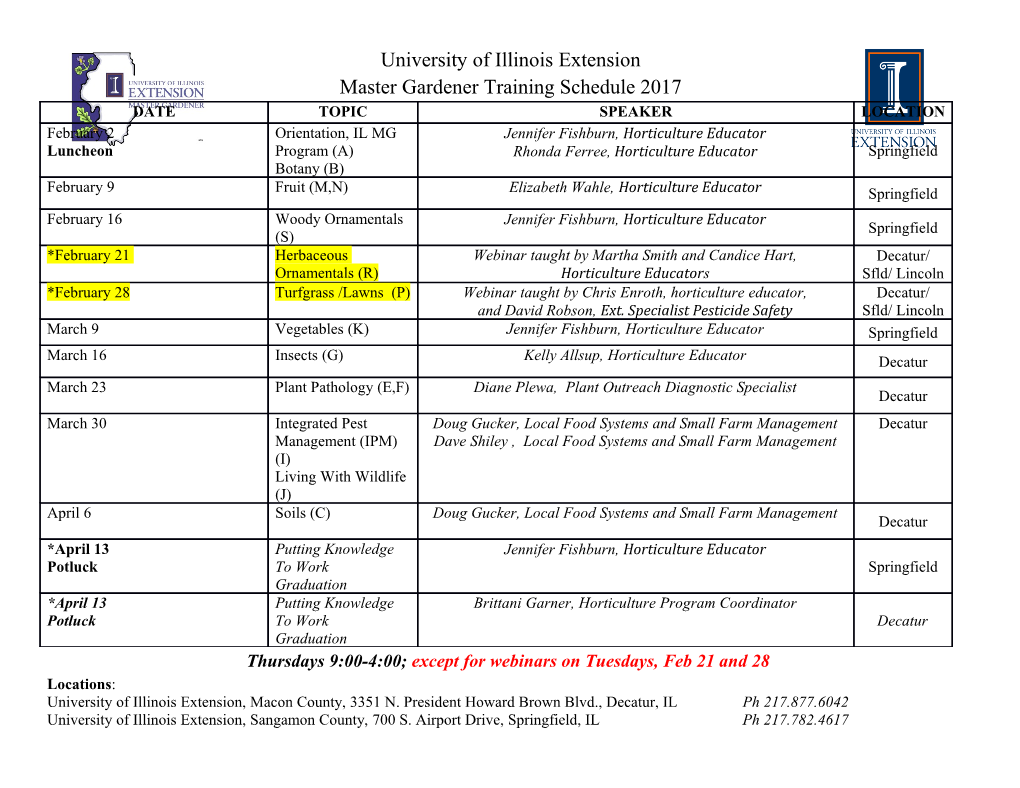
Dynamic hand gesture recognition - From traditional handcrafted to recent deep learning approaches Quentin de Smedt To cite this version: Quentin de Smedt. Dynamic hand gesture recognition - From traditional handcrafted to recent deep learning approaches . Computer Vision and Pattern Recognition [cs.CV]. Université de Lille 1, Sciences et Technologies; CRIStAL UMR 9189, 2017. English. tel-01691715 HAL Id: tel-01691715 https://hal.archives-ouvertes.fr/tel-01691715 Submitted on 24 Jan 2018 HAL is a multi-disciplinary open access L’archive ouverte pluridisciplinaire HAL, est archive for the deposit and dissemination of sci- destinée au dépôt et à la diffusion de documents entific research documents, whether they are pub- scientifiques de niveau recherche, publiés ou non, lished or not. The documents may come from émanant des établissements d’enseignement et de teaching and research institutions in France or recherche français ou étrangers, des laboratoires abroad, or from public or private research centers. publics ou privés. Année 2017 Num d’ordre: 42562 Université Lille 1 Ecole Doctorale Sciences Pour l’Ingénieur Laboratoire CRIStAL (umr cnrs 9189) THÈSE pour obtenir le grade de Docteur, spécialité Informatique soutenu par Quentin De Smedt le 14/12/2017 Dynamic hand gesture recognition - From traditional handcrafted to recent deep learning approaches COMPOSITION DU JURY M. Laurent Grisoni Professeur, Université de Lille 1 Président Mme. Saida Bouakaz Professeur, Université Claude Bernard Lyon 1 Rapporteur M. Fabien Moutarde Professeur, Mines ParisTech Rapporteur M. Francisco Flórez-Revuelta Associate professor, Université Alicante, Espagne Examinateur M. Jean-Philippe Vandeborre Professeur, IMT Lille Douai Directeur M. Hazem Wannous Maître de conférences, Université de Lille 1 Encadrant Abstract Hand gestures are the most natural and intuitive non-verbal communica- tion medium while using a computer, and related research efforts have recently boosted interest. Additionally, data provided by current com- mercial inexpensive depth cameras can be exploited in various gesture recognition based systems. The area of hand gesture analysis covers hand pose estimation and gesture recognition. Hand pose estimation is con- sidered to be more challenging than other human part estimation due to the small size of the hand, its greater complexity and its important self occlusions. Beside, the development of a precise hand gesture recognition system is also challenging due to high dissimilarities between gestures derived from ad-hoc, cultural and/or individual factors of users. First, we propose an original framework to represent hand gestures by using hand shape and motion descriptors computed on 3D hand skeletal fea- tures. We use a temporal pyramid to model the dynamic of gestures and a linear SVM to perform the classification. Additionally, we create the Dynamic Hand Gesture dataset containing 2800 sequences of 14 gesture types. Evaluation results show the promising way of using hand skele- tal data to perform hand gesture recognition. Experiments are carried out on three hand gesture datasets, containing a set of fine and coarse heterogeneous gestures. Furthermore, results of our approach in terms of latency demonstrated improvements for a low-latency hand gesture recog- nition systems, where an early classification is needed. Then, we extend the study of hand gesture analysis to online recognition. Using a deep learning approach, we employ a transfer learning strategy to learn hand posture and shape features from depth image dataset originally created for hand pose estimation. Second, we model the temporal variations of the hand poses and its shapes using a recurrent deep learning technology. Finally, both information are merged to perform accurate prior detection and recognition of hand gestures. Experiments on two datasets demon- strate that the proposed approach is capable to detect an occurring gesture and to recognize its type far before its end. i ii Abstract Key words: hand gesture recognition, depth data, skeletal data, deep learning, online detection. Résumé Reconnaisance de gestes dynamiques de la main - De la création de descripteurs aux récentes méthodes d’apprentissage profond. Les gestes de la main sont le moyen de communication non verbal le plus naturel et le plus intuitif lorsqu’il est question d’interaction avec un ordinateur. Les efforts de recherche qui y sont liés ont récemment relancé son intérêt. De plus, les données fournies par des caméras de pro- fondeur actuellement commercialisées à des prix abordables peuvent être exploitées dans une large variété de systèmes de reconnaissance de gestes. L’analyse des gestes de la main s’appuie sur l’estimation de la pose de la main et la reconnaissance de gestes. L’estimation de la pose de la main est considérée comme étant un défi plus important que l’estimation de la pose de n’importe quelle autre partie du corps du fait de la petite taille d’une main, de sa plus grande complexité et de ses nombreuses occultations. Par ailleurs, le développement d’un système précis de reconnaissance des gestes de la main est également difficile du fait des grandes dissimilarités entre les gestes dérivant de facteurs ad-hoc, culturels et/ou individuels in- hérents aux acteurs. Dans un premier temps, nous proposons un système original pour représenter les gestes de la main en utilisant des descrip- teurs de forme de main et de mouvement calculés sur des caractéristiques de squelette de main 3D. Nous utilisons une pyramide temporelle pour modéliser la dynamique des gestes et une machine à vecteurs de support (SVM) pour effectuer la classification. De plus, nous proposons une base de données de gestes de mains dynamiques contenant 2800 séquences de 14 types de gestes. Les résultats montrent une utilisation prometteuse des données de squelette pour reconnaître des gestes de la main. Des ex- périmentations sont menées sur trois ensembles de données, contenant un ensemble de gestes hétérogènes fins et grossiers. En outre, les résultats de notre approche en termes de latence ont démontré que notre système peut reconnaître un geste avant sa fin. Dans un second temps, nous étendons l’étude de l’analyse des gestes de main à une reconnaissance en ligne. En utilisant une approche d’apprentissage profond, nous employons une iii iv Résumé strategie de transfert d’apprentissage afin d’entrainer des caractéristiques de pose et de forme de la main à partir d’images de profondeur d’une base de données crée à l’origine pour un problème d’estimation de la pose de la main. Nous modélisons ensuite les variations temporelles des poses de la main et de ses formes grâce à une methode d’apprentissage profond récurrente. Enfin, les deux informations sont fusionnées pour effectuer une détection préalable et une reconnaissance précise des gestes de main. Des expériences menées sur deux ensembles de données ont démontré que l’approche proposée est capable de détecter un geste qui se produit et de reconnaître son type bien avant sa fin. Mots-clés reconnaisance de gestes de la main, données de profondeur, données de squelettes, apprentissage profond, detection en temps réel. Acknowledgement A thesis is often defined as a lonely process made by a single PhD student. In practice, it is the opposite. In hindsight, I never could have reach the end of my thesis without the help and the engagement of many people the last three years. Special thanks to my PhD committee members for taking the time to participate in this process, and especially the reviewers of the manuscript for having accepted this significant task: Prof. Fabien Moutarde, Prof. Saida Bouakaz, Ass. Prof. Francisco Flórez-Revuelta and Prof. Laurent Grisoni. I would like to express my gratitude to my advisor, Prof. Jean-Philippe Vandeborre for guiding me through my research with professionalism, understanding, and patience. His high experience strongly helped me to make this experience productive and stimulating. I also thank my co-advisor, Dr. Hazem Wannous, for his time and advice in beneficial scientific discussions. His friendly and encouraging guidance make me more confident in my research. A special thanks to my friends and PhD student colleagues with whom I shared this experience: Maxime Devanne, Vincent Léon, Meng Meng, Taleb Alashkar, Matthieu Allon, Vincent Itier, Sarah Ribet, Anis Kacem, Omar Ben Tanfous, Kaouthar Larbi and Nadia Hosni. I also thank gratefully my family and my friends for their support and encouragements. I would like to cite them all but I am too afraid to forget one and I know they will make me pay for it. Villeneuve d’Ascq, October 16, 2017. v Publications International journal • Quentin De Smedt, Hazem Wannous and Jean-Philippe Vande- borre. Heterogeneous Hand Gesture Recognition Using 3D Dynamic Skeletal Data. Computer Vision and Image Understanding, (Under re- view). International worshops • Quentin De Smedt, Hazem Wannous, Jean-Philippe Vandeborre, Joris Guerry, Bertrand Le Saux and David Filliat. SHREC’17 Track: 3D Hand Gesture Recognition Using a Depth and Skeletal Dataset. 10th Eurographics Workshop on 3D Object Retrieval, 2017. • Quentin De Smedt, Hazem Wannous and Jean-Philippe Vande- borre. Skeleton-based dynamic hand gesture recognition. Proceed- ings of the IEEE Conference on Computer Vision and Pattern Recognition (CVPR) Workshops, 2016. • Quentin De Smedt, Hazem Wannous and Jean-Philippe Vande- borre. 3D Hand Gesture Recognition by Analysing Set-of-Joints Tra- jectories. 2nd International Conference on Pattern Recognition (ICPR)
Details
-
File Typepdf
-
Upload Time-
-
Content LanguagesEnglish
-
Upload UserAnonymous/Not logged-in
-
File Pages214 Page
-
File Size-