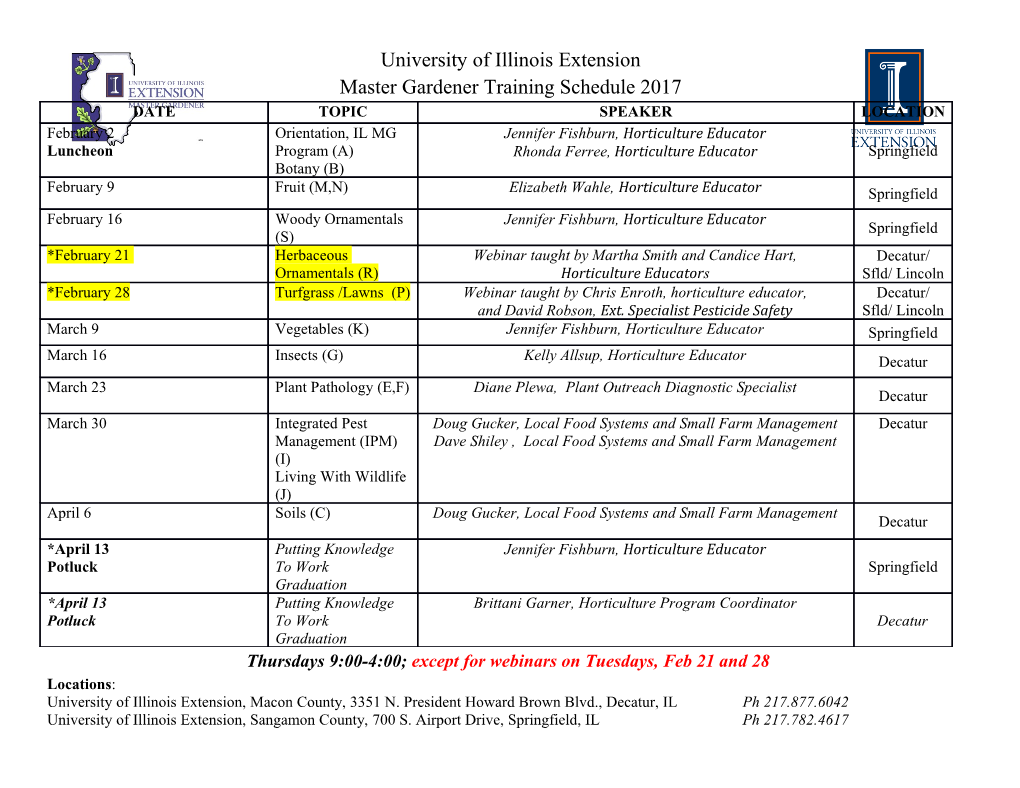
Kriging: An Introduction to Concepts and Applications Eric Krause Prahlad Jat Sessions of note… Tuesday • Interpolating Surfaces in ArcGIS (1:00-2:00 SDCC Rm33C) • Kriging: An Introduction to Concepts and Applications (2:30-3:30 SDCC Rm33C) • Geostatistical Analyst: An Introduction (4:00-5:00 SDCC Rm30C) Wednesday • Surface Interpolation in ArcGIS (11:15-12:00 SDCC Demo Theater 10) • Empirical Bayesian Kriging and EBK Regression Prediction in ArcGIS (2:30-3:15 SDCC Demo Theater 10) Thursday • Geostatistics in Practice: Learning Interpolation Through Examples (8:30-9:30 SDCC Rm30A) • Polygon-to-Polygon Predictions Using Areal Interpolation (11:15-12:00 SDCC Demo Theater 10) • Geostatistical Analyst: An Introduction (1:00-2:00 SDCC Rm30A) • Using Living Atlas Data and ArcGIS Pro for 3D Interpolation (2:30-3:30 SDCC Rm 30C) • Interpolating Surfaces in ArcGIS (4:00-5:00 SDCC Rm15A) • Kriging: An Introduction to Concepts and Applications (4:00-5:00 SDCC Rm15B) 2 Geostatistical Analyst Resources http://esriurl.com/GeostatGetStarted • GeoNet – community.esri.com - Blogs - Free textbook and datasets - Spatial Statistical Analysis For GIS Users - Lots of discussions and Q&A • Learn GIS – learn.arcgis.com - Model Water Quality Using Interpolation - Analyze Urban Heat Using Kriging - Interpolate 3D Oxygen Measurements in Monterey Bay Outline • Introduction to interpolation • Introduction to kriging • Validating interpolation results • Empirical Bayesian Kriging and EBK Regression Prediction • Empirical Bayesian Kriging 3D • Areal Interpolation • Questions What is interpolation? • Predict values at unknown locations using values at measured locations • Many interpolation methods: kriging, IDW, LPI, etc What is autocorrelation? Tobler’s first law of geography: "Everything is related to everything else, but near things are more related than distant things." Building up interpolation • Given the data on the right, how would you predict the value of the red point? • Simplest answer: guess the average of all the points: 49 - Ignores spatial information Building up interpolation • Next best guess: average of a local neighborhood • Average of points within 12km buffer: 40.75 - Better, but points that are closer should have more influence Building up interpolation • Next best guess: Weight points by inverse distance • Weighted average of points within 12km buffer: 41.01 - This seems like a reasonable guess with no obvious problems Building up interpolation • What about this point? • More points below than above Building up interpolation • What about this point? • More points below than above • Inverse-distance weighted average in 40km buffer: 49.8 Building up interpolation • What about this point? • More points below than above • Kriging prediction: 56.2 - Some weights are even negative Semivariogram What is kriging? • Kriging is the optimal interpolation method if the data meets certain conditions. • What are these conditions? - Normally distributed - Stationary - No trends • How do I check these conditions? - Exploratory Analysis and charting What is an “optimal” interpolator? • Estimates the true value, on average • Lowest expected prediction error • Able to use extra information, such as covariates • Can be generalized to polygons (Areal interpolation, Geostatistical simulations) • Estimates a prediction distribution, not just a single prediction Kriging output surface types Prediction Error of Predictions Probability Quantile Kriging workflow 1. Explore the data – check kriging assumptions 2. Configure options: trend removal, transformations, etc 3. Estimate a semivariogram model 4. Validate the results 5. Repeat steps 2-4 as necessary 6. Map the data for decision-making Does my data follow a normal distribution? • Histogram chart - Symmetric and bell-shaped - Look for outliers - Mean ≈ Median • What can I do if my data is not normally distributed? - Apply a transformation - Log, Box Cox, Arcsin, Normal Score Transformation Why the normal distribution is important • Kriging predicts mean and standard error at every location Why the normal distribution is important • Kriging predicts mean and standard error at every location Skewed distributions and outliers Skewed Distribution Outlier Assessing models using cross validation • Used to determine the reliability of the model - Iteratively discard each sample - Use remaining points to estimate value at measured location - Compare predicted versus measured value • Calculates various statistics - Root-mean-square : root of average squared deviation from true value - Smaller is better - Mean : the average of the deviations - Should be close to zero - Root-mean-square standardized : measures whether standard errors are estimated correctly - Should be close to one - Average standard error : should be small and close to the root-mean-square Kriging in the Geostatistical Wizard Demo Empirical Bayesian Kriging • Advantages - Requires minimal interactive modeling, spatial relationships are modeled automatically - Usually more accurate, especially for small or nonstationary datasets - Uses local models to capture small scale effects - Doesn’t assume one model fits the entire data - Standard errors of prediction are more accurate than other kriging methods • Disadvantages - Processing is slower than other kriging methods - Limited customization How does EBK work? 1. Divide the data into subsets of a given size - Controlled by “Subset Size” parameter - Subsets can overlap, controlled by “Overlap Factor” 2. For each subset, estimate the semivariogram 3. Simulate data at input point locations and estimate new semivariogram 4. Repeat step 3 many times. This results in a distribution of semivariograms 5. Mix the local surfaces together to get the final surface. EBK Regression Prediction • Allows you to use explanatory variable rasters to improve predictions • Automatically extracts useful information from explanatory variables • Uses Principle Components to handle multicollinearity EBK and EBK Regression Prediction Demo Empirical Bayesian Kriging 3D • New in ArcGIS Pro 2.3 • Interpolate points measured in 3D using EBK • Visualize 2D transects using Range Slider • Export to rasters, contours, and 3D points EBK 3D Demo Areal Interpolation Obesity by school zone Obesity surface and Obesity by census block error surface • Predict data in a different geometry - School zones to census tracts • Estimate values for missing data Areal Interpolation Workflow .
Details
-
File Typepdf
-
Upload Time-
-
Content LanguagesEnglish
-
Upload UserAnonymous/Not logged-in
-
File Pages32 Page
-
File Size-