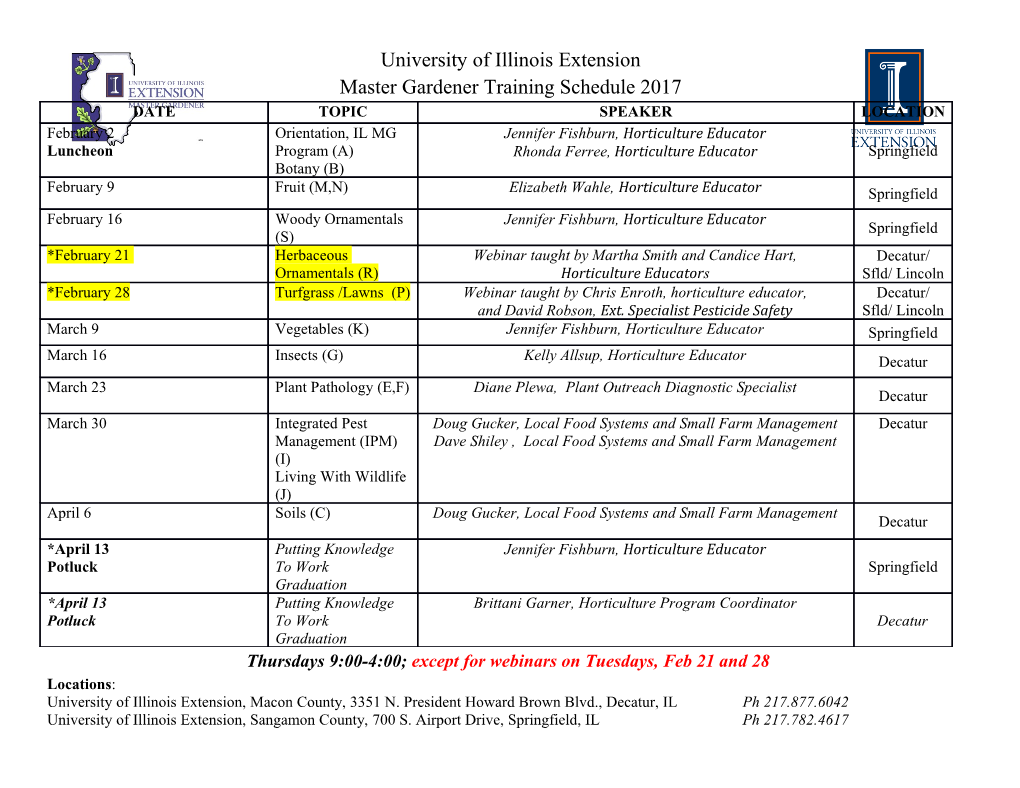
WSEAS TRANSACTIONS on MATHEMATICS Jyh-Jiuan Lin, Ching-Hui Chang, Rosemary Jou A NOTE ON SKEW-NORMAL DISTRIBUTION APPROXIMATION TO THE NEGATIVE BINOMAL DISTRIBUTION 1 2* 3 JYH-JIUAN LIN , CHING-HUI CHANG AND ROSEMARY JOU 1Department of Statistics Tamkang University 151 Ying-Chuan Road, Tamsui, Taipei County 251, Taiwan [email protected] 2Department of Applied Statistics and Information Science Ming Chuan University 5 De-Ming Road, Gui-Shan District, Taoyuan 333, Taiwan [email protected] 3 Graduate Institute of Management Sciences, Tamkang University 151 Ying-Chuan Road, Tamsui, Taipei County 251, Taiwan [email protected] Abstract: This article revisits the problem of approximating the negative binomial distribution. Its goal is to show that the skew-normal distribution, another alternative methodology, provides a much better approximation to negative binomial probabilities than the usual normal approximation. Key-Words: Central Limit Theorem, cumulative distribution function (cdf), skew-normal distribution, skew parameter. * Corresponding author ISSN: 1109-2769 32 Issue 1, Volume 9, January 2010 WSEAS TRANSACTIONS on MATHEMATICS Jyh-Jiuan Lin, Ching-Hui Chang, Rosemary Jou 1. INTRODUCTION where φ and Φ denote the standard normal pdf and cdf respectively. The This article revisits the problem of the parameter space is: μ ∈( −∞ , ∞), σ > 0 and negative binomial distribution approximation. Though the most commonly used solution of λ ∈(−∞ , ∞). Besides, the distribution approximating the negative binomial SN(0, 1, λ) with pdf distribution is by normal distribution, Peizer f( x | 0, 1, λ) = 2φ (x )Φ (λ x ) is called the and Pratt (1968) has investigated this problem standard skew-normal distribution. When thoroughly and proposed a different method λ = ,0 the above pdf (1.1) boils down to the which outperforms the normal approximation. N(μ , σ 2 ) pdf. Also parameter λ > 0 (< 0) However, the methodology used in Peizer and implies positively (negatively) skewed shape Pratt (1968) is rather complicated and difficult. of f( x | μ, σ , λ) above. For more With different motive, we propose another skew-normal distribution’s properties, one can alternative, the skew-normal distribution, and see Azzalini (1985), Gupta, Nguyen and show that it provides a much better Sanqui (2004), Arnold and Lin (2004) or approximation to negative binomial Chang et al. (2008). probabilities than the usual normal approximation. It seems that the skew-normal The skew-normal distribution is first distribution is a better choice than the normal introduced by O’Hagan and Leonard (1976), distribution to approximate the discrete which is a generalization of the normal distribution. The reason is that the distribution. Its definition is as follows. skew-normal distribution can take negatively skewed, symmetric or positively skewed A r.v. X is said to follow a skew-normal shape through its skew parameter and hence is distribution with location parameter μ, scale more versatile than the normal distribution. parameter σ and skew parameter λ (the Comparing to the normal distribution, Chang SN(μ , σ 2 , λ) distribution) if the pdf of X is et al. (2008) has shown that the extra (skew) given as parameter λ would provide a better approximation of the binomial distribution by f( x |μ , σ , λ) the SN(μ, σ2 , λ) distribution. Besides, = (2 /σ )φ ((x −μ ) /σ ) Φ (λ (x − μ ) /σ ), (1.1) Chang et al. (2008) has also mentioned that it ISSN: 1109-2769 33 Issue 1, Volume 9, January 2010 WSEAS TRANSACTIONS on MATHEMATICS Jyh-Jiuan Lin, Ching-Hui Chang, Rosemary Jou should also work for negative binomial applying to various areas. For the applications distribution approximation. However, a lot of of the CLT, please see Wang and Wei (2008), details of the numerical results are not Giribon, Revetria and Oliva (2007), Takacs, reported in Chang et al. (2008). Therefore, we Nagy and Vujacic (2007) and Mceachen et al will show that the extra (skew) parameter λ (2005). By the Central Limit Theorem, the cdf would provide a better approximation of the of NB(r, p) (negative binomial distribution negative binomial distribution by the where Y represents the number of failures th SN(μ , σ2 , λ) distribution than the normal before the r success) at k, k ∈ ,2,1,0{ K}, distribution in this paper along with the Fr, p ( k ), i.e., P( Y≤ k), is approximated by details. the normal cdf as The skew-normal distribution and its Fr, p ()k ≈ Φ((p ( k + 0.5)− rq ) / rq ) usefulness to approximate the negative binomial distribution is discussed in the next where q = 1− p. section. The usual normal approximation method is also introduced in Section 2 and 2.2. Skew-Normal Approximation numerical results and comparisons are For a given NB(r, p) distribution, the provided in Section 3. parameters of the approximating (or, matching) SN(μ , σ2 , λ) distribution are 2. APPROXIMATION BY THE found by equating the first three central NORMAL AND SKEW-NORMAL moments, i.e., DISTRIBUTIONS (a) EY() = rq /(p= E X ) In this section we’ll see how the 2 SN(μ , σ2 , λ) distribution can be used to = μ + σ ( 2 /π )( λ / 1+ λ ); approximate a negative binomial distribution. (b) EY( −E( Y ))2 = rq / p2 = E ( X − E ( X ))2 2.1. Normal Approximation by the CLT = σ 2{1− (2 /π ) λ2 /(1+ λ2 )}; It is a common practice to approximate the sampling distribution of the sample mean by (c ) EY( −E( Y ))3 = rq (1 + q ) / p3 the fact of the Central Limit Theorem (CLT). = E(XEX− ( ))3 The CLT is a very powerful theorem and is = σ 3 ( 2 /π )( λ / 1+ λ2 ) 3 ((4 / π )− 1). ISSN: 1109-2769 34 Issue 1, Volume 9, January 2010 WSEAS TRANSACTIONS on MATHEMATICS Jyh-Jiuan Lin, Ching-Hui Chang, Rosemary Jou Solving the above three equations is in order to make the skew-normal straight forward. We obtain the values of λ, approximation work, i.e., σ and μ as follows. Fr, p ( k )≈ Ψλ (( k + 0.5− μ ) /σ ). Since the skewness of the skew-normal distribution lies (A) Given (r , p), the above last two in (-0.99527, 0.99527), the skew-nor mal equations yield approximation is applied only when the λ = {(rq (2 /π )((4 / π )− 1) /(1 + q ))2 / 3 skewness of NB(r, p), (2−p ) / r (1 − p ), lies in this interval. However, the negative +(2 /π ) − 1}−1/ 2 binomial distribution is always positively (B) Given (r , p) and after obtaining λ as skewed. Hence, strictly speaking, the above, σ is found as restriction is that the skewness of NB(r, p) lies in (0, 0.99527). σ = (rq / p ) /{1− (2/π ) λ2 /(1+ λ2 )} 1/2 . 2.4. Evaluation Criterion (C) Finally, after obtaining λ and σ as Chang et. al. (2008) uses the “maximal above, get μ as absolute error (MABS)” of the skew-normal approximation to investigate the binomial μ =( rq/) p −σ (2/)/1 π λ+ λ2 . approximation B(n, p) as in Schader and Schmid (1989) (though their work is related After obtaining λ, σ and μ as to the normal approximation to the binomial given above, the negative binomial cdf is distribution). MABS can be substantial when p approximated as is near 0 or 1. In this paper we will also adopt the MABS criterion for this negative binomial F( k )≈ k+0.5 f ( x |μ , σ, λ)dx r, p ∫−∞ approximation problem and it is defined as = Ψλ ((k + 0.5− μ ) /σ ), * c MABS( r , p)= max | Fr, p ( k )− Fr, p ( k ) | k∈ RY where Ψλ (c ) = 2φ (z )Φ ( λ z ) dz is the ∫−∞ (2.1) standard skew-normal cdf. * where Fr, p ( k )= Φ (( p ( k + 0.5) − rq ) / rq ) 2.3. Restriction is for the normal case and A mild restriction is put on r and p, which is * Fr, p () k= Ψλ (( k + 0.5 − μ ) / σ ) is for the (2−p ) / r (1 − p ) < 0.99527, ISSN: 1109-2769 35 Issue 1, Volume 9, January 2010 WSEAS TRANSACTIONS on MATHEMATICS Jyh-Jiuan Lin, Ching-Hui Chang, Rosemary Jou skew-normal case. Y~ N ( r (1− p )/ p = 6.6667, r (1− p )/ p2 = 8.8889) (3.2) 3. EXAMPLES AND NUMERICAL RESULTS ENA= Fr, p ( k ) − Φ (( p ( k + 0.5) − rq ) / rq ) As a demonstration of the improved (3.3) approximation of the negative binomial distribution by the skew-normal distribution, ESA= Fr, p ( k )− Ψλ (( k + 0.5 − μ ) /σ ) (3.4) we first show the matching skew-normal (as Next, we calculate the MABS (see Eq. in Eq. (3.1) and normal (as in Eq. (3.2)) pdfs (2.1)) for the skew-normal approximations as for the negative binomial distribution well as the normal approximations ()Y~ NB ( r= 20, p = 0.75 ) in the Figure 3.1 (henceforth called MABS(SN) and MABS(N), and the errors are reported in the Table 3.1. respectively) for various r and p. The results Here ENA and ESA stand for “Error in are reported in Table 3.2. On the other hand, Normal Approximation” and “Error in Figure 3.2 shows the plots of MABS(SN) and Skew-Normal Approximation”, and they are Figure 3.3 shows the plots of MABS(N) as a defined as in (3.3) and (3.4) respectively. It function of p (for fixed r = 20) and r (for can be seen that the skew-normal curve fixed p = 0.75). Plots for other values of r follows the asymmetric negative binomial showed almost identical patterns. The results distribution closely. and plots both clearly show that the Y~ SN (μ = 3.4107, σ 2 = (4.4148)2 ,λ = 2.4224) skew-normal approximation is much superior (3.1) to the usual normal approximation.
Details
-
File Typepdf
-
Upload Time-
-
Content LanguagesEnglish
-
Upload UserAnonymous/Not logged-in
-
File Pages10 Page
-
File Size-