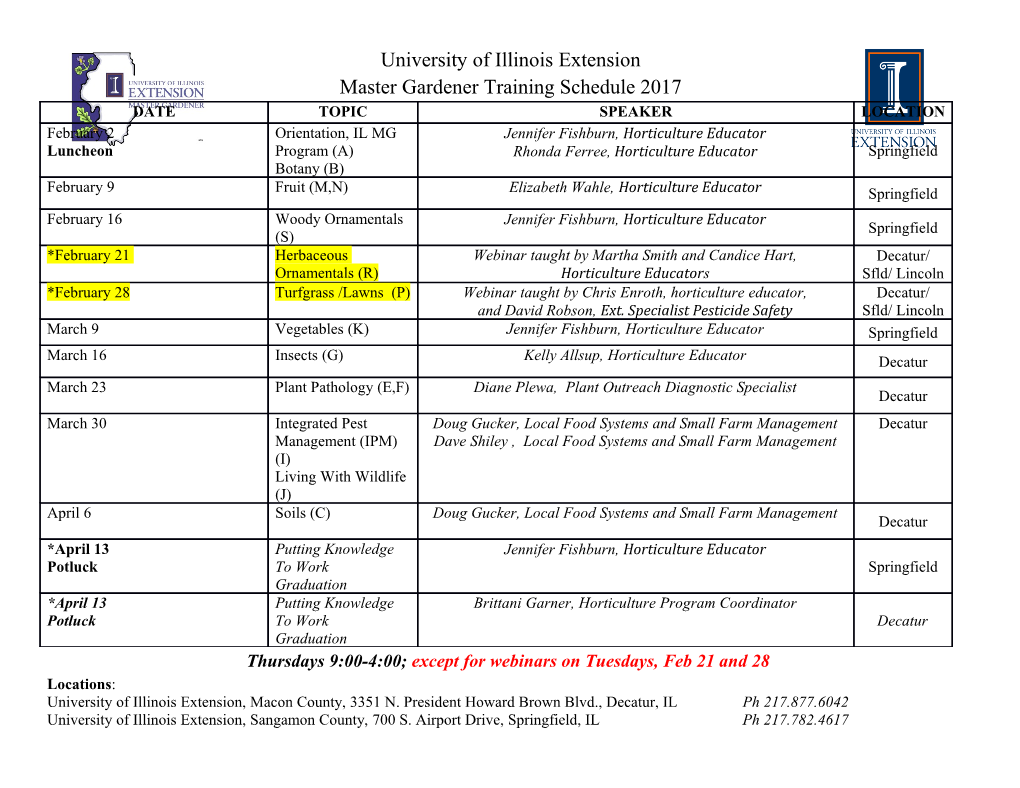
Contribution to High Performance Computing and Big Data Infrastructure Convergence Michael Mercier To cite this version: Michael Mercier. Contribution to High Performance Computing and Big Data Infrastructure Con- vergence. Symbolic Computation [cs.SC]. Université Grenoble Alpes, 2019. English. NNT : 2019GREAM031. tel-02284996v2 HAL Id: tel-02284996 https://hal.archives-ouvertes.fr/tel-02284996v2 Submitted on 31 Oct 2019 HAL is a multi-disciplinary open access L’archive ouverte pluridisciplinaire HAL, est archive for the deposit and dissemination of sci- destinée au dépôt et à la diffusion de documents entific research documents, whether they are pub- scientifiques de niveau recherche, publiés ou non, lished or not. The documents may come from émanant des établissements d’enseignement et de teaching and research institutions in France or recherche français ou étrangers, des laboratoires abroad, or from public or private research centers. publics ou privés. THÈSE Pour obtenir le grade de DOCTEUR DE LA COMMUNAUTÉ UNIVERSITÉ GRENOBLE ALPES Spécialité : Informatique Arrêté ministériel : 25 mai 2016 Présentée par Michael Mercier Thèse dirigée par Bruno RAFFIN et codirigée par Olivier RICHARD préparée au sein du Laboratoire d’Informatique de Grenoble dans l’École Doctorale Mathématiques, Sciences et technologies de l’information, Informatique Contribution à la convergence d’infrastructure entre le calcul haute performance et le traitement de données a large échelle Contribution to High Performance Computing and Big Data Infrastructure Convergence Thèse soutenue publiquement le 1er juillet 2019, devant le jury composé de : Frédéric SUTER Directeur de recherche, IN2P3, France, Président Kate KEAHEY Senior Fellow, Computation Institute University of Chicago, and Computer Scien- tist, MCS, Argonne National Laboratory, États-Unis, Rapporteur Gabriel ANTONIU Directeur de Recherche, Inria Rennes Bretagne, France, Rapporteur Frédéric DESPREZ Directeur de Recherche, LIG, Inria, France, Examinateur Christian PEREZ Directeur de Recherche, LIP, Inria, France, Examinateur Benoit PELLETIER Directeur de section R&D, Atos, France, Examinateur Bruno RAFFIN Directeur de Recherche, LIG, Inria, France, Directeur de thèse Olivier RICHARD Maître de conférences, LIG, Univ. Grenoble Alpes, France, Co-Directeur de thèse Abstract The amount of produced data, either in the scientific community or the commercial world, is constantly growing. The field of Big Data has emerged to handle large amounts of data on distributed computing infrastructures. High-Performance Com- puting (HPC) infrastructures are traditionally used for the execution of compute intensive workloads. However, the HPC community is also facing an increasing need to process large amounts of data derived from high definition sensors and large physics apparati. The convergence of the two fields -HPC and Big Data- is currently taking place. In fact, the HPC community already uses Big Data tools, which are not always integrated correctly, especially at the level of the file system and the Resource and Job Management System (RJMS). In order to understand how we can leverage HPC clusters for Big Data usage, and what are the challenges for the HPC infrastructures, we have studied multiple aspects of the convergence: We initially provide a survey on the software provi- sioning methods, with a focus on data-intensive applications. We contribute a new RJMS collaboration technique called BeBiDa which is based on 50 lines of code whereas similar solutions use at least 1000 times more. We evaluate this mecha- nism on real conditions and in simulated environment with our simulator Batsim. Furthermore, we provide extensions to Batsim to support I/O, and showcase the developments of a generic file system model along with a Big Data application model. This allows us to complement BeBiDa real conditions experiments with simulations while enabling us to study file system dimensioning and trade-offs. All the experiments and analysis of this work have been done with reproducibility in mind. Based on this experience, we propose to integrate the development workflow and data analysis in the reproducibility mindset, and give feedback on our experiences with a list of best practices. ii Résumé La quantité de données produites, que ce soit dans la communauté scientifique ou commerciale, est en croissance constante. Le domaine du Big Data a émergé face au traitement de grandes quantités de données sur les infrastructures infor- matiques distribuées. Les infrastructures de calcul haute performance (HPC) sont traditionnellement utilisées pour l’exécution de charges de travail intensives en cal- cul. Cependant, la communauté HPC fait également face à un nombre croissant de besoin de traitement de grandes quantités de données dérivées de capteurs haute définition et de grands appareils physique. La convergence des deux domaines -HPC et Big Data- est en cours. En fait, la communauté HPC utilise déjà des outils Big Data, qui ne sont pas toujours correctement intégrés, en particulier au niveau du système de fichiers ainsi que du système de gestion des ressources (RJMS). Afin de comprendre comment nous pouvons tirer parti des clusters HPC pour l’utilisation du Big Data, et quels sont les défis pour les infrastructures HPC, nous avons étudié plusieurs aspects de la convergence: nous avons d’abord proposé une étude sur les méthodes de provisionnement logiciel, en mettant l’accent sur les applications utilisant beaucoup de données. Nous contribuons a l’état de l’art avec une nouvelle technique de collaboration entre RJMS appelée BeBiDa basée sur 50 lignes de code alors que des solutions similaires en utilisent au moins 1000 fois plus. Nous évaluons ce mécanisme en conditions réelles et en environnement simulé avec notre simulateur Batsim. En outre, nous fournissons des extensions à Batsim pour prendre en charge les entrées/sorties et présentons le développements d’un modèle de système de fichiers générique accompagné d’un modèle d’application Big Data. Cela nous permet de compléter les expériences en conditions réelles de BeBiDa en simulation tout en étudiant le dimensionnement et les différents compromis autours des systèmes de fichiers. Toutes les expériences et analyses de ce travail ont été effectuées avec la repro- ductibilité à l’esprit. Sur la base de cette expérience, nous proposons d’intégrer le flux de travail du développement et de l’analyse des données dans l’esprit de la reproductibilité, et de donner un retour sur nos expériences avec une liste de bonnes pratiques. iii Contents 1 Introduction 1 2 HPC and Big Data: Definition, Comparison, and Convergence 5 2.1 History.................................. 6 2.1.1 History of High-Performance Computing........... 6 2.1.2 History of Data Processing and Big Data ........... 8 2.1.3 History of the convergence................... 9 2.2 Workloads................................ 10 2.2.1 HPC workloads......................... 11 2.2.2 Big Data workloads....................... 12 2.2.3 Workloads Convergence .................... 14 2.3 Applications and Runtimes....................... 16 2.3.1 Keep as-is ............................ 18 2.3.2 Modify.............................. 19 2.3.3 Rewrite ............................. 20 2.4 Hardware ................................ 21 2.4.1 Big Data Hardware....................... 21 2.4.2 HPC Hardware ......................... 22 2.4.3 Converged Hardware...................... 23 2.5 Infrastructure.............................. 23 2.5.1 Resource and Job Management System............ 23 2.5.2 File Systems........................... 26 2.5.3 Network............................. 32 2.5.4 Virtualization and Isolation .................. 32 3 Software Stack Provisioning for HPC Platforms 35 3.1 Introduction............................... 36 3.2 HPC: user requirements and constraints ............... 37 3.2.1 Who’s who on HPC platforms ................. 37 3.2.2 Build............................... 39 3.2.3 Install .............................. 40 3.2.4 Configure ............................ 41 iv 3.2.5 Run ............................... 41 3.2.6 Package and Share ....................... 42 3.2.7 Summary ............................ 43 3.3 Traditional Package Managers..................... 43 3.4 Virtual Machines ............................ 44 3.5 Containers................................ 45 3.5.1 Singularity............................ 47 3.5.2 Charlie cloud .......................... 48 3.5.3 Udocker............................. 48 3.5.4 Container integration to RJMS................. 49 3.5.5 Summary ............................ 51 3.6 HPC provisioning............................ 51 3.6.1 Bare metal provisioning .................... 52 3.6.2 Do It Yourself.......................... 53 3.6.3 Environment Modules ..................... 53 3.6.4 EasyBuild ............................ 54 3.6.5 Spack .............................. 54 3.6.6 Summary ............................ 55 3.7 Functional Package Managers..................... 55 3.7.1 Nix................................ 56 3.7.2 Guix............................... 57 3.7.3 Summary ............................ 58 3.8 Conclusion................................ 59 4 BeBiDa: Best effort Big Data on HPC cluster 61 4.1 Introduction............................... 63 4.2 Definition of the Collocation Problem................. 66 4.3 Related works.............................. 68 4.3.1 Static partitioning........................ 69 4.3.2 Batch submission........................ 69 4.3.3 Scheduler Adapter ......................
Details
-
File Typepdf
-
Upload Time-
-
Content LanguagesEnglish
-
Upload UserAnonymous/Not logged-in
-
File Pages203 Page
-
File Size-