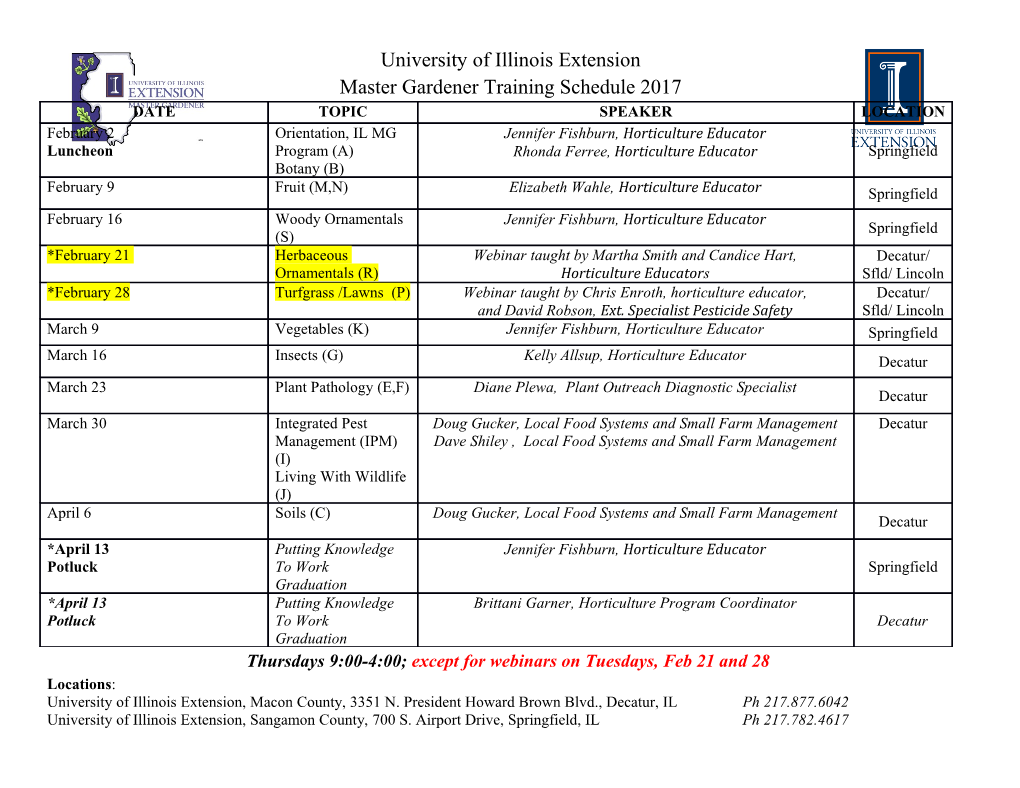
A Novel Wavelet Based Approach for Time Series Data Analysis Zur Erlangung des akademischen Grades eines Doktors der Wirtschaftswissenschaften (Dr. rer. pol.) von der Fakult¨at f¨ur Wirtschaftswissenschaften des Karlsruhe Instituts f¨ur Technologie (KIT) genehmigte Dissertation von Dipl.-Math. Thomas Meinl Tag der m¨undlichen Pr¨ufung: 10. 05. 2011 Referent: Prof. Dr. Svetlozar Rachev Korreferent: Prof. Dr. Karl-Heinz Waldmann 2011 Karlsruhe To Jack. Acknowledgements This work would not have been possible without the loving support of all my family, my dog, and my ex-wife. Thank you all, I am most deeply obliged to you for being along my side over all these years. I also thank my supervisor Prof. Dr. Svetlozar Rachev and Dr. Edward Sun for their guid- ance and their invaluable input which without this thesis could not have been finished in such a short time and with such excellent results. I also thank Christof Weinhardt who provided me with the opportunity to undertake some serious research at his institute, not to mention the fun I had with all the nice colleagues. Thank you, we had some times together we will remember for sure. During the time this thesis was written the author undertook a stay abroad in Brazil as well as in Japan, which was only made feasible thanks to the highly appreciated support of the Karlsruhe House of Young Scientists. My thanks also goes to all the people there who welcomed me so heartily. Karlsruhe, May 2011 Thomas Meinl Abstract Time series analysis is still a very wide field of research from both a theoretical point of view as well as amongst practitioners. Among the very first tasks in the analysis proce- dure is the estimation of long-term trends, that is, the separation of this generally slowly evolving component from any short-term fluctuations. Usually, the trend curve, which in most cases is expected to be smooth, can be extracted by a variety of different methods. However, in many application scenarios the trend must also account for sudden changes. These sudden changes comprise of not only jumps, but also other phenomena like steep slopes and valleys. This challenge constitutes an on-going problem for traditional trend estimation methods. While established filtering techniques either fail to capture these sudden changes accurately or are sensitive to high-amplitude fluctuations, the applica- tion of parametric methods is challenging due to the generally unknown trend and the innumerable shapes that these sudden changes can assume. This thesis proposes a trend extraction approach based on wavelet methods. The new algorithm, named local linear scaling approximation (LLSA), is developed by analyzing specific wavelet coefficient step response structures and by transferring these structures onto real signals. This procedure enables the analyst to extract a trend whose smooth- ness is comparable to the output of linear filtering techniques, while at the same time capturing the details of sudden changes with arbitrary shapes, an area in which usu- ally most nonlinear filters excel. Therefore, LLSA can be seen as a novel approach to bridge the gap between linear and nonlinear filters. The algorithm was developed to be applicable on homogeneous time series without any further requirements on these, and to work with only two additional input parameters, which can also be set in a heuristic manner, yielding a directly implementable and usable method. v Moreover, the algorithm’s properties are shown, namely its computational complexity, its local linearity, and its impulse and step response. The robustness of LLSA is first shown analytically, and then substantiated by several analyses performed on simulated signals as well as on empirical data. LLSA’s performance is further evaluated in two separate application scenarios, that are, price volatility estimation and value at risk. The algorithm’s superior performance in relation to two benchmark filtering techniques is shown for a considerable number of cases, and several aspects (i. e., possibilities and limitations) of LLSA’s general application are discussed. vi Contents List of Figures xi List of Tables xiii 1. Introduction 1 1.1. Requirements and Research Questions . ..... 3 1.2. ContributionsofthisThesis . .... 5 1.3. Structure .................................... 6 2. Methods of Trend Extraction 9 2.1. Time Series Analysis and Trend Extraction . ...... 9 2.2. LinearFilters .................................. 22 2.2.1. General Formulation . 22 2.2.2. Transfer Functions: Time vs. Frequency Domain . ..... 23 2.3. NonlinearFilters ............................... 26 2.3.1. GeneralPerception. 26 2.3.2. FilterExamples............................. 30 2.4. FurtherRelatedMethods . 34 2.4.1. Algorithms for Jump Detection and Modeling . 35 2.4.2. Alternative Methods for Trend Estimation . ..... 37 2.5. Summary .................................... 42 3. Wavelets and Their Transforms 45 3.1. Wavelets..................................... 45 3.2. WaveletTransforms .............................. 51 vii Contents 3.3. Wavelet Trend Extraction and Denoising Methods . ....... 62 3.4. Summary .................................... 66 4. The Local Linear Scaling Approximation 67 4.1. Methodology and Implementation . 67 4.1.1. Derivation................................ 67 4.1.2. Final Formulation and Remarks . 75 4.1.3. Implementation and Usage . 78 4.2. Properties.................................... 81 4.2.1. Computational Complexity . 81 4.2.2. LocalLinearity ............................. 81 4.2.3. ImpulseandStepResponse . 83 4.3. Summary .................................... 84 5. Evaluation and Application 87 5.1. RobustnessandPerformanceStudies . ..... 87 5.1.1. Analytical Consistency . 88 5.1.2. SimulatedSignals. .. .. .. .. .. .. .. .. 90 5.1.3. EmpiricalResults. .. .. .. .. .. .. .. .. 97 5.2. Applications................................... 102 5.2.1. General Application and Examples . 102 5.2.2. Price Volatility Estimation . 110 5.2.3. Estimating Value at Risk of High-Frequency Data . 114 5.3. Summary ....................................116 6. Conclusion and Outlook 119 6.1. Summary ....................................119 6.1.1. Requirement Satisfaction and Research Questions . .......119 6.1.2. Contributions. .. .. .. .. .. .. .. .. ..124 6.2. FutureResearchDirections . 125 6.2.1. AlgorithmicExtensions . 125 6.2.2. FurtherApplications . 129 viii Contents A. Empirical Robustness Study 133 A.1. Statistical Ratios Tables . 133 A.2. Bootstrap Confidence Interval Tables . 142 B. Empirical One-step-ahead Forecasting 151 B.1. Conditional Mean One-step-ahead Forecasting . .........151 B.2. EmpiricalPercentiles. 158 Bibliography 169 ix List of Figures 1.1. Structure .................................... 7 2.1. Hourly Wikipedia server requests and filtered trends . .......... 30 3.1. Haarsquaredgainfunctions . 60 3.2. D4squaredgainfunctions. 60 4.1. Wavelet coefficient structure for step functions . ......... 73 4.2. LLSA(Haar & D4) step response functions, K =1 ............. 85 4.3. LLSA(Haar) impulse response with different K ............... 85 4.4. LLSA(D4) impulse response with different K ................ 86 5.1. Robustness test signal with different noise levels . ......... 92 5.2. MAE/MSE for the Haar wavelet with 5 jumps and σ =1. ......... 95 5.3. MAE/MSE variances for the Haar wavelet with 5 jumps and σ = 1. 95 5.4. Wikipedia refinement using the Haar wavelet . 105 5.5. Wikipedia refinement with D4wavelets ...................106 5.6. Wikipedia refinement with LA8wavelets.. .. .. .. .. ..106 5.7. Filtered trend of the SAP stock data . 107 5.8. LLSA(Haar) filtered trend of the SAP stock data . 108 5.9. LLSA(D4) filtered trend of the SAP stock data . 108 5.10. LLSA(Haar and D4) filtered trend of the SAP stock data, K =3 . ..109 5.11. SAP stock price percentile deviations, K =2. ................114 6.1. Flow diagram of wavelet transforms . 126 xi List of Figures 6.2. Two-dimensional response structures . .......130 6.3. Furtherapplications . 131 xii List of Tables 2.1. Today’s algorithms’ requirement fulfillment . ......... 43 wvlt 4.1. nα, nβ and L for different wavelets . 72 4.2. LLSAdefaultinputparameters . 79 5.1. MSEmeanandvariance,5jumps. 94 5.2. MAEmeanandvariance,5jumps . 94 5.3. MSE mean and variance, 10 jumps, σ =1 .................. 96 5.4. MAE mean and variance, 10 jumps, σ =1.................. 97 5.5. LLSA inferior performance amount in percentile exceedances . 113 5.6. LLSA inferior performance amount in VaR and ES estimation . 117 6.1. Today’s algorithms’ and LLSA’s requirement fulfillment ..........122 A.1. Kolmogorov-Smirnov distance statistics, κ =4 ...............134 A.2. Anderson-Darling distance statistics, κ =4 .................135 A.3. Kuiper distance statistics, κ =4 .......................136 A.4. Cram´er-von Mises distance statistics, κ =4 .................137 A.5. Kolmogorov-Smirnov distance statistics, κ =10...............138 A.6. Anderson-Darling distance statistics, κ =10.................139 A.7. Kuiper distance statistics, κ =10.......................140 A.8. Cram´er-von Mises distance statistics, κ =10.................141 A.9. Mean difference bootstrap confidence intervals, κ =4) ...........143 A.10.Mean difference bootstrap confidence intervals, κ =5) ...........144 A.11.Mean difference bootstrap confidence intervals, κ =6) ...........145 xiii List of Tables A.12.Mean difference bootstrap confidence intervals, κ =7) ...........146 A.13.Mean difference bootstrap confidence intervals, κ =8) ...........147 A.14.Mean difference bootstrap confidence intervals, κ =9) ...........148
Details
-
File Typepdf
-
Upload Time-
-
Content LanguagesEnglish
-
Upload UserAnonymous/Not logged-in
-
File Pages197 Page
-
File Size-