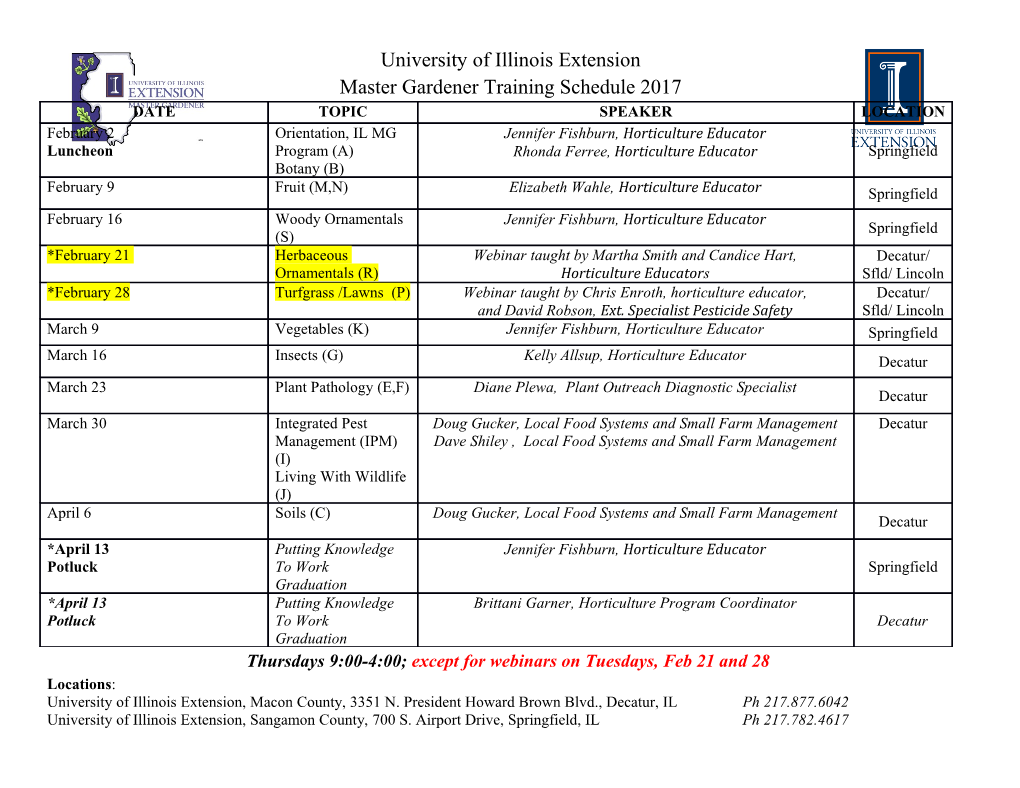
SDS 384 11: Theoretical Statistics Lecture 8: U Statistics Purnamrita Sarkar Department of Statistics and Data Science The University of Texas at Austin U Statistics • We will see many interesting examples of U statistics. • Interesting properties • Unbiased • Reduces variance • Concentration (via McDiarmid) • Asymptotic variance • Asymptotic distribution 1 An estimable parameter • Let P be a family of probability measures on some arbitrary measurable space. • We will now define a notion of an an estimable parameter. (coined \regular parameters" by Hoeffding.) • An estimable parameter θ(P) satisfies the following. Theorem (Halmos) θ admits an unbiased estimator iff for some integer m there exists an iid unbiased estimator of θ(P) based on X1;:::; Xm ∼ P that is, if there exists a real-valued measurable function h(X1; :::; Xm) such that θ = Eh(X1;:::; Xm): The smallest integer m for which the above is true is called the degree of θ(P). 2 U statistics • The function h may be taken to be a symmetric function of its arguments. • This is because if f (X1;:::; Xm) is an unbiased estimator of θ(P), so is P π2Πm f (Xπ1;:::; Xπm ) h(X ;:::; Xm) := 1 m! • For simplicity, we will assume h is symmetric for our notes. 3 U Statistics (Due to Wassily Hoeffding in 1948) Definition iid Let Xi ∼ f , let h(x1;:::; xr ) be a symmetric kernel function and Θ(F ) = E[h(x1;:::; xr )]. A U-statistic Un of order r is defined as P h(X ; X ;:::; X ) fi1;:::;ir g2Ir i1 i2 ir Un = n ; r where Ir is the set of subsets of size r from [n]. 4 Sample variance as an U-Statistic Example The sample variance is an U-statistic of order 2. Proof. Let θ(F ) = σ2. n X 2 X 2 X (Xi − Xj ) = 2n Xi − 2 Xi Xj i6=j i i;j X 2 2 ¯2 = 2n Xi − 2n X i P X 2 − nX¯2 = 2n(n − 1) i i n − 1 Pn 2 i<j (Xi − Xj ) =2 2 Un := = 2s n(n − 1)=2 n 5 Sample variance as U-statistic • Is its expectation the variance? 1 1 • E[(X − X )2] = E(X − µ − (X − µ))2 = σ2 2 1 2 2 1 2 6 U-statistics examples: Wilcoxon one sample rank statistic Example X Un = Ri 1(Xi > 0), where Ri is the rank of Xi in the sorted order i jX1j ≤ jX2j ::: . • This is used to check if the distribution of Xi is symmetric around zero. • Assume Xi to be distinct. n X • Ri = 1(jXj j ≤ jXi j) j=1 7 U-statistics examples: Wilcoxon one sample rank statistic Example X Tn = Ri 1(Xi > 0), where Ri is the rank of Xi in the sorted order i jX1j ≤ jX2j ::: . n n X X X Tn = Ri 1(Xi > 0) = 1(jXj j ≤ jXi j)1(Xi > 0) i i=1 j=1 n n n n X X X X = 1(jXj j ≤ Xi )1(Xi 6= 0) = 1(jXj j < Xi ) + 1(Xi > 0) i=1 j=1 i6=j i=1 n X X X = 1(jXj j < Xi ) + 1(jXi j < Xj ) + 1(Xi > 0) i<j i<j i=1 n ! X X n = 1(X + X > 0) + 1(X > 0) = U + nU i j i 2 2 1 i<j i=1 • Asymptotically dominated by the first term, which is an U statistic. • Why isn't it a U statistic? 8 Kendal's Tau Example Let P1 = (X1; Y1) and P2 = (X2; Y2) be two points. P1 and P2 are called concordant if the line joining them (call this P1P2) has a positive slope and discordant if it has a negative slope. Kendal's tau is defined as: τ := P(P1P2 has +ve slope) − P(P1P2 has -ve slope) • This is very much like a correlation coefficient, i.e. lies between −1; 1 • Its zero when X ; Y are independent, and ±1 when Y = f (X ) is a monotonically increasing (or decreasing) function. 9 Kendal's Tau 8 <1 If P1; P2 is concordant • Define h(P1; P2) = :−1 If P1; P2 is discordant • Now define h(P1; P2) = sgn(X1 − X2)(Y1 − Y2) P i<j h(Pi ; Pj ) • So U = n is an U statistic which computes Kendals 2 Tau, and it has order 2. 10 More novel examples Example (Gini's mean difference/ mean absolute deviation) Let θ(F ) := E[jX − X j]; the corresponding U statistic is P 1 2 i<j jxi − xj j Un = n . 2 Example (Quantile Statistic) Let θ(F ) := P(X ≤ t) = E[1(X ≤ t)]; the corresponding U statistic is P 1 1 i 1(Xi ≤ t) Un = . n 11 Properties of the U-statistic • The U is for unbiased. • Note that E[U] = Eh(X1;:::; Xr ) • var(U(X1;:::; Xr )) ≤ var(h(X1;:::; Xr )) (Rao Blackwell theorem) • Just h(X1;:::; Xr ) is an unbiased estimator of θ(F ). • But averaging over many subsets reduces variance. 12 Properties of U-statistics • Let X(1) :::; X(n) denote the order statistics of the data. • The empirical distribution puts 1=n mass on each data point. • So we can think about the U statistic as Un = E[h(X1;:::; Xr )jX(1);:::; X(n)] • We also have: 2 2 E[(U − θ) ] = E E[h(X1;:::; Xr ) − θjX(1);:::; X(n)] 2 ≤ E[E[(h(X1;:::; Xr ) − θ) jX(1);:::; X(n)]] = var(h(X1;:::; Xr )) • Rao-Blackwell theorem says that the conditional expectation of any estimator given the sufficient statistic has smaller variance than the estimator itself. iid • For X1;:::; Xn ∼ P, the order statistics are sufficient. (why?) 13 Concentration P i<j h(Xi ; Xj ) • Consider a U statistic of order 2 U = n : 2 • How does U concentrate around its expectation? • Recall McDiarmid's inequality? Theorem n Let f : X ! R satisfy the following bounded difference condition 0 8x1;:::; xn; xi 2 X : 0 jf (x1;:::; xi−1; xi ; xi+1;:::; xn) − f (x1;:::; xi−1; xi ; xi+1;:::; xn)j ≤ Bi ; ! 2t2 then, P(jf (X ) − E[f (X )]j ≥ t) ≤ 2 exp − P 2 i Bi 14 Concentration P i<j h(Xi ; Xj ) Consider a U statistic of order 2. U = n : 2 Theorem If jh(X1; X2)j ≤ B a.s., then, ! nt2 P(jU − E[U]j ≥ t) ≤ 2 exp − : 8B2 15 Concentration Proof. • Consider two samples X ; X 0 which differ in the ith coordinate. P 0 0 j6=i jh(Xi ; Xj ) − h(Xi ; Xj )j jU(X ) − U(X )j ≤ n • We have: 2 . 4B ≤ n • Now we have: ! nt2 P(jU − E[U]j ≥ t) ≤ 2 exp − : 8B2 16 Concentration P h(X ;:::; X ) i2Ir i1 ir Now consider a U statistic of order r. U = n : r Theorem If jh(X ;:::; X )j ≤ B a.s., then, i1 ir ! nt2 P(jU − E[U]j ≥ t) ≤ 2 exp − : 2r2B2 17 Concentration Proof. • Consider two samples X ; X 0 which differ in the first coordinate. • Let Ir−1 is the set of r − 1 subsets from 2;:::; n. • We have: P jh(X ; X ;:::; X ) − h(X ; X 0 ;:::; X 0 )j 0 j2Ir−1 1 j1 jr 1 j1 jr jU(X ) − U(X )j ≤ n r . n−1 2B r−1 2rB ≤ n = r n • Now we have: ! nt2 P(jU − E[U]j ≥ t) ≤ 2 exp − : 2r2B2 18 Hoeffding’s bound from his 1963 paper P h(X ;:::; X ) i2Ir i1 ir Now consider a U statistic of order r. U = n : r Theorem If jh(X ;:::; X )j ≤ B a.s., then, i1 ir ! bn=rct2 P(jU − E[U]j ≥ t) ≤ 2 exp − : 2B2 • What are we missing? 19 Lets start with Markov X X • First note that if I can write U − E[U] = pi Ti where pi = 1, i i • Then, X P(U − E[U] ≥ t) ≤ E[exp(λ pi (Ti − t))] i X ≤ pi E[exp(λ(Ti − t))] i • So, if Ti is a sum of independent random variables, we can plug in previous bounds into the above. • But how can we write the U statistics as a sum of such Ti 's? 20 Lets do a bit of combinatorics • For simplicity assume that n = kr. h(X1;:::; Xr ) + ··· + h(X(k−1)r+1;:::; Xkr )) • Write V (X ;:::; Xn) = 1 k P V (Xπ ;:::; Xπn ) • Note that U = π2Π 1 n! • So set Tπ = V (Xπ1;:::; Xπn ) − E[:]. • Since V is an average of k = n=r independent random variables, using Hoeffding’s inequality we have 2 2 2 2 E[exp(λ(Ti − t))] ≤ exp(−λt + λ B =2k) ≤ exp(−kt =2B ) • Since each Vπ behave stochastically equivalently, we can take the λ the same everywhere. 21 Variance of U statistic Next time! 22.
Details
-
File Typepdf
-
Upload Time-
-
Content LanguagesEnglish
-
Upload UserAnonymous/Not logged-in
-
File Pages23 Page
-
File Size-