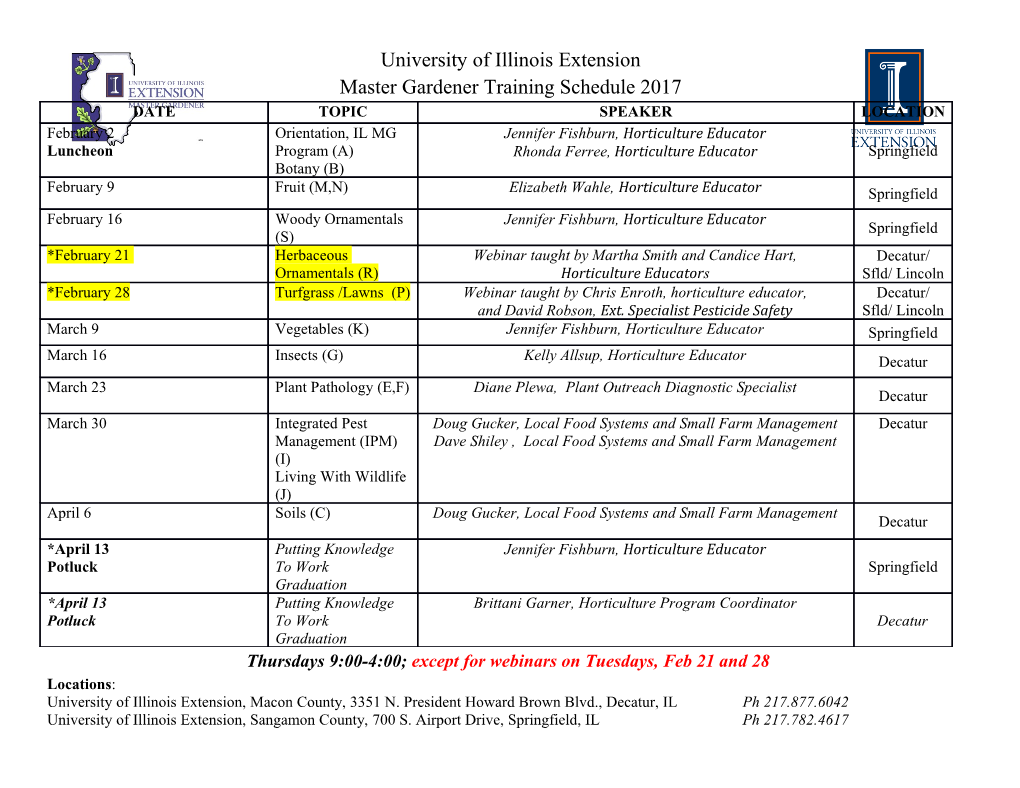
LC•GC Europe Online Supplement statistics and data analysis 13 Regression and Calibration Shaun Burke, RHM Technology Ltd, High Wycombe, Buckinghamshire, UK. One of the most frequently used statistical methods in calibration is linear regression. This third paper in our statistics refresher series concentrates on the practical applications of linear regression and the interpretation of the regression statistics. Calibration is fundamental to achieving to do this is least-squares regression, which possible for several different data sets to consistency of measurement. Often works by finding the “best curve” through yield identical regression statistics (r value, calibration involves establishing the the data that minimizes the sums of residual sum of squares, slope and relationship between an instrument squares of the residuals. The important intercept), but still not satisfy the linear response and one or more reference term here is the “best curve”, not the assumption in all cases (9). It, therefore, values. Linear regression is one of the most method by which this is achieved. There remains essential to plot the data in order frequently used statistical methods in are a number of least-squares regression to check that linear least-squares statistics calibration. Once the relationship between models, for example, linear (the most are appropriate. the input value and the response value common type), logarithmic, exponential As in the t-tests discussed in the first (assumed to be represented by a straight and power. As already stated, this paper paper (10) in this series, the statistical line) is established, the calibration model is will concentrate on linear least-squares significance of the correlation coefficient is used in reverse; that is, to predict a value regression. dependent on the number of data points. from an instrument response. In general, [You should also be aware that there are To test if a particular r value indicates a regression methods are also useful for other regression methods, such as ranked statistically significant relationship we can establishing relationships of all kinds, not regression, multiple linear regression, non- use the Pearson’s correlation coefficient just linear relationships. This paper linear regression, principal-component test (Table 1). Thus, if we only have four concentrates on the practical applications regression, partial least-squares regression, points (for which the number of degrees of of linear regression and the interpretation etc., which are useful for analysing instrument freedom is 2) a linear least-squares of the regression statistics. For those of you or chemically derived data, but are beyond correlation coefficient of –0.94 will not be who want to know about the theory of the scope of this introductory text.] significant at the 95% confidence level. regression there are some excellent However, if there are more than 60 points references (1–6). What do the linear least-squares an r value of just 0.26 (r2 = 0.0676) would For anyone intending to apply linear regression statistics mean? indicate a significant, but not very strong, least-squares regression to their own data, Correlation coefficient: Whether you use a positive linear relationship. In other words, it is recommended that a statistics/graphics calculator’s built-in functions, a a relationship can be statistically significant package is used. This will speed up the spreadsheet or a statistics package, the but of no practical value. Note that the test production of the graphs needed to first statistic most chemists look at when used here simply shows whether two sets confirm the validity of the regression performing this analysis is the correlation are linearly related; it does not “prove” statistics. The built-in functions of a coefficient (r). The correlation coefficient linearity or adequacy of fit. spreadsheet can also be used if the ranges from –1, a perfect negative It is also important to note that a routines have been validated for accuracy relationship, through zero (no relationship), significant correlation between one (e.g., using standard data sets (7)). to +1, a perfect positive relationship variable and another should not be taken (Figures 1(a–c)). The correlation coefficient as an indication of causality. For example, What is regression? is, therefore, a measure of the degree of there is a negative correlation between In statistics, the term regression is used to linear relationship between two sets of time (measured in months) and catalyst describe a group of methods that data. However, the r value is open to performance in car exhaust systems. summarize the degree of association misinterpretation (8) (Figures 1(d) and (e), However, time is not the cause of the between one variable (or set of variables) show instances in which the r values alone deterioration, it is the build up of sulfur and another variable (or set of variables). would give the wrong impression of the and phosphorous compounds that The most common statistical method used underlying relationship). Indeed, it is gradually poisons the catalyst. Causality is, 14 statistics and data analysis LC•GC Europe Online Supplement (n Ϫ1) RSE ϭ s 1 Ϫr2 (y) (n Ϫ2) where s(y) is the standard deviation of the y values in the calibration, n is the number of in fact, very difficult to prove unless the data pairs and r is the least-squares regression correlation coefficient. chemist can vary systematically and independently all critical parameters, while Confidence intervals measuring the response for each change. As with most statistics, the slope (b) and intercept (a) are estimates based on a finite sample, so there is some uncertainty in the values. (Note: Strictly, the uncertainty arises Slope and intercept from random variability between sets of data. There may be other uncertainties, such as In linear regression the relationship measurement bias, but these are outside the scope of this article.) This uncertainty is between the X and Y data is assumed to quantified in most statistical routines by displaying the confidence limits and other be represented by a straight line, Y = a + statistics, such as the standard error and p values. Examples of these statistics are given in bX (see Figure 2), where Y is the estimated Table 2. response/dependent variable, b is the slope (gradient) of the regression line and a is the intercept (Y value when X = 0). This straight-line model is only appropriate if Degrees of freedom Confidence level the data approximately fits the assumption (n-2) 95% ( = 0.05) 99% ( = 0.01) of linearity. This can be tested for by 2 0.950 0.990 plotting the data and looking for curvature 3 0.878 0.959 (e.g., Figure 1(d)) or by plotting the 4 0.811 0.917 residuals against the predicted Y values or 5 0.754 0.875 X values (see Figure 3). 6 0.707 0.834 Although the relationship may be known to be non-linear (i.e., follow a different 7 0.666 0.798 functional form, such as an exponential 8 0.632 0.765 curve), it can sometimes be made to fit the 9 0.602 0.735 linear assumption by transforming the data 10 0.576 0.708 in line with the function, for example, by 11 0.553 0.684 taking logarithms or squaring the Y and/or 12 0.532 0.661 X data. Note that if such transformations 13 0.514 0.641 are performed, weighted regression 14 0.497 0.623 (discussed later) should be used to obtain an accurate model. Weighting is required 15 0.482 0.606 because of changes in the residual/error 20 0.423 0.537 structure of the regression model. Using 30 0.349 0.449 non-linear regression may, however, be a 40 0.304 0.393 better alternative to transforming the data 60 0.250 0.325 when this option is available in the Significant correlation when |r| ≥ table value statistical packages you are using. Residuals and residual standard error 1 A residual value is calculated by taking the difference between the predicted value 0.8 and the actual value (see Figure 2). When 0.6 the residuals are plotted against the predicted (or actual) data values the plot 0.4 becomes a powerful diagnostic tool, 0.2 enabling patterns and curvature in the data to be recognized (Figure 3). It can also be 0 5 1015 2025303540455055 60 used to highlight points of influence (see -0.2 Bias, leverage and outliers overleaf). The residual standard error (RSE, also -0.4 Correlation coefficient (r) known as the residual standard deviation, -0.6 RSD) is a statistical measure of the average 95% confidence level -0.8 residual. In other words, it is an estimate 99% confidence level of the average error (or deviation) about -1 the regression line. The RSE is used to Degrees of freedom (n-2) calculate many useful regression statistics including confidence intervals and outlier test values. table 1 Pearson's correlation coefficient test. LC•GC Europe Online Supplement statistics and data analysis 15 The p value is the probability that a value could arise by chance if the true value was x data for the n points in the calibration. zero. By convention a p value of less than 0.05 indicates a significant non-zero statistic. RSE is the residual standard error for the Thus, examining the spreadsheet’s results, we can see that there is no reason to reject the calibration. hypothesis that the intercept is zero, but there is a significant non-zero positive If we want, therefore, to reduce the size gradient/relationship. The confidence intervals for the regression line can be plotted for all of the confidence interval of the prediction points along the x-axis and is dumbbell in shape (Figure 2).
Details
-
File Typepdf
-
Upload Time-
-
Content LanguagesEnglish
-
Upload UserAnonymous/Not logged-in
-
File Pages6 Page
-
File Size-