions will make a probabilistic model more conservative • Probabilistic models may fail to predict effect of design modifications on safety • The above differences are due to the difference in the axioms about union of events Risk assessment: Independence of uncertain variables • Assuming that uncertain parameters are independent always makes a possibility model more conservative. This is not the case with probabilistic models 2 P, P PFS=P if independent PFS=P if perfectly correlated P, P PFS= P in both cases where components are independent or correlated A paradox Probability-possibility consistency: The possibility of any event should always be greater or equal to its probability P, P ... PFS=1-(1-P)n PFS=P 1 System failure probability P System failure possibility P 1 Number of components To ensure that failure possibility remains equal or greater than failure possibility need to impose the condition: ∀A: P(A) f 0, Π(A) =1 Design for maximum safety • Probabilistic design : • Possibility-based – find d1,…, dn design: – to minimize PFS – find d1,…, dn – so that g0 – to minimize PFS – so that g0 Two failure modes: PFS=PF1+PF2-PF12 PFS=max(PF1, PF2) Optimality conditions Assume PF12 small P PF1 P PF1 PF2 PF2 d 0 d d0 d ∂ ∂ PF1 = − PF2 PF1=PF2 ∂ d ∂ d Comparison using a design problem • No imprecision in defining failure • Only random uncertainties • Only numerical data is available about uncertainties How to evaluate methods: Average probability of failure General approach for comparison Information about uncertainties Incomplete Budget information Optimization: Maximize Safety Fuzzy Design Probabilistic Design Probabilistic Analysis Probabilistic Analysis Compare relative frequencies of failure m, wn2 absorber Normalized M, wn1 original system system amplitude y F=cos(wet) Figure 2. Tuned damper system Original SDOF system Failure modes 1. Excessive vibration 2. Cost > budget (cost proportional to m) 60 48 b normalized natural 36 frequencies System amplitude 24 (assumed equal) 12 0 0.8 0.84 0.88 0.92 0.96 1 1.04 1.08 1.12 1.16 1.2 β :R=0.05; : R=0.01 Figure 4. Amplitude of system vs. β, ζ=0.01 Uncertainties 1 Normalized frequencies 2 Budget • Know true probability distribution of budget • Know type of probability distribution of normalized frequencies and their mean values, but not their scatter • Samples of values of normalized frequencies are available Design problem • Find m • to minimize PF (PF) • PF=P(excessive vibration¯cost overrun) • ½F=P(excessive vibration ¯cost overrun) • heavy absorber, low vibration but high cost Estimation of variance of b Concept of maximum uncertainty: if little information is available, assume model with largest uncertainty that is consistent with the data Comparison of ten probabilistic and ten possibility-based designs. Three sample values were used to construct models of uncertainties. Blue bars indicate possibility-based designs. Red bars indicate probabilistic designs. - Inflation factor method, unbiased estimation 0.35 0.3 0.25 0.2 0.15 0.1 Actual probability of failure 0.05 0 12345678910 Data group sample size equal to 3,000 0.25 0.2 0.15 0.1 Actual probability of failure of probability Actual 0.05 0 12345678910 Data group Probabilistic approach cannot predict design trends 0.3 0.2 0.1 to excessive vibration excessive to Failureprobability due 0 0 0.01 0.02 0.03 0.04 0.05 0.06 0.07 R distribution of frequency - U(1,0.05) distribution of frequency - U(1,0.075) distribution of frequency - U(1,0.1) Figure 5. Effect of standard deviations of b1 and b2 on the probability of failure vs. R , b1 and b2 are equal Comparison in terms of average failure probability as a function of amount of information Sample size Best design 3 5 10 20 100 1000 Blue bullet: on average possibility is better, red bullet: on average probability is better Concluding remarks • Overview of problems and methods for design under uncertainty • Probabilistic and fuzzy set methods -- comparison of theoretical foundations • Probabilistic and fuzzy set methods -- comparison using design problem Concluding remarks • Important to consider uncertainties • There is no method that is best for all problems involving uncertainties • Probabilistic design better if sufficient data is available • Possibility can be better if little information is available – easier to construct most conservative model consistent with data – probabilistic methods may fail to predict effect of design modifications • Major difference in axioms about union of events.
Details
-
File Typepdf
-
Upload Time-
-
Content LanguagesEnglish
-
Upload UserAnonymous/Not logged-in
-
File Pages41 Page
-
File Size-