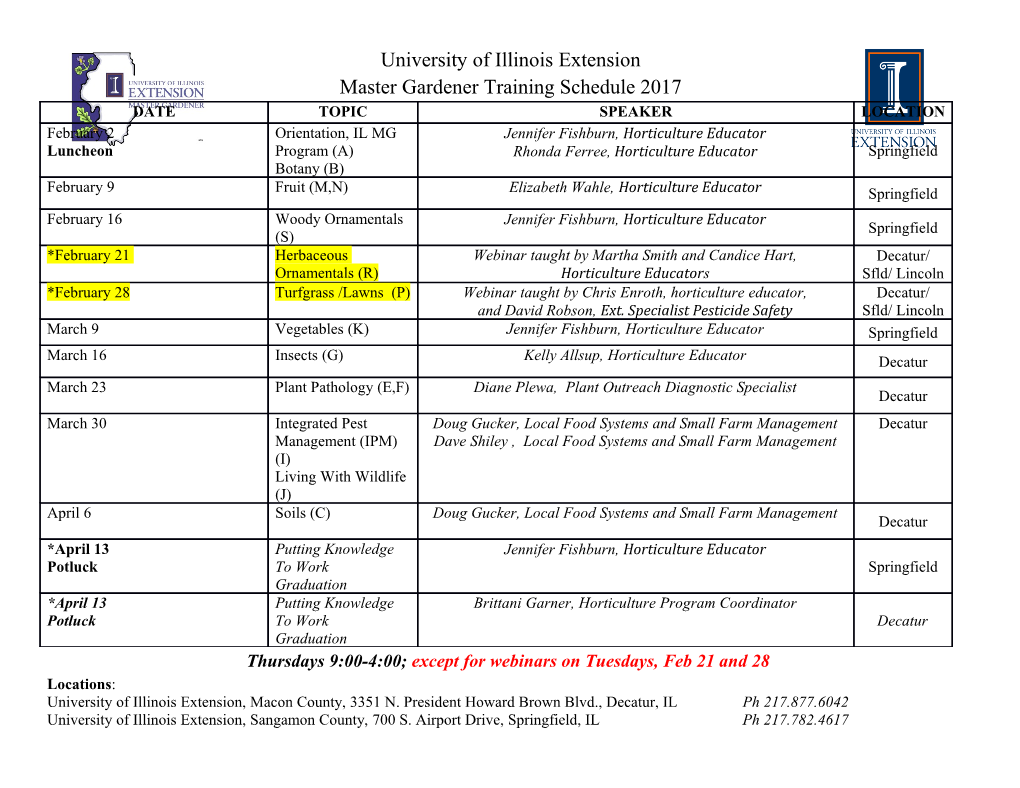
A Case Study of the Actor-Network Effects of Moneyball on Major League Baseball A Research Paper submitted to the Department of Engineering and Society Presented to the Faculty of the School of Engineering and Applied Science University of Virginia • Charlottesville, Virginia In Partial Fulfillment of the Requirements for the Degree Bachelor of Science, School of Engineering Corey Nolan Spring 2020 On my honor as a University Student, I have neither given nor received unauthorized aid on this assignment as defined by the Honor Guidelines for Thesis-Related Assignments Advisor Sean M. Ferguson, Department of Engineering and Society Introduction Entering the 2002 Major League Baseball (MLB) season, the Oakland Athletics found themselves in a tough spot. As one of the poorest organizations in the MLB, they were unable to re-sign three star players Jason Giambi, Johnny Damon, and Jason Isringhausen as the New York Yankees, Boston Red Sox, and St. Louis Cardinals, three large-market, powerhouse organizations, signed the free agents to deals the Athletics could not match. Desperate to keep his team competitive but unable to afford the exorbitant price tag of high-profile athletes, Billy -minded assistant Paul DePodesta to find a way their team could compete with larger market teams with only a third of their salary cap. DePodesta was long a student to find a way the Athletics could compete (A Guide to Sabermetric Research, 2020). Sharply departing from tradition scouting theory, the theory DePodesta and Beane discovered did not emphasize how an athlete looks or their physical tools; instead, it asked if a player could get on base or hit, meaning players with higher on-base (OBP) and slugging percentages (SLG). many runs a team scores, therefore maximizing their chance to win games. With about $40 million to assemble a competitive team, Beane took a gamble and trusted his assistant, having faith that the objective analytics would help them field a more competitive team than Athletics clinching their division in 2002 and 2003 with a team consisting of many players -competitive and at best Double-A or Triple-A talent (Sickels, 1 2011). inated the league in wins per dollar spent, tying the Yankees for most wins while spending less than one-third on their payroll (Figure 1). Figure 1 shorthand for anything related to analytics in sports can be seen in entertainment (how fans watch the game and what statistics they pay attention to), sports betting, and even in other sports like soccer, where managers and statisticians replicated the Moneyball soccer labor market undervalues players with higher total distances run per game (Weimar & Wicker, 2017). This massive network effect stemming from Moneyball is very intriguing and complex, but it is important to narrow the focus of my research to understand the strongest actor- network effects that occurred. Therefore, this research paper will serve as a case study on the Actor-Network theoretical framework applied to the effects of Moneyball on the MLB, specifically the actor-network of the players, management of organizations, and analytics. 2 Theoretical Framework of the Actor-Network Theory The Actor-Network Theory (ANT) is a theoretical framework used to consider the shifting networks between society and technological achievements that assigns agency to both human and non-human actors. It treats these actors or actants as active participants in a dynamically changing network of relationships, holding that no actor acts alone. ANT considers every factor in the social and natural worlds as actors in a continuously woven web of relations within which they are located (Law, 2009). Darnell, Giulianotti, Howe, and Collison (2018) hold hen new ideas or movements are formed research paper. To understand how ANT is an appropriate framework for my research, I reviewed Reassembling the Social: an Introduction to Actor-Network-Theory and the first three sources of uncertainties of ANT he explores in this book. The first source of uncertainty holds that there are no groups, only group formations that are constantly formed and re-formed et al., 2018, p. 91). The third source of uncertainty holds that if society cannot explain power, which requires explanation in ANT, then the notion of agency extends past humans and human action. Essential to my research, the first source of uncertainty holds with the Moneyball movement, as play ly changing, shaping each other, and being re-formed. I support this claim later in this paper with clear evidence regarding how each actor drastically changed after the spread of Moneyball. 3 In the second source of uncertainty, actions and agencies lead to the previously mentioned social action and organization. Applied to my topic, Michael Lewis solely possessed the agency of publishing Moneyball, which had a profound effect on players, management, and analytics. One of the greatest network effects of Moneyball was a shift in . From a talent acquisition standpoint, every baseball team has always had its power to oppose the power of other teams in signing free agents. In the team-player dynamic, teams generally held all of the power, as they were the ones cutting the checks. After the Moneyball movement spread across the League, the previously undervalued players gained power. These players understood what was occurring and used their newfound power to leverage their salaries to pit teams against each other in their own power struggle, just like superstars have always done. Over time, these players began to lose their leveraging power as they were now appropriately compensated and the player market had adjusted (Brown, Link, & Rubin, 2017) and are experiencing the endgame of Moneyball (Sheinin, 2018). With the third source of uncertainty, analytics are non-human factors with notable agency. In regards to my research, neither a player's skill nor changes in the management of organizations can directly explain the previously mentioned power as the shift in power was fueled by analytics. Therefore, because these newfound analytics "modify a state of affairs by making a difference" it is reasonable to consider agency beyond humans only to non-human actants (Latour, 2005). For this reason, I hold that analytics are appropriately considered an actor through the ANT framework. In baseball, we can apply Darnell (2018), who uses ANT to paint a more specific picture about how agency led to action and organization in sports development in Jamaica, to better 4 understand the network-effects of the Moneyball revolution. Harnessing analytics allowed organizations and players to better understand the value of a player. Teams changed their assessment schemes from what a player looks like on the field to what the data informs them, which is how teams still operate today. The analytics related to Moneyball were black-boxed and As seen, the agentic forces of analytics led to organizational changes, but they also empowered players to train to become what organizations now view as more valuable. Additionally, in the complicated network of organizational decision-making, owners do not micromanage those below them. Therefore, the relationships between coaches, hiring managers, and scouts were impacted most significantly as they, in the complicated nexus of a baseball team, had to completely reassess what players are now more valuable, what players are available, and then go fill their talent gap with these players. This is important to have in your mind going forward as I am black-boxing the actual analytics related to Moneyball and focusing more on using ANT to look at the relationships between players, organizational management, and analytics as a whole. Evidence and Data Collection Using ANT, I draw upon the following set of evidence: the propagation of Moneyball -game strategy, player off- nce to better understand the actor-network effects of Moneyball and the mutually shaping relationships among players, organizational management, and analytics. Propagation of Moneyball across the MLB 5 While the change Beane and DePodesta brought to the Athletics in 2002 was an incredible turning point for their organization, the Moneyball effect led to a paradigm shift that changed the entire game of b following their shift to statistics-based drafting and player acquisition was the focus of Michael (2003) book Moneyball: The Art of Winning an Unfair Game, and the sabermetrics approach to drafting and scouting quickly gained traction throughout the league. In 2004, the Boston Red Sox u advice to hire Theo Epstein, who shared the same analytical approach to the game as both , p. 184). The following year, the Red Sox won the World Series. By this time, the Moneyball approach to baseball proved itself with a small market team clinching their division twice and a large market team winning the World Series. With ANT, I discovered the connection between James and DePodesta to be a leader- follower relationship. Bill James developed his analysis of baseball over two decades and became a network builder in the baseball analytics community. DePodesta followed his teachings as an acolyte. These are not two random people out of the woodworks rather two baseball fanatics with a passion to discover the objective truths about baseball. They both had specific training in analytics and took their social capital with them, as James was hired by the Red Sox and DePodesta became the chief strategy officer for the Cleveland Browns. There is a very clear power in their association with sabermetrics. Before diving into more analytics, it is important to remember why the Athletics decided to do something absolutely different existing relationship with Beane, who was in a position where he would completely change his 6 operation because of their dismal prospect on the future season with small-cap space and the departure of three stars. Hired as the GM and Assistant GM, Beane and DePodesta were two of the most prominent actors in the clubhouse and had the power to do something completely different, which is exactly what they did.
Details
-
File Typepdf
-
Upload Time-
-
Content LanguagesEnglish
-
Upload UserAnonymous/Not logged-in
-
File Pages26 Page
-
File Size-