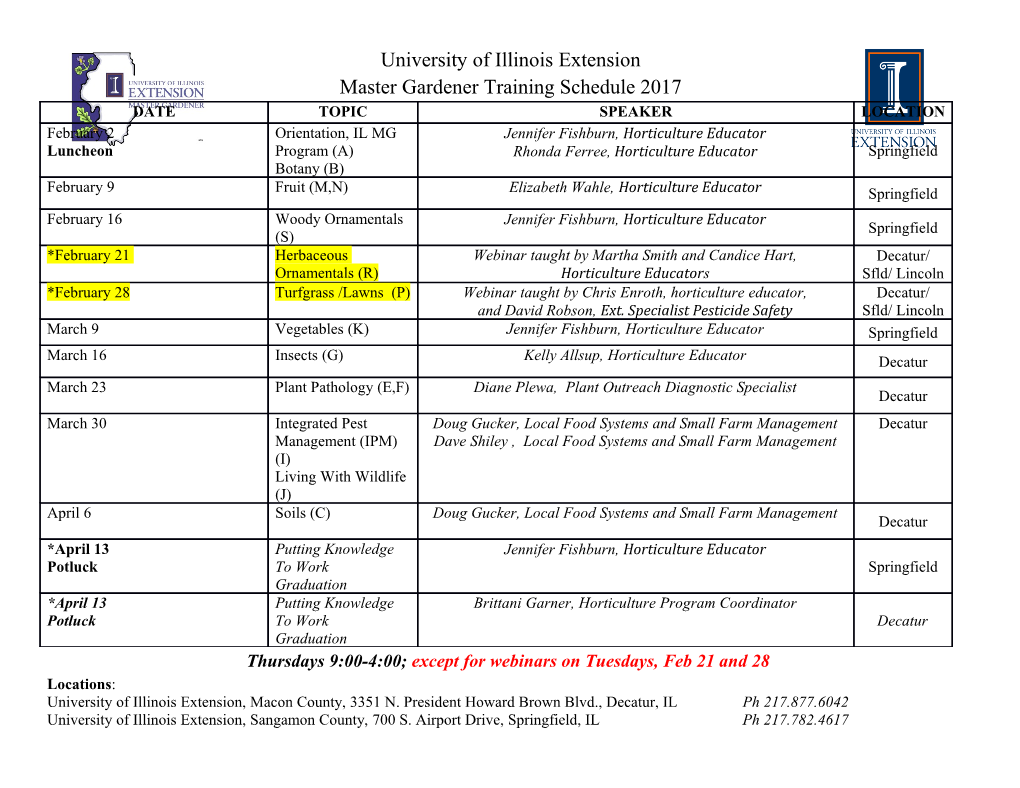
DEGREE PROJECT IN MATHEMATICS, SECOND CYCLE, 30 CREDITS STOCKHOLM, SWEDEN 2020 Capturing Tail Risk in a Risk Budgeting Model FILIP LUNDIN MARKUS WAHLGREN KTH ROYAL INSTITUTE OF TECHNOLOGY SCHOOL OF ENGINEERING SCIENCES Capturing Tail Risk in a Risk Budgeting Model FILIP LUNDIN MARKUS WAHLGREN Degree Projects in Financial Mathematics (30 ECTS credits) Master's Programme in Industrial Engineering and Management KTH Royal Institute of Technology year 2020 Supervisor at Nordnet AB: Gustaf Haag Supervisor at KTH: Anja Janssen Examiner at KTH: Anja Janssen TRITA-SCI-GRU 2020:050 MAT-E 2020:016 Royal Institute of Technology School of Engineering Sciences KTH SCI SE-100 44 Stockholm, Sweden URL: www.kth.se/sci Abstract Risk budgeting, in contrast to conventional portfolio management strategies, is all about distributing the risk between holdings in a portfolio. The risk in risk budgeting is traditionally measured in terms of volatility and a Gaussian distribution is commonly utilized for modeling return data. In this thesis, these conventions are challenged by introducing different risk measures, focusing on tail risk, and other probability distributions for modeling returns. Two models for forming risk budgeting portfolios that acknowledge tail risk were chosen. Both these models were based on CVaR as a risk measure, in line with what previous researchers have used. The first model modeled re- turns with their empirical distribution and the second with a Gaussian mixture model. The performance of these models was thereafter evaluated. Here, a diverse set of asset classes, several risk budgets, and risk targets were used to form portfolios. Based on the performance, measured in risk-adjusted returns, it was clear that the models that took tail risk into account in general had su- perior performance in relation to the standard model. Nevertheless, it should be noted that the superiority was significantly higher for portfolios that consti- tuted of mainly high-risk assets than for portfolios with more low-risk assets and also that the superior performance did not hold in all time periods con- sidered. It was also clear that the model that used the empirical distribution to model returns performed better than the model based on an assumption of returns belonging to the Gaussian mixture model when the portfolio consisted of more assets with heavier tails. Filip Lundin i Markus Wahlgren En riskbudgeteringsmodell som tar hän- syn till svansrisk Sammanfattning Jämfört med konventionella portföljhanteringsstrategier handlar riskbudgete- ring mer om att fördela risken mellan innehav i en portfölj. Risken i riskbud- getering mäts traditionellt med avseende på volatilitet och en Gaussisk för- delning används normalt för att modellera avkastningsdata. I den här avhand- lingen anlyseras andra modeller som istället fokuserar på svansrisk genom att införa andra riskmått och genom att använda andra sannolikhetsfördelningar för modellering av avkastningsdata. Två modeller för att konstruera riskbudgeteringsportföljer som tar hänsyn till svansrisk har analyserats i den här avhandlingen. Båda dessa modeller använ- de sig av CVaR som ett riskmått, i linje med vad tidigare forskare har använt. Den första modellen modellerade avkastningar med den empiriska fördelning- en och den andra modellen med en Gaussisk blandningsmodell. Därefter ut- värderades hur de olika modellerna presterade. Här användes en mångfald av tillgångsklasser, flera riskbudgetar och riskmål för att bilda portföljerna. Ba- serat på prestanda, mätt i termer av riskjusterad avkastning, var det tydligt att de modeller som tog hänsyn till svansrisk generellt presterade bättre än den konventionella modellen. Det bör emellertid noteras att för portföljer som hu- vudsakligen bestod av tillgångar med låg risk så var detta resultat mindre signi- fikant och även att resultatet inte gällde för alla tidsperioder som analyserades. Det var också tydligt att modellen som använde den empiriska fördelningen för att modellera avkastningsdata fungerade bättre än den Gaussiska bland- ningsmodellen när portföljen till större del bestod av tillgångar med tyngre svansar. Filip Lundin ii Markus Wahlgren Acknowledgements We want to thank Nordnet for presenting us with the opportunity to write this thesis and for providing the necessary data. We especially want to thank our supervisor at Nordnet, Gustaf Haag, for introducing us to the subject of risk budgeting and for helping us to pinpoint a subject to write about. Also, we want to thank our supervisor at KTH Royal Institute of Technology, Anja Janssen for the helpful guidance and feedback that we have received throughout the process of writing this thesis. Filip Lundin & Markus Wahlgren Stockholm, May 13, 2020 Filip Lundin iii Markus Wahlgren Contents 1 Introduction 1 1.1 Research Questions . .2 1.2 Scope & Limitations . .3 1.3 Related Work . .3 1.4 Outline . .6 2 Background 7 2.1 Distributions . .7 2.1.1 Gaussian Distribution . .7 2.1.2 Gaussian Mixture Distribution . .8 2.1.3 Empirical Distribution . .9 2.2 Parameter Estimation . 10 2.2.1 Gaussian Distribution . 10 2.2.2 Maximum Likelihood . 10 2.2.3 Gaussian Mixture Model . 11 2.3 Risk Measures . 14 2.3.1 Coherent Risk Measures . 14 2.3.2 Volatility . 15 2.3.3 Value-at-Risk . 16 2.3.4 Conditional Value-at-Risk . 17 2.4 Risk Budget Portfolios . 18 2.4.1 Existence and Uniqueness of the Portfolio . 19 2.4.2 Euler’s Theorem on Homogeneous Functions . 19 2.4.3 Volatility as a Risk Measure . 20 2.4.4 CVaR as a Risk Measure . 21 2.4.5 CVaR as a Risk Measure with Gaussian Mixture Model 23 2.5 Optimization . 28 2.5.1 Optimization Problem . 28 2.5.2 Quadratic Programming . 29 iv 2.5.3 Sequential Quadratic Programming . 29 2.6 Risk Targeting . 29 2.7 Performance Measures . 31 2.7.1 Sharpe Ratio . 31 2.7.2 Other Measures of Risk-Adjusted Returns . 31 2.7.3 Maximum Drawdown . 32 3 Data and Methodology 33 3.1 Data . 33 3.2 Risk Budgeting Portfolio Creation . 37 3.2.1 Portfolios with Leverage . 38 3.3 Portfolio Evaluation . 38 4 Results 40 4.1 Performance Graphs . 40 4.2 Performance Measures for the Full Period . 46 4.3 Performance Measures for Sub-Periods . 48 5 Discussion 52 5.1 Evaluation of the Findings . 52 5.2 Sources of Error . 55 5.3 Future Work . 56 6 Conclusions 57 7 Bibliography 59 A Model data 62 A.1 Capital Weights . 62 A.2 QQ-Plots for the Models . 68 A.3 Leverage . 70 A.4 Performance Measures for Sub-Periods . 73 List of Figures 2.1 Illustration of VaR for a density function fL of losses . 17 2.2 Illustration of CVaR for a density function fL of losses . 18 2.3 Illustration of maximum drawdown . 32 4.1 Performance for unleveraged risk parity portfolios . 41 4.2 Performance for low-risk portfolios for a daily empirical CVaR0:05 target of 0.5% . 43 4.3 Performance for mid-risk portfolios for a daily empirical CVaR0:05 target of1% ................................... 44 4.4 Performance for high-risk portfolios for a daily empirical CVaR0:05 tar- get of 1.5% . 45 4.5 The Sharpe ratio for the different periods for the risk parity portfolio . 48 4.6 The Tail risk-adjusted return for the different periods for the risk parity portfolio . 49 4.7 The Sharpe ratio for the different periods for the high-risk portfolio . 50 4.8 The Tail risk-adjusted return for the different periods for the high-risk portfolio . 50 vi List of Tables 3.1 Portfolio risk budgets . 34 3.2 The daily empirical CVaR0:05 target for the different risk levels . 34 3.3 Performance measures for individual assets . 36 4.1 Performance measures for risk parity portfolio . 46 4.2 Performance measures for low-risk portfolio . 46 4.3 Performance measures for mid-risk portfolio . 47 4.4 Performance measures for high-risk portfolio . 47 vii Chapter 1 Introduction Historically, portfolio allocation strategies have often involved investing 60 % in equities and 40 % in bonds (60/40-portfolio) or applying Markowitz’s [1] mean-variance analysis to determine portfolio weights. The mean-variance analysis has allowed investors to find the optimal capital allocation that maximizes the expected return for a given volatility level or minimize the volatility for a given level of expected return. Although both these strategies have been very popular, some criticism has been directed against them. For instance, the 60/40-portfolio is said to not diversify risks properly and the mean-variance method is highly sensitive to the input parameters. In the past decades, alternative ap- proaches for portfolio construction have therefore gained traction in the investment com- munity, one of them being risk budgeting. Risk budgeting, in contrast to the previously discussed allocation strategies, is all about distributing the risk between holdings in a portfolio. The risk in risk budgeting is often measured by volatility, which is a com- monly used measure of risk in finance. A special case of risk budgeting is risk parity or equal risk contribution (ERC) where the risk is allocated equally between assets. The first risk budgeting fund, called All-Weather, was developed by Bridgewater asso- ciates in 1996 and used a risk parity approach to establish an investment strategy that would perform well in all economic conditions [2]. Since then and particularly after the financial crisis of 2008, when numerous institutional investors understood that their current models yielded riskier portfolios than expected, the popularity of risk budgeting and risk parity has increased considerably [3]. Risk budgeting as an investment strategy has been praised for its ability to generate true diversification considering risks in portfolios. In for instance the 60/40-portfolio 90 % of the portfolio risk, measured in volatility, normally originates from the equity component since equities are significantly riskier than bonds [4].
Details
-
File Typepdf
-
Upload Time-
-
Content LanguagesEnglish
-
Upload UserAnonymous/Not logged-in
-
File Pages94 Page
-
File Size-