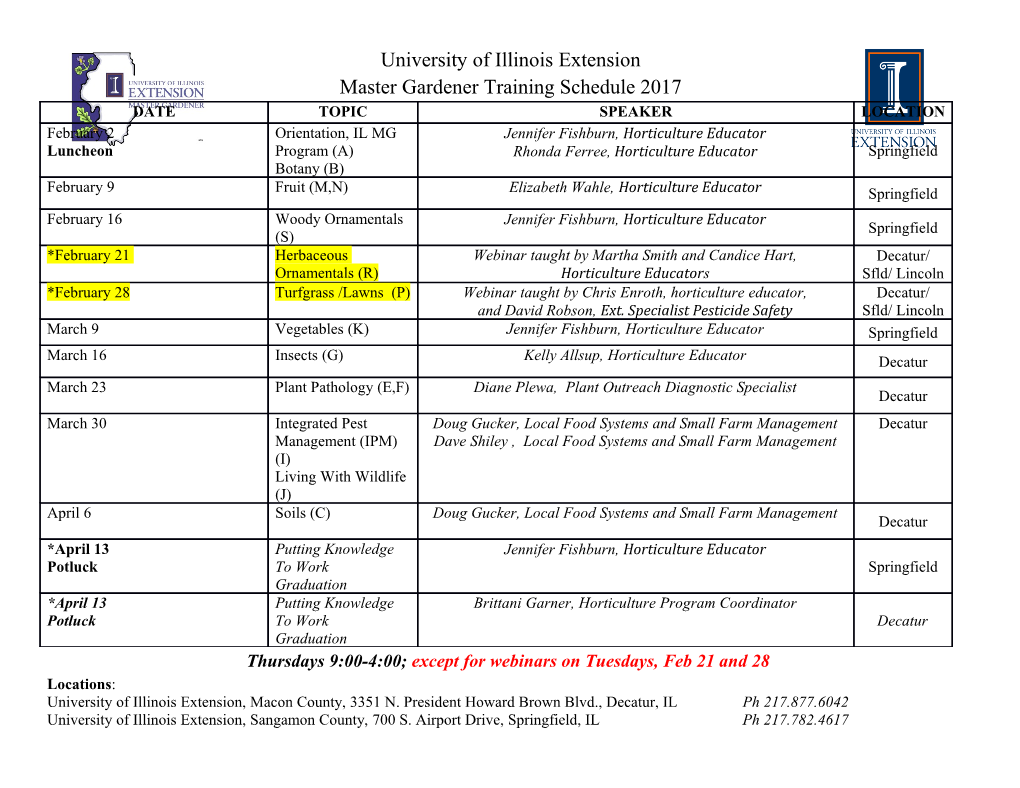
CSE 613: Parallel Programming Lecture 2 ( Analytical Modeling of Parallel Algorithms ) Rezaul A. Chowdhury Department of Computer Science SUNY Stony Brook Spring 2019 Parallel Execution Time & Overhead et al., et Edition Grama nd 2 Source: “Introduction to Parallel Computing”, to Parallel “Introduction Parallel running time on 푝 processing elements, 푇푃 = 푡푒푛푑 – 푡푠푡푎푟푡 , where, 푡푠푡푎푟푡 = starting time of the processing element that starts first 푡푒푛푑 = termination time of the processing element that finishes last Parallel Execution Time & Overhead et al., et Edition Grama nd 2 Source: “Introduction to Parallel Computing”, to Parallel “Introduction Sources of overhead ( w.r.t. serial execution ) ― Interprocess interaction ― Interact and communicate data ( e.g., intermediate results ) ― Idling ― Due to load imbalance, synchronization, presence of serial computation, etc. ― Excess computation ― Fastest serial algorithm may be difficult/impossible to parallelize Parallel Execution Time & Overhead et al., et Edition Grama nd 2 Source: “Introduction to Parallel Computing”, to Parallel “Introduction Overhead function or total parallel overhead, 푇푂 = 푝푇푝 – 푇 , where, 푝 = number of processing elements 푇 = time spent doing useful work ( often execution time of the fastest serial algorithm ) Speedup Let 푇푝 = running time using 푝 identical processing elements 푇1 Speedup, 푆푝 = 푇푝 Theoretically, 푆푝 ≤ 푝 ( why? ) Perfect or linear or ideal speedup if 푆푝 = 푝 Speedup Consider adding 푛 numbers using 푛 identical processing Edition nd elements. Serial runtime, 푇1 = 푛 Parallel runtime, 푇푛= log 푛 푇1 푛 Speedup, 푆푛= = 푇푛 log 푛 et al., “Introduction to Parallel Computing”, 2 Computing”, to Parallel al., “Introduction et Speedup not ideal. Grama Source: Superlinear Speedup Theoretically, 푆푝 ≤ 푝 But in practice superlinear speedup is sometimes observed, that is, 푆푝 > 푝 ( why? ) Reasons for superlinear speedup ― Cache effects ― Exploratory decomposition Superlinear Speedup ( Cache Effects ) Let cache access latency = 2 ns DRAM DRAM access latency = 100 ns cache cache Suppose we want solve a problem instance that executes k FLOPs. CPU CPU With 1 Core: Suppose cache hit rate is 80%. core core If the computation performs 1 FLOP/memory access, then each FLOP will take 2 × 0.8 + 100 × 0.2 = 21.6 ns to execute. With 2 Cores: Cache hit rate will improve. ( why? ) Suppose cache hit rate is now 90%. Then each FLOP will take 2 × 0.9 + 100 × 0.1 = 11.8 ns to execute. Since now each core will execute only k / 2 FLOPs, 푘×21.6 푆 = 3.66 > 2 Speedup, 2 (푘/2)×11.8 Superlinear Speedup ( Due to Exploratory Decomposition ) Consider searching an array of 2n unordered elements for a specific element x. Suppose x is located at array location k > n and k is odd. Serial runtime, 푇 = 푘 A[1] A[2] A[3] … … … A[k] … … … A[2n] 1 x Parallel running time with n sequential search processing elements, 푇푛 = 1 푇 1 A[1] A[2] A[3] … … … A[k] … … … A[2n] Speedup, 푆푛 = = 푘 > 푛 푇푛 x P1 P2 … … … P … … … Pn Speedup is superlinear! 풌/ퟐ parallel search Parallelism & Span Law We defined, 푇푝 = runtime on 푝 identical processing elements Then span, 푇∞ = runtime on an infinite number of identical processing elements 푇 Parallelism, 푃 = 1 푇∞ Parallelism is an upper bound on speedup, i.e., 푆푝 ≤ 푃 ( why? ) Span Law 푇푝 ≥ 푇∞ Work Law The cost of solving ( or work performed for solving ) a problem: On a Serial Computer: is given by 푇1 On a Parallel Computer: is given by 푝푇푝 Work Law 푇 푇 ≥ 1 푝 푝 Work Optimality Let 푇푠 = runtime of the optimal or the fastest known serial algorithm A parallel algorithm is cost-optimal or work-optimal provided 푝푇푝 = 푇푠 Our algorithm for adding 푛 numbers using 푛 identical processing elements is clearly not work optimal. Adding n Numbers Work-Optimality We reduce the number of processing elements which in turn increases the granularity of the subproblem assigned to each processing element. Suppose we use 푝 processing elements. First each processing element locally Source: Grama et al., “Introduction to Parallel Computing”, 2nd Edition 푛 푛 adds its numbers in time . 푝 푝 Then 푝 processing elements adds these 푝 partial sums in time log 푝 . 푛 Thus 푇 = + log 푝 , and 푇 = 푛 . 푝 푝 푠 So the algorithm is work-optimal provided 푛 = 푝 log 푝 . Scaling Laws Scaling of Parallel Algorithms ( Amdahl’s Law ) Suppose only a fraction f of a computation can be parallelized. 푇 Then parallel running time, 푇 ≥ 1 − 푓 푇 + 푓 1 푝 1 푝 푇1 푝 1 1 Speedup, 푆푝 = ≤ = 푓 ≤ 푇푝 푓+ 1−푓 푝 1−푓 + 1−푓 푝 Scaling of Parallel Algorithms ( Amdahl’s Law ) Suppose only a fraction f of a computation can be parallelized. 푇1 1 1 Speedup, 푆푝 = ≤ 푓 ≤ 푇푝 1−푓 + 1−푓 푝 Source: Wikipedia Scaling of Parallel Algorithms ( Gustafson-Barsis’ Law ) Suppose only a fraction f of a computation was parallelized. Then serial running time, 푇1 = 1 − 푓 푇푝 + 푝푓푇푝 푇1 1−푓 푇푝+푝푓푇푝 Speedup, 푆푝 = = = 1 + 푝 − 1 푓 푇푝 푇푝 Scaling of Parallel Algorithms ( Gustafson-Barsis’ Law ) Suppose only a fraction f of a computation was parallelized. 푇 푇1 1−푓 푇푝+푝푓푇푝 Speedup, 푆푝 = ≤ = = 1 + 푝 − 1 푓 푇푝 푇푝 푇푝 f = 0.9 f = 0.8 f = 0.7 f = 0.6 f = 0.5 f = 0.4 f = 0.3 Speedup f = 0.2 f = 0.1 Number of Processors Source: Wikipedia Strong Scaling vs. Weak Scaling Martha Kim, Columbia University Kim, ColumbiaMartha Source: Strong Scaling How 푇푝 ( or 푆푝 ) varies with 푝 when the problem size is fixed. Weak Scaling How 푇푝 ( or 푆푝 ) varies with 푝 when the problem size per processing element is fixed. Scalable Parallel Algorithms 푆푝 푇1 Efficiency, 퐸푝 = = 푝 푝푇푝 et al., et Edition Grama nd 2 Source: “Introduction to Parallel Computing”, to Parallel “Introduction A parallel algorithm is called scalable if its efficiency can be maintained at a fixed value by simultaneously increasing the number of processing elements and the problem size. Scalability reflects a parallel algorithm’s ability to utilize increasing processing elements effectively. Scalable Parallel Algorithms In order to keep 퐸푝 fixed at a constant k, we need 푇1 퐸푝 = 푘 = 푘 푇1 = 푘푝푇푝 푝푇푝 For the algorithm that adds n numbers using p processing elements: 푛 푇 = 푛 and 푇 = + 2 log 푝 1 푝 푝 So in order to keep 퐸푝 fixed at k, we must have: 푛 2푘 푛 = 푘푝 + 2 log 푝 푛 = 푝 log 푝 푝 1 − 푘 Fig: Efficiency for adding n numbers using p processing elements Source: Grama et al., “Introduction to Parallel Computing”, 2nd Edition.
Details
-
File Typepdf
-
Upload Time-
-
Content LanguagesEnglish
-
Upload UserAnonymous/Not logged-in
-
File Pages21 Page
-
File Size-