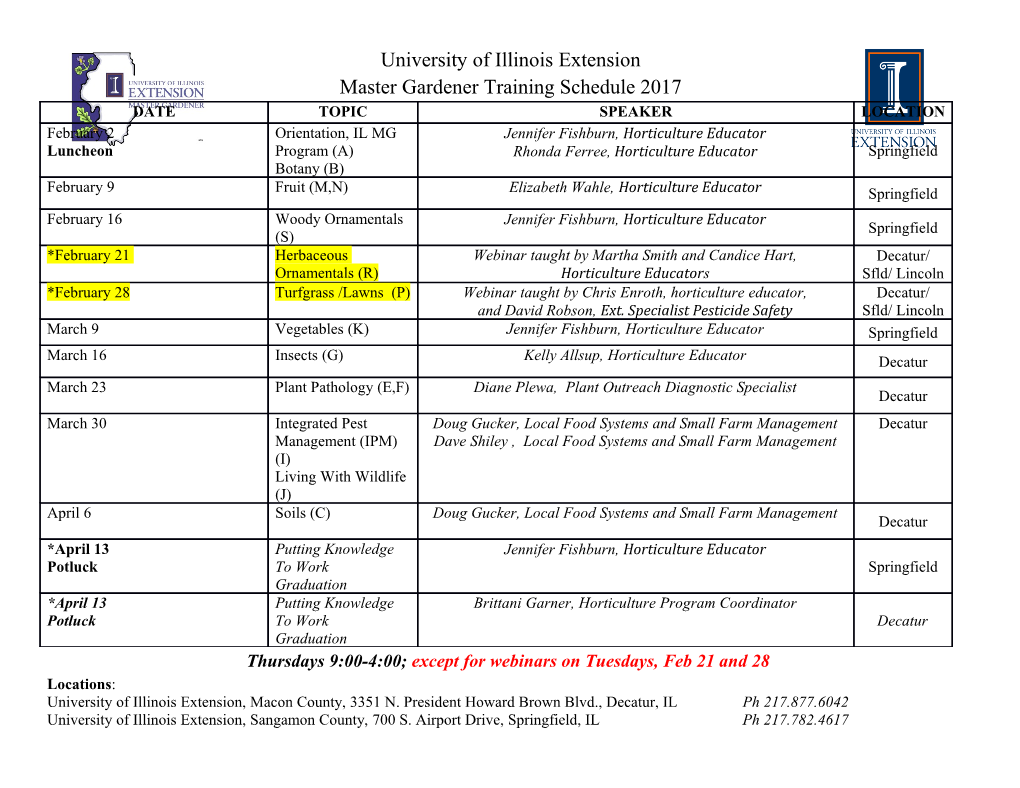
Die approbierte Originalversion dieser Diplom-/ Masterarbeit ist in der Hauptbibliothek der Tech- nischen Universität Wien aufgestellt und zugänglich. http://www.ub.tuwien.ac.at The approved original version of this diploma or master thesis is available at the main library of the Vienna University of Technology. http://www.ub.tuwien.ac.at/eng Large-scale bird song identification using convolutional neural networks MASTER’S THESIS submitted in partial fulfillment of the requirements for the degree of Master of Science in Computational Intelligence by Botond Fazekas, BSc Registration Number 0925351 to the Faculty of Informatics at the TU Wien Advisor: Ao.Univ.Prof. Dipl.-Ing. Dr.techn. Andreas Rauber Assistance: Dipl.-Ing. Thomas Lidy Dipl.-Ing. Alexander Schindler Vienna, 3rd May, 2018 Botond Fazekas Andreas Rauber Technische Universität Wien A-1040 Wien Karlsplatz 13 Tel. +43-1-58801-0 www.tuwien.ac.at Erklärung zur Verfassung der Arbeit Botond Fazekas, BSc Linzer Straße 261/2/25, 1140 Wien, Österreich Hiermit erkläre ich, dass ich diese Arbeit selbständig verfasst habe, dass ich die verwen- deten Quellen und Hilfsmittel vollständig angegeben habe und dass ich die Stellen der Arbeit – einschließlich Tabellen, Karten und Abbildungen –, die anderen Werken oder dem Internet im Wortlaut oder dem Sinn nach entnommen sind, auf jeden Fall unter Angabe der Quelle als Entlehnung kenntlich gemacht habe. Wien, 3. Mai 2018 Botond Fazekas iii Kurzfassung Um Ökosysteme begreifen zu können, ist es wichtig, Wildtiere zu verstehen; deren Beob- achtung kann jedoch kompliziert und zeitintensiv sein. Eine automatisierte Aufnahme von Tönen und Lauten der Umwelt selbst ist ein einfaches Unterfangen, für eine nach- trägliche Identifizierung der Wildtiere ist allerdings ein komplexeres System vonnöten. Eine manuelle Identifizierung wäre zu umständlich, wodurch eine automatisierte Methode im Forschungsbereich eine vielversprechende Alternative wäre. Vögel eigenen sich dabei besonders gut für diese Aufgabe, da ihre Kommunikation zum Großteil durch das Singen passiert und sie darüber hinaus aufgrund ihrer schnellen Reaktion auf Änderungen in ihrer Umgebung gute ökologische Indikatoren darstellen. Die aktuell verfügbaren Datensets beinhalten sehr viele Vogelgesänge verschiedenster Arten in diversen Umgebungen und so ist neben der Klassifizierungs-Genauigkeit auch die Skalierbarkeit ein wichtiger Faktor. Das Ziel dieser Studie ist das Verbessern von den akustischen state-of-the-art Vögel- identifzierungs-Methoden, die in dem BirdCLEF2016 Wettbewerb1 evaluiert wurden, sowohl im Sinne der Genauigkeit als auch der benötigten Trainingszeit, und damit im Sinne der Skalierbarkeit. Diese Arbeit beschreibt die Vorbereitungs-Schritte, die für die Unterscheidung von Vogelgesang von Hintergrundsgeräuschen verwendet wurde, sie evalu- iert die Leistung von den vorgeschlagenen Convolutional Neural Network (CNN) Modellen mit Rectified Linear Units und Exponential Linear Units (ELU) mit dem BirdCLEF2017 Datenset, sowie den Effekt von Mel-Skalierung und Constant-Q Transformation der Töne. Außerdem wird in dieser Arbeit eine neue, multi-modale Architektur präsentiert, die die verschiedenen vorhandenen Metadaten für die Feld-Aufnahmen für die Klassifizierung verwendet. Die Ergebnisse zeigen, dass einfachere CNN Modelle mit ELUs im Bezug auf Trainingszeit und Klassifizierungs-Leistung besser abschneiden als jene state-of-the-art Lösungen, wohingegen die Verwendung von Metadaten einen deutlich positiveren Effekt auf die Identifizierungs-Genauigkeit hat. 1http://www.imageclef.org/lifeclef/2016/bird v Abstract Understanding wildlife population is important for understanding ecosystems, monitoring it, however, is difficult and time-consuming. Automatically capturing environmental sounds is easy, but it requires subsequent identification of the wildlife. Doing this manually in cumbersome, thus automated methods are a promising field of research. Birds are especially well fitted for this task as their main way of communication is by singing and they are an important ecological indicator since they are responding quickly to changes in their environment. The current datasets available contain a large number of bird songs of different species in various environments, hence besides the classification accuracy the scalability of the methods is an important factor, too. The aim of this study is to improve upon the state-of-the-art acoustical bird identification methods evaluated in the BirdCLEF2016 competition2 in terms of both the identification accuracy as well as the required training time and therefore the scalability. The work describes the pre-processing steps used to separate the bird songs from the background noise, it evaluates the performance proposed simpler convolutional neural network (CNN) models with Rectified Linear Units and Exponential Linear Units on the BirdCLEF2017 dataset, along with the effect of Mel-scaling and Constant-Q transforming of the sounds. Furthermore a novel multi-modal architecture is proposed which, incorporates the various metadata available for the field recordings. The results show that the simpler CNN model with exponential linear units largely improves on the training time and classification performance compared to the state-of- the-art solutions, while using metadata significantly has a major positive effect on the identification accuracy. 2http://www.imageclef.org/lifeclef/2016/bird vii Contents Kurzfassung v Abstract vii Contents ix 1 Introduction 1 1.1 Motivation . 1 1.2 Aim of the work . 2 1.3 Structure of the work . 2 2 Background 5 2.1 Machine learning in general . 5 2.2 Neural networks . 10 2.3 Convolutional neural networks . 15 2.4 Signal processing . 19 2.5 Summary . 20 3 Related works 21 3.1 Acoustic signal processing with CNNs . 21 3.2 Bird classification challenges . 21 3.3 Summary . 26 4 Methodology 29 4.1 Dataset . 29 4.2 Pre-processing . 32 4.3 Network architectures . 38 4.4 Combining the single predictions . 41 4.5 Evaluation methods . 41 4.6 Summary . 43 5 Results 45 5.1 Sound representation . 45 5.2 Metadata . 46 ix 5.3 Data augmentation . 47 5.4 Network architecture . 48 5.5 Activation functions and training times . 49 5.6 Discussion . 50 6 BirdCLEF 2017 53 6.1 Submitted runs . 53 6.2 Other participating teams . 54 6.3 Results and analysis . 55 7 Conclusion and future work 59 7.1 Future work . 59 List of Figures 61 List of Tables 63 Acronyms 65 Bibliography 67 CHAPTER 1 Introduction As the world is facing the climate change, it is getting increasingly important to get acquainted with the animals living in the wild, to discover their habitats and where they live in order to preserve their biodiversity and to understand the impact of humans on the world’s ecology [HP05]. Higher biodiversity has been showed to have positive effects on the human health and on the food chain thus affecting also the economy [SMP12, p. 3–5]. There is still a long way to go for the humanity as it is estimated that there are about 10-14 millions of different species in the world of which only 1.2 million has been described and categorized. Most of these species are concentrated to the tropical forests which only cover 10% of the earth surface while they contain 90% of all living species [You03]. These biomes are hard to study because of their distance from more developed areas and thus they are hard to access. Automatic identification tools may help support the monitoring of animals present in these areas and to close this taxonomic gap. There has been several studies and results in this field [CEP+07, GO04, TPN+12]. 1.1 Motivation Birds are very sensitive to changes in their environments and this renders them a good ecological indicator [GNF+03]. However, due to the great diversity of the bird species, traditional approaches require professional knowledge and it is difficult for the general public. Although an automated visual observation is possible, it is a complicated task especially in rain forests with very dense flora. Therefore most research concentrate on the use of acoustic signals to monitor and classify animals [BLN+12, BHR+13]. Several public communities emerged in the past decades that focus on the collection of acoustic observations of birds (e.g. eBird1, Xeno-canto2) which may help domain experts around the world to use them in their research without the need of traveling to the native 1http://ebird.org/ 2http://www.xeno-canto.org 1 1. Introduction territory of these animals. However, it is hard for the professionals to keep the pace with the growth of these data sets [GGV+14], so several competitions have been held in the previous years in order to stimulate the research in the automatic classification of bird vocalizations (See Section 3.2). The major challenges include the vast number of bird species, simultaneously vocalizing birds of the same or of different species, sound of other animals in the area (e.g. insects) and background noise like rain, wind or vehicles. 1.2 Aim of the work The aim of this thesis is to improve performance of the state-of-the-art acoustic bird classification solution [SJKH16], in the sense of both the classification performance and the scalability, i.e. the time required for the training. Many cutting edge Convolutional Neural Network (CNN) models are highly complex and thus require professional equipment and large amount of time to train [SVI+16, SIVA17]. In some cases the training must be stopped because of time constraints before a full convergence was reached [SG17]. An objective of this thesis is to evaluate simpler CNN architectures for bird song classification which can be efficiently trained with a large amount of data, while improving the classification performance. Furthermore, two methods for perceptual scaling of pitches, Mel-Scale and Constant-Q transform are examined. While the perceptual scaling yields smaller inputs and thus smaller models, they may lead to information loss, especially as these methods were designed for the human sound perception system. The state-of-the-art solutions do not incorporate all of the information available e.g. the date/time and the location of the recordings, therefore an additional objective of this thesis is to evaluate the effects of the metadata on the performance of the classification.
Details
-
File Typepdf
-
Upload Time-
-
Content LanguagesEnglish
-
Upload UserAnonymous/Not logged-in
-
File Pages84 Page
-
File Size-