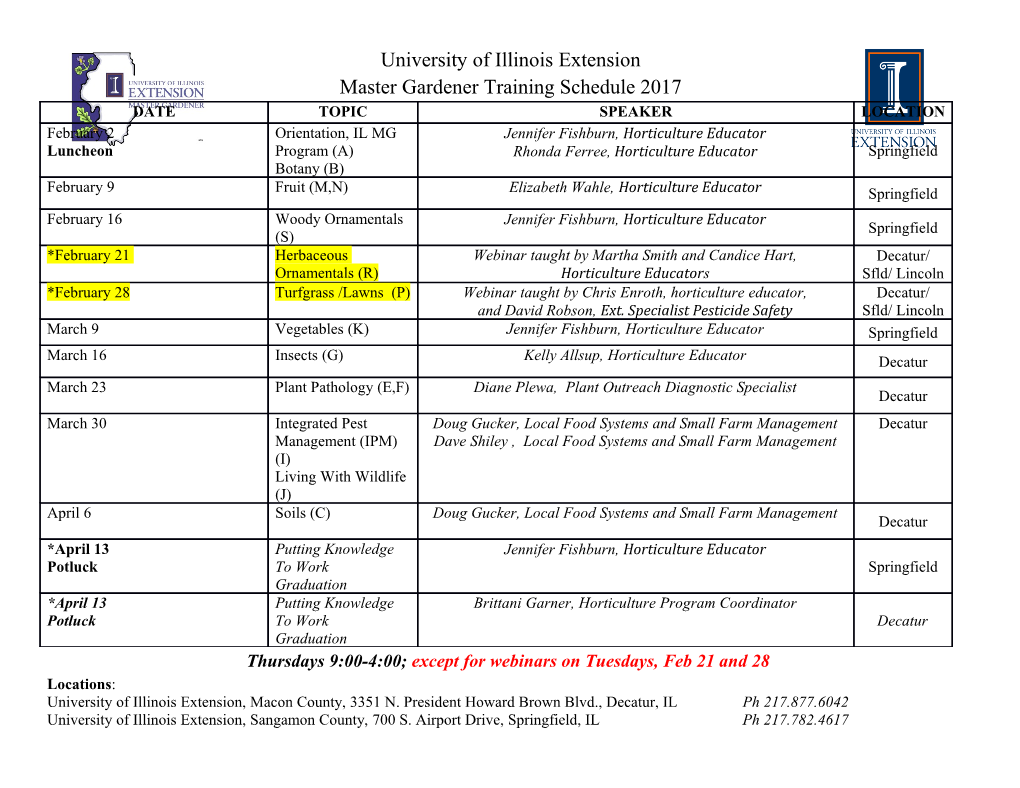
Bivariate Normal Distribution X and Y are bivariate normal if: X = µX + aZ1 Y = µY + bZ1 + cZ2 2 2 Parameters are µX ; µY ; σX ; σY ; σXY If σXY = 0, then X and Y are independent. Note: This is special for bivariate normal. Most often used: XjY has a normal distribution with ρσX E [XjY ] = µX + (Y − µY ) σY 2 2 Var (XjY ) = σX 1 − ρ Cov(X; Y ) Recall: The Correlation Coefficient is ρXY = ρ = σX σY The Bivariate Normal Distribution looks pretty complicated. Honestly, we hardly ever use the first definition. More often than not, we need to know two things: • XjY and Y jX are normally distributed, with mean and variance according to the formulas above. • A linear combination of X and Y is also normal, with mean E[aX +bY ] = aE[X]+bE[Y ] and variance Var(aX + bY ) = a2Var(X) + b2Var(Y ) + 2abCov(X; Y ). An attempt to explain the conditional expectation formula: Bivariate normal RVs have some correlation coefficient ρ which measures their tendency to vary with one another. The conditional expectation formula looks similar to a linear approximation formula. • It says the mean of X is a good place to start if you're going to adjust that mean based on Y . ρσ • The term X is a measurement of how much X changes as a result of a change in Y . σY • Lastly, Y − µY is the amount Y has shifted from it's mean. Multiplying the latter terms together gives us a measure of the change in X, and that is added to our original µX to give us a new expected value. The Conditional Variance formula can be broken down similarly. The important thing to note is that while X and Y are both \free," the variance in each is greater. Once you have been given information about Y , the variance in X shrinks, since X and Y have some relationship. Of course, if the variables are independent, the ρ is zero, so there is no change in the variance of X. 1 Example: Let X and Y have a bivariate normal distribution with means µX = 8 and µY = 7, standard deviations σX = 4 and σY = 3, and covariance σXY = 2. Let Φ denote the cumulative distribution function of a normal random variable with mean 0 and variance 1. Determine P (3X − 2Y ≤ 9) in terms of Φ. 2 2 Let X and Y have a bivariate normal distribution with means µX = µY = 0 and variances σX = 2, σY = 3, 1 and correlation ρ = . XY 3 Find the conditional variance of Y , given X = x. 2 Example: Auto collision losses X and auto comprehensive losses Y are modeled as a bivariate normal 2 2 distribution with µX = 3000, µY = 2000, σX = 90; 000, σY = 62; 500, and ρXY = 0:4. Calculate the probability that Y is greater than 2000, given that auto collision losses are 5000. 3.
Details
-
File Typepdf
-
Upload Time-
-
Content LanguagesEnglish
-
Upload UserAnonymous/Not logged-in
-
File Pages3 Page
-
File Size-