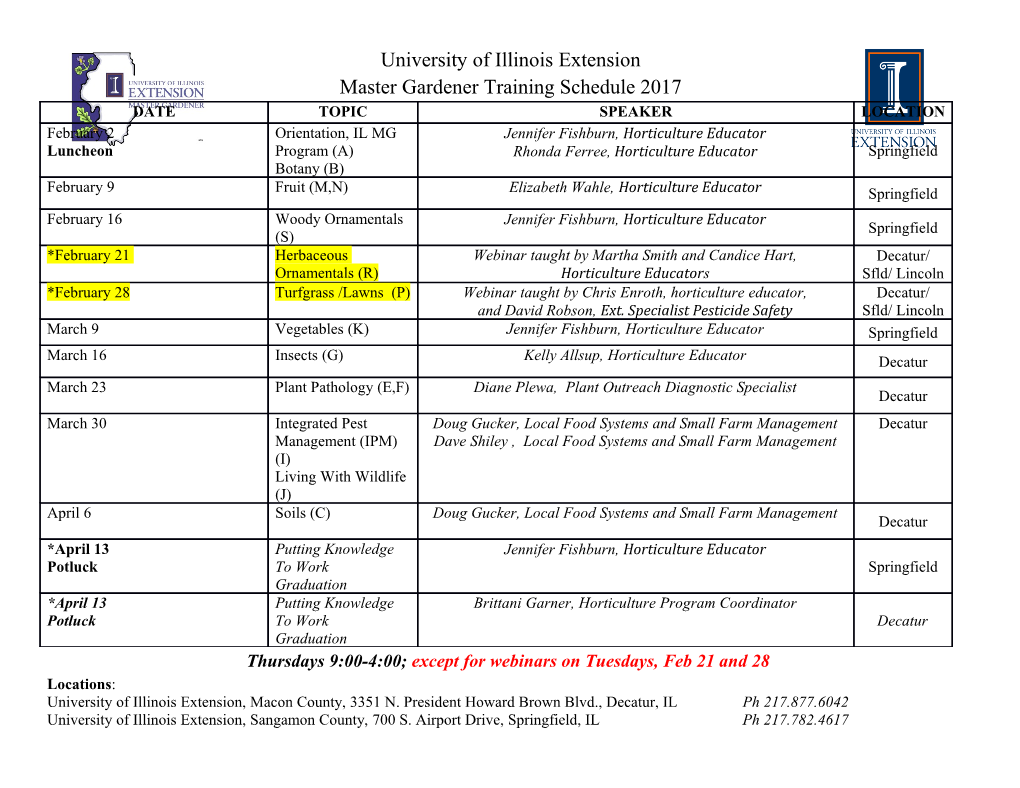
Magne Skålevik Brekke & Strand, Oslo www.akutek.info Predicting Concert Hall Preference from Physical Quantities (Parameters) UNCERTAINTIES RELATED TO SELECTION OF DATA ISRA 2013, Toronto, June 9th 2013 Concert Hall Preference . Preference? The degree to which a concert hall is preferred, statistically . Why predict? Chance of Success Scientifical or academical reasons Risk of Failure Curiosity Basis for decisions (Building Committee) RISKY BUSINESS . Null Hypothesis: Preference and Physical Quantities just covaries randomly . We should first try to predict existing halls Subjective data Objective data Beranek ranking of 58 halls: Tocc Beranek Tunocc 1 Vienna Musikverein EDT Calculated from T , V and R 2 Boston Symphony Hall occ G with Barron Revised Theory 3 Buenos Airos, Teatro Colon C R = 11m + 0.67*L 4 Berlin Konzerthaus 5 Amsterdam Concertgebouw Glate 6 Tokyo Opera City, Concert Hall G125 L Beranek geometrical data .......... Halls of rank 7 to 52 .......... W 53 Sydney Opera House, Concert Hall H/W 54 San Fransisco, Davies Hall V/S0T 55 Tel Aviv, Frederic Mann ITDG Beranek data 56 London, Barbican LF Long story, a lot to be said 57 Buffalo Kleinhans 1-IACCe Long story, a lot to be said 58 London, Royal Albert Uncertainty from size of parameter subset Let’s start, like Sabine, with good old T 1p Tocc Max 2.14 Min 1.89 r2=0.26 r=0.51 Let’s start, like Sabine, with good old T Not bad (r=0.51), but there are good halls with bad T and vice-versa. Lets ask Clarity for advice... 1p Tocc Max 2.14 Min 1.89 r2=0.26 r=0.51 Uncertainty from size of parameter subset Oops, Clarity made it worse, let’s ask G Strength instead... T 1p occ 2p Tocc C Max 2.14 Max 2.14 1.0 Min 1.89 Min 1.89 -0.7 r2=0.26 r=0.51 r2=0.18 r=0.42 Uncertainty from size of parameter subset Thanks, G Strength, but still some good halls gets 0% score T 1p occ 2p Tocc G Max 2.14 Max 2.14 5.0 Min 1.89 Min 1.89 3.2 r2=0.26 r=0.51 r2=0.36 r=0.60 Uncertainty surprises! C helped after all.. T T G C 1p occ 2p Tocc G 3p occ Max 2.14 Max 2.14 5.0 Max 2.14 5.0 1.0 Min 1.89 Min 1.89 3.2 Min 1.89 3.2 -0.7 r2=0.26 r=0.51 r2=0.36 r=0.60 r2=0.41 r=0.64 Uncertainty from size of parameter subset Uncertainty from size of parameter set 1,00 1-exp(-x/10) Best fit trend 0,90 correlation r2 0,80 0,70 0,60 0,50 r^2 0,40 0,30 0,20 0,10 0,00 0 2 4 6 8 10 12 14 16 18 Number of parameters Uncertainty from size of parameter subset -G L LF 1- T T EDT G C H/W W GL V/S T occ unocc 125 0 ITDG IACCe G rsq single- 0.00 0.01 0.30 0.06 0.26 0.10 0.14 0.13 0.00 0.06 0.26 0.22 0.23 0.01 parameter rsq-difference when removed -0.03 -0.05 -0.04 -0.05 -0.04 -0.01 -0.01 -0.04 -0.07 -0.04 -0.05 -0.05 -0.03 -0.02 from set of 14 Uncertainty from size of parameter subset -G L LF 1- T T EDT G C H/W W GL V/S T occ unocc 125 0 ITDG IACCe G rsq single- 0.00 0.01 0.30 0.06 0.26 0.10 0.14 0.13 0.00 0.06 0.26 0.22 0.23 0.01 parameter rsq-difference when removed -0.03 -0.05 -0.04 -0.05 -0.04 -0.01 -0.01 -0.04 -0.07 -0.04 -0.05 -0.05 -0.03 -0.02 from set of 14 Apologies, Clarity for jumping to conclusions. You really make a difference Uncertainty from size of concert hall subset 100% 90% 80% 70% 60% 50% 40% Correlation r^2 30% 10 halls 20% 10% 0% 0 10 20 30 40 50 Number of halls Example: 10 halls, 6 paramameters, r2=0.94 Vienna Amsterdam Cardiff Munich Gothenburg Salzburg Stuttgart Edinburg London, Festival London, Barbican Class: I II III EDT G C LF Glate G125 Upper limit 2.2 5.3 0.7 0.25 2.5 5.7 Lower limit 1.8 3.3 -1.3 0.15 0.5 3.7 53 halls, 12 parameters, r=0.86, r2=0.75 L ITDG Tocc Tunocc EDT G C G125-G H/W W GL V/S0T 53 halls, 12 parameters, r=0.86, r2=0.75 100% 90% R² = 0,75 80% 70% 60% 50% 40% 30% Objective score 20% 10% 0% 0 10 20 Rank 30 40 50 60 L ITDG Tocc Tunocc EDT G C G125-G H/W W GL V/S0T Assuming Rank >20 is not acceptable 100% 90% 80% 70% Acceptance limit 60% 50% 40% 30% Objective score 20% 10% 0% 0 10 20 Rank 30 40 50 60 L ITDG Tocc Tunocc EDT G C G125-G H/W W GL V/S0T Uncertainty effect: 15% of Top20 rejected 100% 90% 80% 70% 60% 50% 40% 30% Objective score Salt Lake City, Symphony Hall 20% Tokyo, Bunka Kaikan 10% Costa Mesa, Segerstrøm Hall 0% 0 10 20 Rank 30 40 50 60 L ITDG Tocc Tunocc EDT G C G125-G H/W W GL V/S0T Uncertainty effect is one-sided, good news 100% 90% Phew! - this region is empty 80% – no halls are being 70% overestimated 60% 50% 40% 30% Objective score Salt Lake City, Symphony Hall 20% Tokyo, Bunka Kaikan 10% Costa Mesa, Segerstrøm Hall 0% 0 10 20 Rank 30 40 50 60 L ITDG Tocc Tunocc EDT G C G125-G H/W W GL V/S0T Testing predictions on unranked halls Score≥50% Replication supported Score<50% Replication NOT supported Valencia, Paleu de la Musica Seattle, Benaroya Hall *Lucerne, Cultural Ctr. Concert Hall Sao Paolo, Sala Sao Paulo *Manchester Bridgewater Hall Minneapolis, Minn. Orchestra Hall *Fort Worth, Bass Performance Hall Kuala Lumpur, DewanFil. Petronas Taipei Cultural Centre, Concert Hall Budapest, Patricia Hall Mexico City, Salla Nezahualcoyotl Denver Boettcher Hall Philadelphia, Verizon Hall *Olavshallen, Trondheim Baden-Baden Festspielhaus Sapporo Concert Hall Lahti, Sibelius/Talo Athens, Megaron Concert Hall Birmingham Symphony Hall Belfast, Waterfront Hall *Munich, Herkulessalle Rochester, NY, Eastman Theatre Odense, Koncerthus Nielsen Hall Caracas, Aula Magna Agree or disagree? Take the www.akutek.info Survey *Verified by survey Best fit criteria, 12 parameters, r2=0.75, r=0.86 Par L ITDG Tocc Tunocc EDT G C G125-G H/W W GL V/S0T max 38 31 2.14 3.00 (2.29) (5.0) 1.0 3.1 (1.3) 32 (2.5) 65 min 28 13 1.89 2.20 1.79 3.2 -0.7 1.0 0.77 (20) 0.3 57 weight 0.6 0.9 1.6 0.4 0.9 1.2 1.1 1.0 1.2 1.0 1.0 0.7 Uncertainty from limited value-range in data selection Par L ITDG Tocc Tunocc EDT G C G125-G H/W W GL V/S0T max 38 31 2.14 3.00 (2.29) (5.0) 1.0 3.1 (1.3) 32 (2.5) 65 min 28 13 1.89 2.20 1.79 3.2 -0.7 1.0 0.77 (20) 0.3 57 weight 0.6 0.9 1.6 0.4 0.9 1.2 1.1 1.0 1.2 1.0 1.0 0.7 Selection-related Uncertainty: Values in (paranthesis) are equal to limits in the data selection Uncertainty from limited value-range in data selection Bars indicate the acceptable value ranges; 0-100% is the total range of data values 100% 80% 60% 40% 20% 0% Online survey . Status: 343 votes from 36 respondents, total 77 halls; . 13 Halls with 7 votes or more: Correlation between Survey results and Beranek Ranking is promising 5,0 R² = 0,79 We need more data! 4,0 Survey is still open on Online www.akutek.info survey 3,0 scale 1 to 5 Link to survey 2,0 https://www.surveymon key.com/s/MMFMZ5W 1,0 0 10 20Rank 30 40 50 60 Black Box explanation Predicting response from a Black Box Insight is desired However, prediction cannot wait for insight I invariably prefer A over B Black Box explanation Is the brain alone the Black-Box? Or one box in a network of black boxes? I invariably prefer A over B Black Box Evolution For discussion: Preference evolved together with Music, Orchestras, Architecture, Technology, Population, Musical Instruments. The fittest survives. Who’s the species? Who’s the environment? Suggestion: They all are all I invariably prefer A over B Conclusions . Uncertainties in Preference-Predictors should be tested on existing halls . Increased number of halls reduces uncertainty in r2 . Parameter size and combinations influence on the maximum achevivable r2 . Data should include values of «too much» and «too little» Relationship between Parameter and Preference is Non-Linear . Uncertainty in building-desicions can be reduced by «safety-first» policy However, some could-have-been-good halls may not be built The cost of certainty may be loss of freedom in design .
Details
-
File Typepdf
-
Upload Time-
-
Content LanguagesEnglish
-
Upload UserAnonymous/Not logged-in
-
File Pages33 Page
-
File Size-