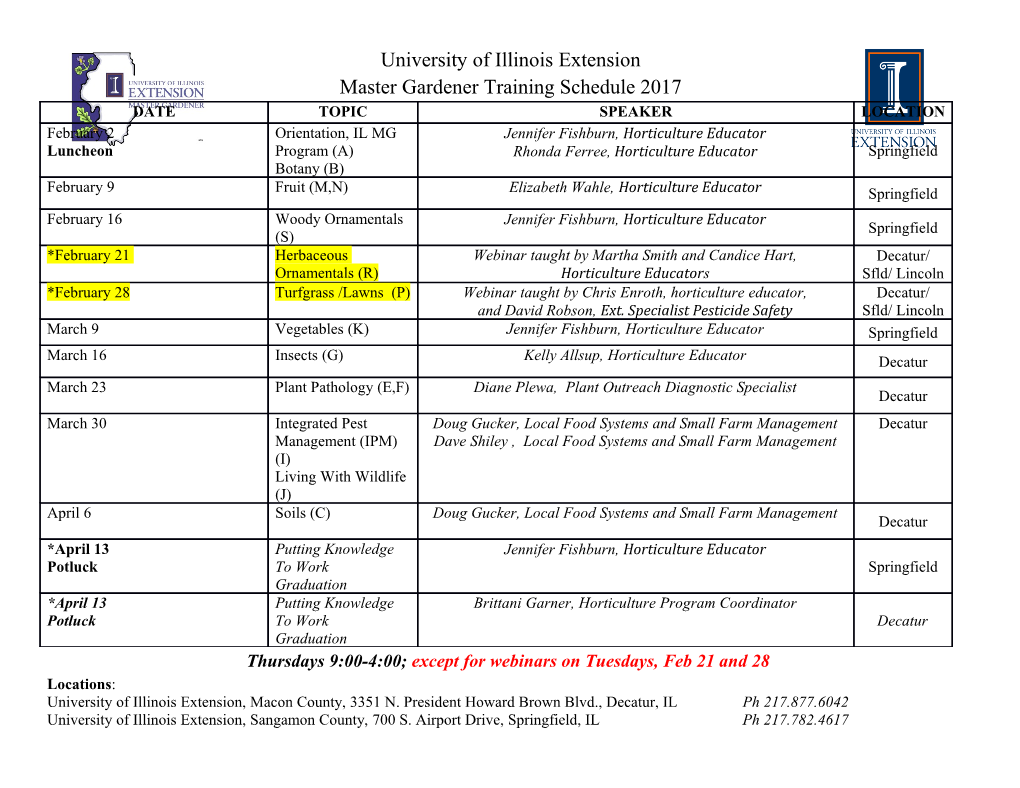
OPTIMAL TRANSPORT AND CONCENTRATION OF MEASURE NATHAEL GOZLAN*, TRANSCRIBED BY BARTLOMIEJPOLACZYK Abstract. This is a preliminary version of the notes of the lectures on the optimal trans- port and concentration of measure that were given during the 2nd Warsaw Summer School in Probability on July 2017. We introduce here the notion of concentration of measure and develop its connections with some functional inequalities via the methods of optimal trans- port theory. At the end we turn to the convex concentration property and weak transport costs. Any comments or corrections are welcome. In case of such, please contact notes' author at [email protected]. 1. Concentration of measure phenomenon 1.1. Preliminaries. Concentration of measure phenomenon states in general that a random variable that depends on many i.i.d. variables via some Lipschitz function is essentially concentrated around its mean. This phenomenon has many consequences in various fields of probability theory or statistics. Throughout the notes we will denote by (X; d; µ) an abstract metric space equipped with a distance d and a probability measure µ on some sigma algebra Σ. Moreover, we set P(X) to be the set of all probability measures on X. We will start with the core definition of this lecture. Definition. Let α : [0; +1) ! [0; 1] be non increasing. We say that a probability measure µ 2 P(X) satisfies concentration of measure property with the concentration profile α if 1 µ(A) ≥ ) µ(A ) ≥ 1 − α(t) 8 8 ; 2 t A⊆X t≥0 where At := fx 2 X : d(x; A) ≤ tg. We will denote this fact by µ 2 Cα(X; d). Example. Some popular profiles are of the form k αk(t) = a exp −b(t − t0)+ ; for k 2 N; a; b; t0 2 R. Setting k = 1; 2 results in exponential and Gaussian profile respectively. Proposition 1.1. The following statements are equivalent: 1. µ 2 Cα(X; d), 2. µ(f > mµ(f) + t) ≤ α(t) 8f2Lip1 (X; d). Here 1 mµ(f) := arg inf µ(f ≥ t) ≤ t2R 2 *Universit Paris Descartes; [email protected]. 1 2 NATHAEL GOZLAN is a median of f w.r.t. µ and Lipk(X; d) is a space of all k-Lipschitz functions on X w.r.t. the metric d. In general will write Lipk if there is no confusion about the underlying space and the metric. Proof. (1: ) 2:): 1 Set A := ff ≤ mµ(f)g for some arbitrary function f 2 Lip1. Clearly µ(A) ≥ 2 and using the fact that A is closed At = fx : d(x; A) ≤ tg = fx : 9y2X : d(x; y) ≤ t ^ f(y) ≤ mµ(f)g ⊆ ff ≤ mµ(f) + tg; whence µ(f > mµ(f) + t) ≤ 1 − µ(At) ≤ α(t): (2: ) 1:): 1 Let A ⊆ X such that µ(A) ≥ 2 and set f(x) = d(x; A). Whence, f 2 Lip1 by the triangle inequality and mµ(f) = 0, thus µ(At) = µ(f ≤ t) = 1 − µ(f > mµ(f) + t) ≥ 1 − α(t): Remark 1.2. From the proof of Proposition 1.1 it follows that f has to be Lipschitz only in the support of µ. Remark 1.3. If µ 2 Cα(X; d) then applying the above proposition for −f results in the following inequality (1) µ(jf − mµ(f)j > t) ≤ 2α(t); which holds for any f 2 Lip1. Depending on the situation, it can sometimes be easier to work with a mean instead of a median. Fortunately, concentration inequalities involving the median are, up to the constant, equivalent to the ones with the mean which illustrates the following remark. Remark 1.4 (Mean vs. median). Let µ 2 Cα(X; d). Integrating (1) with respect to t results in Z (1) Z jµ(f) − mµ(f)j ≤ jf − mµ(f)j dµ ≤ 2 α(t) dt =: t0; from which it follows that µ(f > µ(f) + t) ≤ α(t − t0); R where µ(f) := X f dµ is a mean of f w.r.t. the measure µ. 1.2. Concentration inequalities. Let us focus now on some important results in the con- centration theory, beginning with the result due to Hoeffding [16]. Proposition 1.5 (Hoeffding’s inequality). Let Xi be an i.i.d sequence with a compact support enclosed in the interval [a; b]. It follows that t2 X¯ ≥ [X ] + t ≤ exp −2n ; P E 1 (b − a)2 ¯ 1 P were X = n Xi. The above result can be seen as an easy consequence of the following proposition. OPTIMAL TRANSPORT AND CONCENTRATION OF MEASURE 3 Proposition 1.6 (Hoeffding’s concentration inequality). Let µ 2 P(X) be a probability mea- ⊗n Nn ⊗n n sure on the space X and let µ := j=1 µ be the product measure. Then µ 2 Cαn (X ; dH ), 2 n where αn = exp(−2t =n) and dH is the Hamming distance on X given by the formula 1 d (x; y) = jfi : x 6= y gj : H n i i Remark 1.7. Proposition 1.6 implies Proposition 1.5. b−a Proof. Set µ = L(X1) be the law of X1. Then f(x) =x ¯ is n Lipschitz on the support of ⊗n ⊗n µ (recall Remark 1.2) w.r.t. dH . Applying the assumption that µ 2 Cαn (X; dH ) yields the proof. We now turn to the proof of Proposition 1.6. Proof of Proposition 1.6. The idea is to make use of exponential Chebyshev's inequality for F (X1;:::;Xn) where Xi are an i.i.d. sample, X1 ∼ µ and F is some 1-Lipschitz function w.r.t. tF (X ;:::;Xn) the metric dH . To that end, we need an estimate on the Laplace transform Ee 1 , which can be obtained using conditioning and some basic properties of the moment generating function. Let us split the proof into separate parts. Part 1. Firstly, let Z be a centered random variable on the interval [−1; 1]. And let φ(t) tZ denote its moment generating function, i.e. φ(t) = log Ee . Since ZetZ Z2etZ ZetZ 2 Z2etZ φ0(t) = E ; φ00(t) = E − E ≤ E ; EetZ EetZ EetZ EetZ then φ0(0) = 0 and φ00(t) ≤ 1 from which it follows that tZ t2=2 (2) Ee ≤ e : Part 2. Let EXk denote integration w.r.t. the variable Xk, that is EXk [f(X1;:::;Xn)] = E[f(X1;:::;Xn)jX1;:::;Xk−1;Xk+1;:::;Xn] n for any measurable function f. Note that since F 2 Lip1(X ; dH ), we have jF (x) − F (y)j ≤ 1 and thus F (X1;:::;Xn) − EF is a centered random variable on the interval [−1; 1], where EF := EF (X1;:::;Xn). We can now estimate h i h i tF (X1;:::;Xn) tF (X1;:::;Xn) E e = EX1;:::;Xn−1 EXn e (2) 2 ≤ EX1;:::;Xn−1 exp t =2 + EXn tF (X1;:::;Xn . 2 ≤ exp nt =2 + tEF: Part 3. Finally, it suffices to apply Chebyschev's exponential inequality s(F (X1;:::;Xn)−EF )−st 2 P (F (X1;:::;Xn) ≥ EF + t) ≤ Ee ≤ exp ns =2 − st : Optimizing with respect to s yields the proof. While Proposition 1.6 is much stronger than Proposition 1.5, it still suffers weak dimension scaling which demonstrates the following example. 4 NATHAEL GOZLAN Example. Take any f 2 Lip1(X; dH ). By Proposition 1.6 2 µ(f > mµ(f) + t) ≤ exp(−2t ): n But on the other hand, setting fn : X ! R, fn(x) = f(x1) results in ⊗n 2 µ (fn > mµ⊗n (f) + t) ≤ exp(−2t =n); which is clearly not optimal since ⊗n µ (fn > mµ⊗n (f) + t) = µ(f > mµ(f) + t): The example above gives a motivation to introduce a notion of a concentration that is not dependent on the dimension. Definition (dimension-free concentration property). A measure µ on (X; d) satisfies the 1 dimension-free concentration property with a concentration profile α, denoted as µ 2 Cα (X; d), ⊗n n if µ 2 Cα(X ; d2) for every n ≥ 1, where 1=2 X 2 d2(x; y) = d(x; y) : 1 Obviously Cα (X; d) ⊆ Cα(X; d). Converse is false which illustrates the following observa- tion left as an exercise. Exercise 1.1. Show that if µ satisfies the dimension-free concentration property, then supp µ is connected. A prominent example of a measure that satisfies the dimension-free concentration property d is Gaussian measure on R . Theorem 1.8 (Borell[4], Sudakov { Tsirelson[31]). Setting γ to be standard Gaussian mea- ¯ 1 sure on R, φ { its c.d.f. and φ(t) = 1 − φ(t) = γ (]t; +1[) we have γ 2 Cα¯ (R; |·|), where α = φ¯. Remark 1.9. The concentration profile φ¯ is optimal, which can be seen by plugging f(x) = x into the equivalent definition of the concentration property (see Proposition 1.1). The following exercise proves that obtained concentration profile is in fact subgaussian. Exercise 1.2. Show that for t > 0 ¯ 1 2 1. φ(t) ≤ 2 exp(−t =2), q 1 1 2 2. φ(t) ≤ 2π t exp(−t =2). The proof of Theorem 1.8 we are about to show is due to G. Piser [26]. It is much less technical than the original proof but provides a weaker concentration profile. Proof of Theorem 1.8 for α(t) = e−2t2/π2 . In the same manner as in the previous proof we will try to arrive at some estimate on the Laplace transform of a pushforward of γn by some Lipschitz function and then make use of Chebyshev's inequality.
Details
-
File Typepdf
-
Upload Time-
-
Content LanguagesEnglish
-
Upload UserAnonymous/Not logged-in
-
File Pages20 Page
-
File Size-