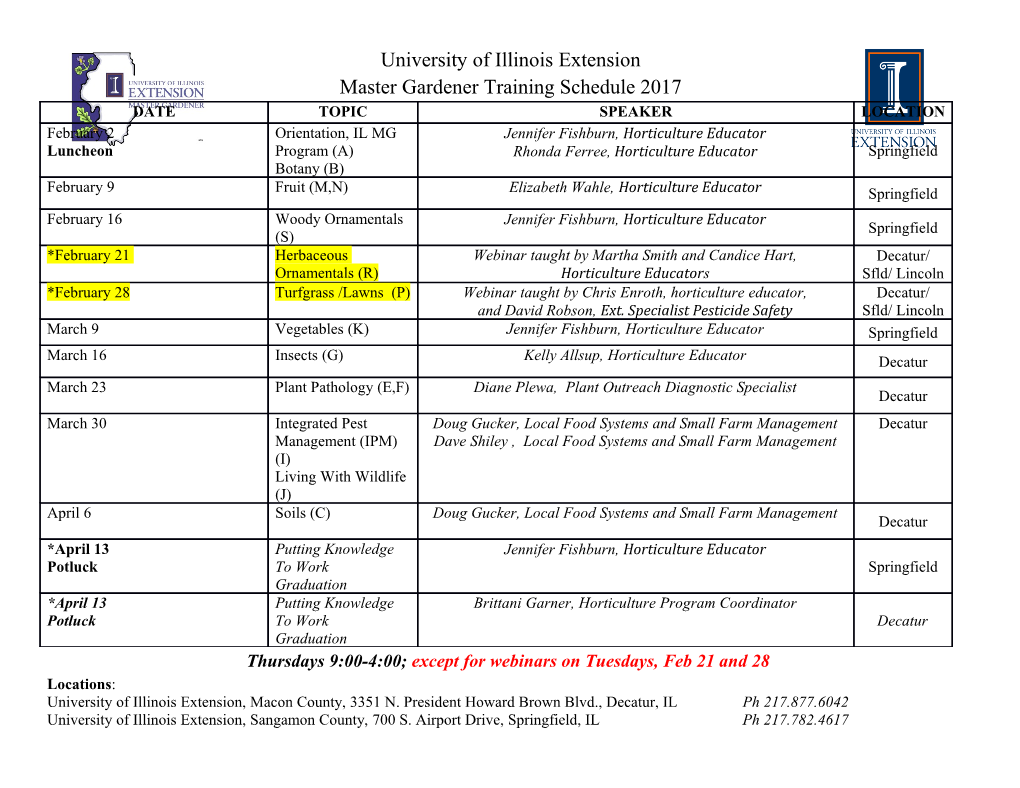
Genet. Res. Camb.(1997), 69, pp. 127–136. With 2 figures. Printed in the United Kingdom # 1997 Cambridge University Press 127 Model dependency of error thresholds: the role of fitness functions and contrasts between the finite and infinite sites models THOMAS WIEHE Department of Integratie Biology, Uniersity of California, Berkeley, CA 94720, USA (Receied 31 January 1996 and in reised form 26 August 1996 and 8 Noember 1996) Summary Based on a deterministic mutation–selection model the concept of error thresholds is critically examined. It has often been argued that genetic information – for instance, an advantageous allele – can be selectively maintained in a population only if the mutation rate is below a certain limit, the error threshold, which is inversely related to the genome size. Here, I will show that such an inverse relationship strongly depends on the fitness model. To produce the error threshold, as given by Eigen (1971), requires that the fitness model is an extreme form of diminishing epistasis. The error threshold, in a strict sense, vanishes as epistasis changes from diminishing to synergistic. In the latter case even the usual definition of error thresholds becomes ambiguous. Initially, a finite sites model has been used to describe error thresholds. However, they can also be defined within the framework of the infinite sites model. I study both models in parallel and compare their properties as far as error thresholds are concerned. It is concluded that error thresholds possibly play a much less important role in molecular evolution than has often been assumed in the past. rate there is a maximal evolutionarily permissible 1. Introduction genome size if genetic information (in the form of an A discussion about the evolutionary role of what was advantageous allele) is to be maintained in the later called error threshold was initiated in the early population. As a corollary, a given sequence length 1970s. Underlying this discussion is a model of defines a maximal permissible mutation rate, the error biochemical replicator systems, devised by Eigen threshold. Based on these relations, it was argued (1971) for a description of prebiotic evolutionary (Eigen & Schuster, 1979) that evolution at the early processes. A somewhat simplified version of this stages of life was confronted with a catch-22 situation model is equivalent to the deterministic population (no enzymes without a large genome and no large genetical mutation–selection equation (see Crow & genome without enzymes, which enhance the rep- Kimura, 1970, and references therein). The concept of lication accuracy), also named ‘information crisis’. error thresholds translates to the question of how In this article I revisit the deterministic mutation– large the mutation rate can be in order not to selection model and investigate the dependency of completely eliminate an advantageous allele from the error thresholds on assumptions such as the underlying population, when mutation and selection are balanced. fitness function or the finite (cf. Wright, 1949) versus For a finite sites model the answer may – among other infinite sites model (Kimura, 1969). Both turn out to quantities – depend on the genome size, measured by be crucial for the existence and magnitude of error the number of sites. Error thresholds are usually thresholds. associated with an inerse relationship between per Based on an infinite sites model and using discrete nucleotide mutation probability (p) and genome size time difference equations Wagner & Krall (1993) (ν). The formula given by Eigen (1971)is reported a condition under which no error threshold exists. However, their model is somewhat more log σ ν ¯ ,(1)restrictive than the one used here. It allows only for max p single-step mutations and considers merely fitness where σ is a so-called superiority parameter which functions which decrease monotonically as the number depends on the fitness model. The interpretation of of mutations increases. For the continuous time this equation is the following. For a given mutation model, I found results which are analogous to those of Downloaded from https://www.cambridge.org/core. IP address: 170.106.33.22, on 02 Oct 2021 at 02:25:45, subject to the Cambridge Core terms of use, available at https://www.cambridge.org/core/terms. https://doi.org/10.1017/S0016672397002619 T. Wiehe 128 the former authors. Further, I treated several quency of the wild-type becomes zero. Alternatively, generalizations of fitness functions for the finite and overall population statistics may be used, such as the infinite sites models in parallel. In particular, explicit average distance E of an allele from the wild-type (in threshold formulae are derived. Since one of the terms of number of mutations) or the index of important characteristics of error thresholds is the dispersion D, both defined below. limit they set on genome size (Nee & Maynard Smith, Mutation–selection balance had been an extensively 1990), an essential point is missed if the study of error discussed topic long before it attracted the attention thresholds and their dependence on fitness functions is of biochemically motivated research. Kimura & restricted to the infinite sites model. Maruyama (1966) investigated the effect of epistasis Here, I concentrate on the haploid version of the on the mutation load. Their interest has been in coupled (Hadeler, 1981) mutation–selection model. It quantifying the genetic load for different models of has been used previously in this context (e.g. Wiehe et selection and contrasting sexual and asexual rep- al., 1995). It describes the dynamics of a wild-type lication. They found that diminishing epistasis pro- allele and its variants, derived by mutation, for an duces a much higher mutational load than synergistic infinitely large population. In this model alleles are – epistasis, if reproduction is diploid. However, they did for simplicity – binary nucleotide sequences with a not directly compare the magnitudes of mutation common length ν %¢, but which may differ by point rates which are permissible for the different fitness mutations. Furthermore, it is assumed that reverse models so as not to stall natural selection. This is done mutations can be neglected, and that alleles which below and it is found that the kind of epistasis also differ from a particular type, called wild-type in the strongly influences the (haploid) error threshold. following, by the same number of point mutations have identical fitness. A fitness model which is often adopted in discussions The model of error thresholds (Swetina & Schuster, 1982; Nowak The fundamental mutation–selection equation, in the & Schuster, 1989; Wiehe et al., 1995) is the so-called form of coupled mutation and selection terms, is single-peaked fitness landscape: only the wild-type is distinguished by some fitness advantage. Any other ν yd k ¯ 3 yi i mki®yk a ,0%k%,ν%¢. (2) type, differing in as little as a single mutated site, is at i=! a disadvantage, expressed in its fitness 1®s. Such a two-class model was found to be adequate, for Here, yk denotes the frequency of the class of alleles instance, to describe evolution of the coliphage Qβ which differ from the wild-type (class 0) by exactly k (Domingo et al., 1978). Other examples discussed point mutations. k are their associated fitness values. ν (Eigen & Biebricher, 1988) in this context are the The mean fitness of the population is a ¯ 3i=o yi i. highly error-prone replication of viruses (Ortin et al., mki are entries of a mutation matrix M. They describe 1980; Martinez-Salas et al., 1985) or the serial transfer transitions from type i to type k. For the finite and the experiments of in itro replication of RNA (Spiegel- infinite sites models the mutation rates have to be man et al., 1965). However, concerns about the defined separately. In the former case, single sites biological adequacy of a single-peaked model and its mutate with probability p; in the latter, p has to be more general applicability, for instance to evolution substituted by a genome mutation rate, denoted λ. of higher organisms, have been raised (Maynard The probabilities mij are from a binomial distribution Smith, 1983; Charlesworth, 1990; A. S. Kondrashov, in the former case (see (3)). They are replaced by personal communication). Poisson mutation rates mW ij in the second case. To first- Although some consideration has been given by order accuracy, p and λ are related b λ ¯ νp. M has Eigen & Biebricher (1988) to the ‘fitness topography size (ν­1)¬(ν­1) and entries (cf. Higgs, 1994) of sequence space’, the dependency of error thresholds 1 ν®j i−j −i on this topography has not been investigated. In p (1®p)ν ,ifi&j m 2 0i®j1 (3) particular, one is left with the impression that any ij ¯ 3 fitness topography with a ‘superior master’ allele 4 0, if i ! j. (Eigen & Biebricher, 1988, p. 222) might produce an The Poisson approximation of Mq of M has entries error threshold which is inversely related to genome i−j λ 1 −λ size. e (i−j)!,ifi&j 2 It therefore still appears worthwhile to ask how mij ¯ 3 (4) 0, if i ! j. general error thresholds are and, if possible, to 4 quantify them in terms of the usual parameters. Matrices M and Mq are asymptotically equivalent (i.e. Despite numerous studies, there is no unambiguous or entries of M converge to the respective entries of Mq ) generally accepted definition of the term ‘error as ν becomes large and p small. When working threshold’. I will adhere to two characterizations without reverse mutation (see mij in (3)), this follows which are commonly used. One is to determine the immediately from the approximation of a binomial mutation parameter such that the equilibrium fre- distribution by a Poisson distribution.
Details
-
File Typepdf
-
Upload Time-
-
Content LanguagesEnglish
-
Upload UserAnonymous/Not logged-in
-
File Pages10 Page
-
File Size-