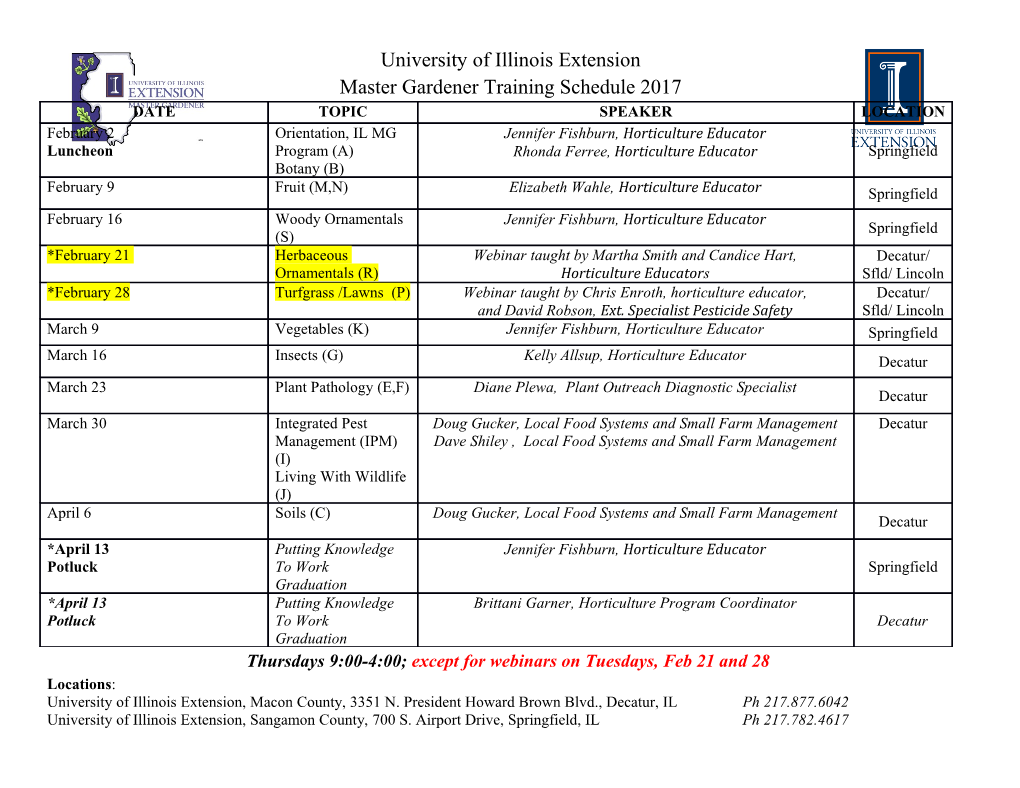
Ann Inst Stat Math (2018) 70:421–438 https://doi.org/10.1007/s10463-016-0592-7 An information criterion for model selection with missing data via complete-data divergence Hidetoshi Shimodaira1,2 · Haruyoshi Maeda1,3 Received: 26 September 2015 / Revised: 4 November 2016 / Published online: 21 January 2017 © The Institute of Statistical Mathematics, Tokyo 2017 Abstract We derive an information criterion to select a parametric model of complete- data distribution when only incomplete or partially observed data are available. Compared with AIC, our new criterion has an additional penalty term for missing data, which is expressed by the Fisher information matrices of complete data and incomplete data. We prove that our criterion is an asymptotically unbiased estima- tor of complete-data divergence, namely the expected Kullback–Leibler divergence between the true distribution and the estimated distribution for complete data, whereas AIC is that for the incomplete data. The additional penalty term of our criterion for missing data turns out to be only half the value of that in previously proposed infor- mation criteria PDIO and AICcd. The difference in the penalty term is attributed to the fact that our criterion is derived under a weaker assumption. A simulation study with the weaker assumption shows that our criterion is unbiased while the other two criteria are biased. In addition, we review the geometrical view of alternating minimizations of the EM algorithm. This geometrical view plays an important role in deriving our new criterion. The research was supported in part by JSPS KAKENHI Grant (24300106, 16H02789). B Hidetoshi Shimodaira [email protected] 1 Division of Mathematical Science, Graduate School of Engineering Science, Osaka University, 1-3 Machikaneyama-cho, Toyonaka, Osaka 560-8531, Japan 2 RIKEN Center for Advanced Intelligence Project, 1-4-1 Nihonbashi, Chuo-ku, Tokyo 103-0027, Japan 3 Present Address: Kawasaki Heavy Industries, Ltd., 1-1 Kawasaki-cho, Akashi, Hyogo 673-8666, Japan 123 422 H. Shimodaira, H. Maeda Keywords Akaike information criterion · Alternating projections · Data manifold · EM algorithm · Fisher information matrix · Incomplete data · Kullback–Leibler divergence · Misspecification · Takeuchi information criterion 1 Introduction Modeling complete data X = (Y, Z) is often preferable to modeling incomplete or partially observed data Y when missing data Z are not observed. The expectation- maximization (EM) algorithm (Dempster et al. 1977) computes the maximum likelihood estimate of parameter vector θ for a parametric model of the probabil- ity distribution of X. In this research, we consider the problem of model selection in such situations. For mathematical simplicity, we assume that X consists of independent and identically distributed random vectors. More specifically, X = (x1, x2,...,xn), and the complete-data distribution is modeled as x1, x2,...,xn ∼ px (x; θ). Each vector is decomposed as xT = ( yT , zT ), and the marginal distribution is expressed as py( y; θ) = px ( y, z; θ) d z, where T denotes the matrix transpose and the integration is over all possible values of z. We formally treat y, z as continuous random variables with the joint density function px . However, when they are discrete random variables, the integration should be replaced with a summation of the probability functions. We use symbols such as px and py for both the continuous and discrete cases, and simply refer to them as distributions. (θ) = n ( ; θ) The log-likelihood function is y t=1 log py yt with the parameter T d vector θ = (θ1,...,θd ) ∈ R . We assume that the model is identifiable, and the parameter is restricted to ⊂ Rd . Then, the maximum likelihood estimator (MLE) ˆ ˆ of θ is defined by θ y = arg max y(θ). The dependence of y(θ) and θ y on Y = θ∈ ( ,..., ) y1 yn is suppressed in the notation. Akaike (1974) proposed the information criterion ˆ AIC =−2y(θ y) + 2d for model selection. The first term measures the goodness of fit, whereas the second term is interpreted as a penalty for model complexity. The AIC values for candidate models are computed, and then the model that minimizes AIC is selected. This information criterion estimates the expected discrepancy between the unknown true distribution of y, which is denoted as qy, and the estimated distribu- ˆ tion py(θ y). This discrepancy is measured by the incomplete-data Kullback–Leibler divergence. In this study, we work on the complete-data Kullback–Leibler divergence instead of the incomplete-data counterpart. An information criterion to estimate the expected discrepancy between the unknown true distribution of x, which is denoted as qx , and the ˆ estimated distribution px (θ y) is derived. This approach makes sense when modeling complete data more precisely describes the part being examined. Similar attempts are found in the literature. Shimodaira (1994) proposed the information criterion PDIO (predictive divergence for incomplete observation models) 123.
Details
-
File Typepdf
-
Upload Time-
-
Content LanguagesEnglish
-
Upload UserAnonymous/Not logged-in
-
File Pages2 Page
-
File Size-