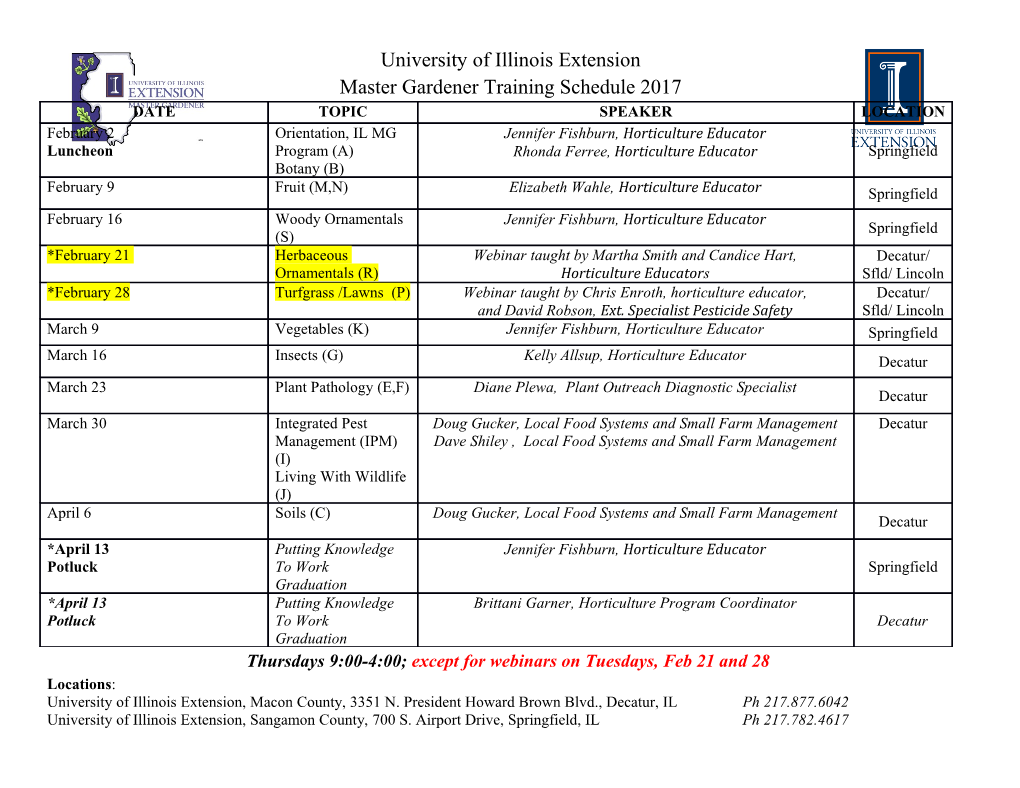
View metadata, citation and similar papers at core.ac.uk brought to you by CORE provided by University of Melbourne Institutional Repository Improving the Utility of Social Media with Natural Language Processing A thesis presented by Bo Han to The Department of Computing and Information Systems in total fulfillment of the requirements for the degree of Doctor of Philosophy The University of Melbourne Melbourne, Australia February 2014 Produced on archival quality paper Declaration This is to certify that: (i) the thesis comprises only my original work towards the PhD except where indi- cated in the Preface; (ii) due acknowledgement has been made in the text to all other material used; (iii) the thesis is fewer than 100,000 words in length, exclusive of tables, maps, bibliographies and appendices. Signed: Date: ⃝c 2014 - Bo Han All rights reserved. Thesis advisor(s) Author Timothy Baldwin Bo Han Paul Cook Improving the Utility of Social Media with Natural Language Processing Abstract Social media has been an attractive target for many natural language processing (NLP) tasks and applications in recent years. However, the unprecedented volume of data and the non-standard language register cause problems for off-the-shelf NLP tools. This thesis investigates the broad question of how NLP-based text processing can improve the utility (i.e., the effectiveness and efficiency) of social media data. In particular, text normalisation and geolocation prediction are closely examined in the context of Twitter text processing. Text normalisation is the task of restoring non-standard words to their standard forms. For instance, earthquick and 2morrw should be transformed into \earthquake" and \tomorrow", respectively. Non-standard words often cause problems for existing tools trained on edited text sources such as newswire text. By applying text nor- malisation to reduce unknown non-standard words, the accuracy of NLP tools and downstream applications is expected to increase. In this thesis, I explore and develop lexical normalisation methods for Twitter text. I shift the focus of text normalisation from a cascaded token-based approach to a type-based approach using a combined lexicon, based on the analysis of existing and developed text normalisation methods. The type-based method achieved the state-of-the-art end-to-end normalisation accu- racy at the time of publication, i.e., 0.847 precision and 0.630 recall on a benchmark dataset. Furthermore, it is simple, lightweight and easily integrable which is particu- larly well suited to large-scale data processing. Additionally, the effectiveness of the proposed normalisation method is shown in non-English text normalisation and other NLP tasks and applications. Geolocation prediction estimates a user's primary location based on the text of their posts. It enables location-based data partitioning, which is crucial to a range of tasks and applications such as local event detection. The partitioned location data can improve both the efficiency and the effectiveness of NLP tools and applications. In this thesis, I identify and explore several factors that affect the accuracy of text- based geolocation prediction in a unified framework. In particular, an extensive range of feature selection methods is compared to determine the optimised feature set for the geolocation prediction model. The results suggest feature selection is an effective method for improving the prediction accuracy regardless of geolocation model and location partitioning. Additionally, I examine the influence of other factors includ- Abstract iv ing non-geotagged data, user metadata, tweeting language, temporal influence, user geolocatability, and geolocation prediction confidence. The proposed stacking-based prediction model achieved 40.6% city-level accuracy and 40km median error distance for English Twitter users on a recent benchmark dataset. These investigations pro- vide practical insights into the design of a text-based normalisation system, as well as the basis for further research on this task. Overall, the exploration of these two text processing tasks enhances the utility of social media data for relevant NLP tasks and downstream applications. The devel- oped method and experimental results have immediate impact on future social media research. Contents Title Page .................................... i Abstract ..................................... iii Table of Contents ................................ v List of Figures .................................. viii List of Tables .................................. ix Citations to Previously Published Work ................... xii Acknowledgments ................................ xiii 1 Introduction 1 1.1 Background and Motivation ....................... 1 1.2 Aim and Scope .............................. 3 1.3 Contributions ............................... 6 1.4 Thesis Outline ............................... 9 2 Literature Review 13 2.1 Social Media ................................ 13 2.1.1 Twitter Data ........................... 16 2.1.2 Summary ............................. 21 2.2 Text Normalisation ............................ 21 2.2.1 Non-standard Words ....................... 22 2.2.2 Normalisation Task and Scope .................. 30 2.2.3 Methodologies ........................... 34 2.2.4 Recent Normalisation Approaches ................ 44 2.2.5 Summary ............................. 48 2.3 Geolocation Prediction .......................... 50 2.3.1 Background ............................ 50 2.3.2 Network-based Geolocation Prediction ............. 55 2.3.3 Text-based Geolocation Prediction ............... 57 2.3.4 Hybrid Methods .......................... 64 2.3.5 Summary ............................. 66 2.4 Literature Summary ........................... 67 v Contents vi 3 Text Normalisation 68 3.1 Normalisation Scope ........................... 68 3.2 Token-based Lexical Normalisation ................... 70 3.2.1 A Pilot Study on OOV Words .................. 70 3.2.2 Datasets and Evaluation Metrics ................ 73 3.2.3 Token-based Normalisation Approach .............. 74 3.2.4 Baselines and Benchmarks .................... 78 3.2.5 Results Analysis and Discussion ................. 79 3.2.6 Lexical Variant Detection .................... 83 3.2.7 Summary ............................. 88 3.3 Type-based Lexical Normalisation .................... 88 3.3.1 Motivation and Feasibility Analysis ............... 89 3.3.2 Word Type Normalisation .................... 90 3.3.3 Contextually Similar Pair Generation .............. 91 3.3.4 Pair Re-ranking by String Similarity .............. 95 3.3.5 Intrinsic Evaluation of Type-based Normalisation ....... 97 3.3.6 Error Analysis and Discussion .................. 103 3.4 Extrinsic Evaluation of Lexical Normalisation ............. 105 3.5 Non-English Text Normalisation ..................... 109 3.5.1 A Comparative Study on Spanish Text Normalisation ..... 110 3.5.2 Adapted Normalisation Approach ................ 111 3.5.3 Results and Discussion ...................... 114 3.6 Recent Progress on Text Normalisation ................. 117 3.6.1 Recent Normalisation Approaches ................ 117 3.6.2 Impact of Normalisation in Recent Research .......... 118 3.7 Summary ................................. 120 4 Geolocation Prediction 122 4.1 Introduction ................................ 122 4.2 Geolocation Prediction Framework ................... 126 4.2.1 Representation: Earth Grid vs. City .............. 126 4.2.2 Geolocation Prediction Models .................. 128 4.2.3 Feature Set ............................ 129 4.2.4 Data ................................ 131 4.2.5 Evaluation Measures ....................... 134 4.3 Finding Location Indicative Words ................... 135 4.3.1 Literature on Feature Selection ................. 136 4.3.2 Location Indicative Words .................... 137 4.3.3 Statistical-based Methods .................... 138 4.3.4 Information Theory-based Methods ............... 142 4.3.5 Heuristic-based Methods ..................... 144 4.4 Benchmarking Experiments on NA ................... 147 Contents vii 4.4.1 Comparison of Feature Selection Methods ........... 148 4.4.2 Comparison with Benchmarks .................. 150 4.5 Experiments on WORLD ......................... 155 4.6 Exploiting Non-geotagged Tweets .................... 157 4.7 Language Influence on Geolocation Predication ............ 160 4.8 Incorporating User Meta Data ...................... 167 4.8.1 Unlocking the Potential of User-declared Metadata ...... 168 4.8.2 Results of Metadata-based Classifiers .............. 169 4.8.3 Ensemble Learning on Text-based Classifiers .......... 171 4.9 Temporal Influence on Geolocation Model ............... 174 4.10 User Tweeting Behaviour ......................... 177 4.11 Prediction Confidence .......................... 180 4.12 Summary ................................. 183 5 Conclusion 185 5.1 Summary of Findings ........................... 185 5.2 Limitations and Future Work ...................... 192 5.3 A Tweet-length Summary of Thesis ................... 198 A Appendix 219 List of Figures 1.1 Examples of social media-based applications. .............. 2 1.2 A demonstration of geolocation prediction input and output for a pub- lic Twitter user. The red dot icon denotes the predicted city centre. 5 2.1 Word categorisations in text normalisation. ............... 31 2.2 From n-grams to a bipartite graph G = (W; C; E). ........... 44 3.1 Out-of-vocabulary word distribution in English Gigaword (NYT), Twit- ter and SMS data.
Details
-
File Typepdf
-
Upload Time-
-
Content LanguagesEnglish
-
Upload UserAnonymous/Not logged-in
-
File Pages232 Page
-
File Size-