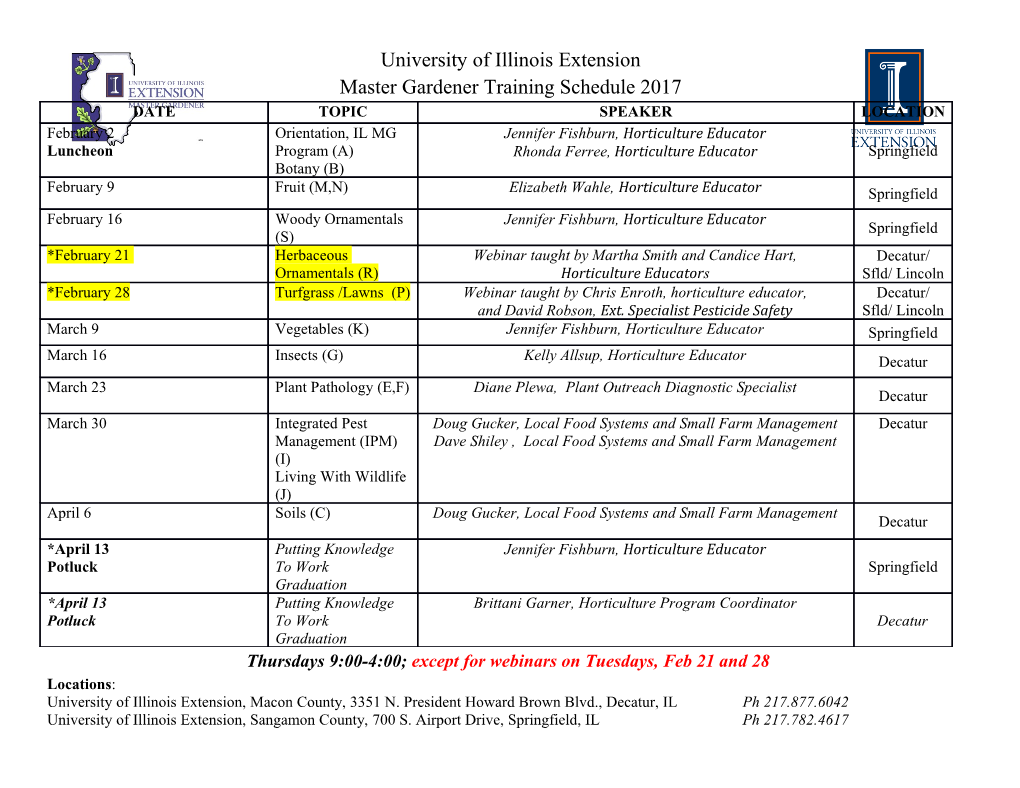
Journal of Rehabilitation in Civil Engineering 7-3 (2019) 15-32 Journal homepage: http://civiljournal.semnan.ac.ir/ Dam Seepage Prediction Using RBF and GFF Models of Artificial Neural Network; Case Study: Boukan Shahid Kazemi's Dam 1* 2 1 S. Emami , Y. Choopan and J. Parsa 1. Water Engineering Department, Faculty of Agriculture, University of Tabriz, Tabriz, Iran 2. Water Engineering Department, Faculty of Agriculture, University of Gorgan, Gorgan, Iran Corresponding author:[email protected] ARTICLE INFO ABSTRACT Article history: Received:11 February 2018 Dams have been always considered as the important Accepted: 11 June 2018 infrastructures and their critical values measured. Hence, evaluation and avoidance of dams’ destruction have a Keywords: specific importance. In this study seepage of the Embankment Dam Seepage, embankmentof Boukan Shahid Kazemi’s dam in Iran has Ann, Rbf Model, been analyzed via RBF (radial basis function network) and Gff Model, GFF (Feed-Forward neural networks) models of Artificial Boukanshahidkazemi's Dam. Neural Network (ANN). RBF and GFF of ANN models were trained and verified using each piezometer’s data and the water levels difference of the dam. To achieve this goal,based on the number of data and inputs,864piezometric data set were used, of which 80% (691 data) was used for the training and 20% (174 data) for the testing the network.The results showed good agreement between observed and predicted values and concluded the RBF model has high potential in estimating seepage with Levenberg Marquardt training and 4 hidden layers. Also the values of statistical parameters R2 and RMSE were 0.81 and the 33.12. 1. Introduction irrecoverable. The study of dam behaviourconsists of three periods; One of the ways to solve the water scarcity construction, first dewatering and the problem is to control surface waters. Dam operation time. construction and water storage are considered For providing useful information about the as one of the best methods to control water possible problems of the embankment, surface. The behavior of dams should be earthquake and its effects on dam, pore always evaluated and controlled due to the pressure of the core, and the vertical and fact that their construction’s cost are too high horizontal displacements should be studied in and the damage of their destruction is all three stages. DOI: 10.22075/JRCE.2018.13986.1254 16 S. Emami et al./Journal of Rehabilitation in Civil Engineering 7-3 (2019) 15-32 To study dam behavior, numerical methods 0.95, 0.232 and 0.205 respectively, and for were used. Since these methods are usually test data were 0.93, 0.477 and 0.125 difficult, time-consuming and require respectively. In his study, hidden layers and complex programming researchers were various activity functions were used to obtain looking for easier ways with less time and the best results. Finally, the network with the cost [1]. sigmoid activity function and one hidden layers yielded the most accurate result [2]. Artificial neural networks which are modeled on biological neural networks can be helpful Nourani et al. analyzed the piezometric in solving problems such as the above height at the core of Sattarkhan embankment problem. Now, these networks which are dam in Iran using artificial neural networks considered as intelligent systems, are used in (ANNs). The ANNs with details of the three different science including water engineering layer prediction with the Levenger-Marqurdt with a wide variety of constructions. algorithm post-propagation trained the data. While for the integrated FFBP network, the Generally, it can be said that in any given number of hidden neurons was 6 and for the case which learning a linear or nonlinear integrated RBF network the radius was mapping and a special space would be considered 0.5, the hidden neurons for single needed, these networks can ideally perform network were 5 and 7. The results of their this conversion [1]. study showed a good adaptation between Recently, with the development of software prediction and measured values. The programs, we can predict different correlation coefficient (R2) for the single phenomena with high accuracy. Artificial network was 0.798 and for the FFBF and neural networks are one of the good and RBF networks were 0.67 and 0.87, accurate predicting methods employed in respectively. They concluded that the results different disciplines, including water of artificial neural networks are closer to resources engineering. reality, compared to the numerical methods which have been used in previous studies [3]. Ersayin examined the seepage of the dam embankment, specifically. In his study, after Pourkarimi et al. presented a new method for a comprehensive introduction of various determining the seepage flow from the types of dams and seepage phenomenon, this foundation and body of embankment dam phenomenon was modeled using artificial based on data analysis methods. After neural networks (ANNs). In his thesis, studying Fileh-Khaseh embankment dam in Ersayin used a set of data including 125 Zanjan province in Iran, the seepage of piezometric data collected from the Jeziorsko embankment’s soil was estimated by the earth fill dam in Poland to train and test his finite element software called SEEP. In his proposed model. Upstream and downstream study, a set of 96 data on effective parameters water levels as inputs and water surface of of seepage including permeability coefficient piezometers were considered as output. The of the foundation and water heights behind statistical parameters used in his study were the dam in allowable range were produced. consisted of; correlation coefficient (R2), root Then, 65 and 31 data were used for training mean square error (RMSE) and MAE. The and testing the model respectively. The values of these parameters for train data were results showed that ANN provides an S. Emami et al./ Journal of Rehabilitation in Civil Engineering 7-3 (2019) 15-32 17 effective appliance for detecting patterns in showed the seepage foundation of dam data and accurate prediction of seepage from decreased to 0.05 that it ignorable [8]. the foundation and body of Filleh-Khaseh Nourani and Babakhani predicted seepage dam. The statistical parameters used in this from Sattarkhan dam using RBF model. The study were R2, RMSE, MSE and MAE. The results of RBF model indicated this method values of these parameters for the train data has better accuracy and less computation in were 0.93, 43, 1870 and for the test data were comparison with the finite difference method 0.092, 17 and 1841 respectively [4]. [9]. Kamanbedast and Delvari Analyzed seepage Naderpour et al. estimated the Shear Strength and stability of earth dam using Ansys and Capacity of Masonry Walls Improved with Geo-Studio software.Therefore, result wore Fiber Reinforced Mortars (FRM) Using compared whit Geo studio Software result. ANN-GMDH Approach. The results showed Firstly, Dam were studied with using there the proposed model (ANN-GMDH) has a analysis method, then seepage are predicated correlation coefficient of 0.95, which the seepage rate in Ansys, 18% is lower than represented the high efficiency of the model Geo-Studio results. Results showed the [10]. significant difference of two software is related to safety factor and eventually it can Jamal et al. predicted the amount of be deducted that Ansys answer is more discharge to the clay core of embankment acceptable. Brifly, as a result, dam is at dams under unstable permeability conditions suitable situation according to the software using ANN. In his study, finite element results and Just vertical settlement at core models were constructed and analyzed for zone should be studied more and perfectly non-saturated conditions. Then the results [5]. Ebtehaj and Bonakdari (2013), Evaluated with input conditions were used to train the of Sediment Transport inSewer using perceptron neural network model. The results Artificial Neural Network (ANNs). They of this research indicate the ability of ANN to reported in comparison with existing accurately predict seepage of embankment methods, the ANN showed acceptable results dam in unsaturated condition [11]. [6]. Santillán et al. analyzed dam seepage by Aljeyri attempted to investigate soil dam means of an artificial neural network model. behavior using Ansys. In this study, H was Results showed artificial neural network assumed that, none impervious layer behind models a powerful tool for predicting and layer are exist and downstream seepage is understanding seepage phenomenon [12]. influenced by each change of two impervious layers which are concluded dams [7]. Naderpour et al. predicted the torsional strength of RC beams strengthened with FRP Shamsaie et al. studied the behavior of sheets by using neural network and reported Mahabad dam using numerical methods. In it was possible to achieve a satisfactory result their study, the status of Mahabad dam was with less cost and time by training the analyzed for changes in seepage. After artificial neural networks [13]. constructing the cutoff wall and grout curtain at foundation of dam, numerical simulation Ebtehaj et al. predicted sediment transport in sewers using an expert system with radial 18 S. Emami et al./Journal of Rehabilitation in Civil Engineering 7-3 (2019) 15-32 basis function neural network based on - Determine the best topology of ANN model decision trees. The results of DT-RBF are (number of nodes and neurons, number of compared with RBF and RBF particle swarm layers and appropriate training function). optimization (PSO), which uses PSO for RBF training. It appears that DT-RBF is 2. Materials and Methods more accurate R2= 0.934, MARE = 0.103, RMSE = 0.527, SI = 0.13, BIAS = -0.071) 2.1.Area of Study than the two other RBF methods [14]. In order to use artificial neural network Naderpour et al. used artificial neural (ANN) method to predict seepage value from networks (ANNs) for compressive strength dam body, ShahidKazemiBoukan dam prediction of environmentally friendly located in Boukan city of west Azarbaijan concrete.
Details
-
File Typepdf
-
Upload Time-
-
Content LanguagesEnglish
-
Upload UserAnonymous/Not logged-in
-
File Pages18 Page
-
File Size-