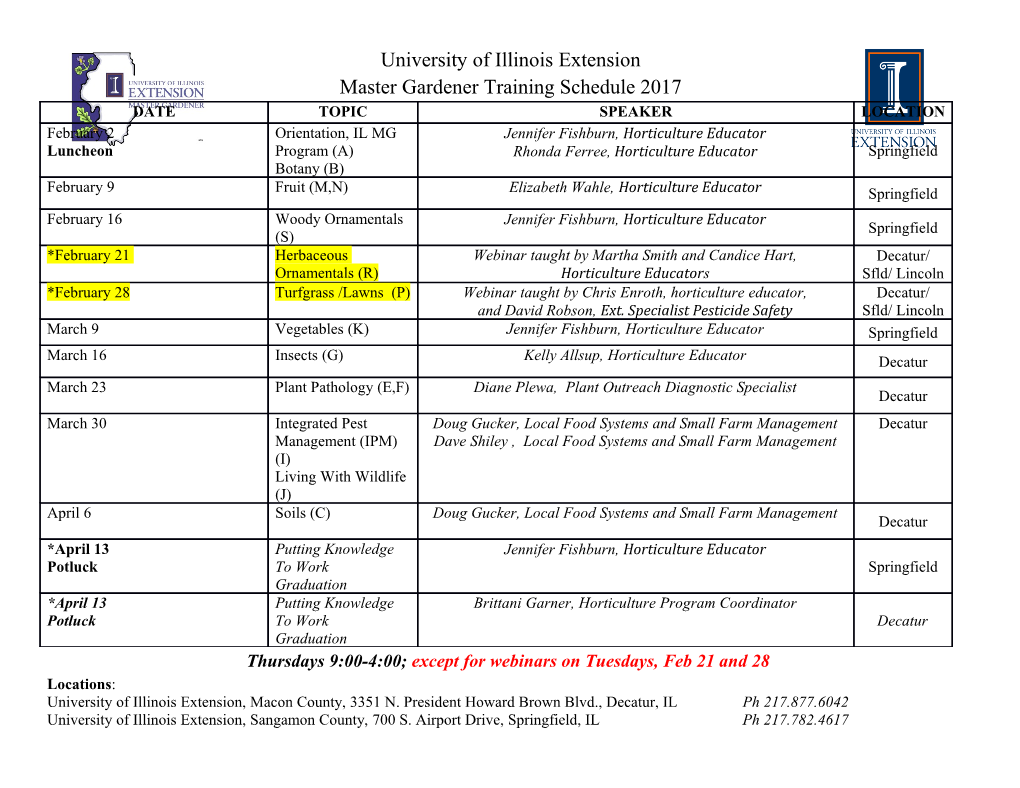
DM534 Arthur Zimek Color Histograms as Feature Spaces for Representation of Images A First Glimpse on Clustering References Introduction to Computer Science Arthur Zimek University of Southern Denmark DM534, fall 2020 1 Outline DM534 Arthur Zimek Color Histograms as Color Histograms as Feature Spaces for Representation of Images Feature Spaces for Representation of Images A First Glimpse on A First Glimpse on Clustering Clustering References 2 Outline DM534 Arthur Zimek Color Histograms as Color Histograms as Feature Spaces for Representation of Images Feature Spaces for Representation of Distances Images Distances Features for Images Features for Images Summary Summary A First Glimpse on Clustering A First Glimpse on Clustering References 3 Outline DM534 Arthur Zimek Color Histograms as Color Histograms as Feature Spaces for Representation of Images Feature Spaces for Representation of Distances Images Distances Features for Images Features for Images Summary Summary A First Glimpse on Clustering A First Glimpse on Clustering References 4 Similarity DM534 Arthur Zimek I Similarity (as given by some distance measure) is a Color Histograms as Feature Spaces for central concept in data mining, e.g.: Representation of Images Distances I clustering: group similar objects in the same cluster, Features for Images separate dissimilar objects to different clusters Summary A First Glimpse on I outlier detection: identify objects that are dissimilar (by Clustering some characteristic) from most other objects References I definition of a suitable distance measure is often crucial for deriving a meaningful solution in the data mining task I images I CAD objects I proteins I texts ... 5 I Spaces and Distance Functions DM534 Arthur Zimek Common distance measure for (Euclidean) feature vectors: Color Histograms as Feature Spaces for LP-norm Representation of Images 1 Distances P P P P Features for Images distP(p; q) = jp1 − q1j + jp2 − q2j + ::: + jpn − qnj Summary A First Glimpse on Clustering References Euclidean norm Manhattan norm Maximum norm (L2): (L1): (L1, also: Lmax, supremum dist., Chebyshev dist.) 6 Spaces and Distance Functions DM534 Arthur Zimek weighted Euclidean norm: quadratic form*: Color Histograms as 2 Feature Spaces for dist(p; q) = w1jp1 − q1j + dist(p; q) = Representation of 1 1 Images T 2 2 2 2 Distances w2jp2 − q2j + ::: + wnjpn − qnj ((p − q)M(p − q) ) Features for Images Summary A First Glimpse on Clustering References *note that we assume vectors to be row vectors here 7 Outline DM534 Arthur Zimek Color Histograms as Color Histograms as Feature Spaces for Representation of Images Feature Spaces for Representation of Distances Images Distances Features for Images Features for Images Summary Summary A First Glimpse on Clustering A First Glimpse on Clustering References 8 Categories of Feature Descriptors for Images DM534 Arthur Zimek Color Histograms as I distribution of colors Feature Spaces for Representation of Images I texture Distances Features for Images I shapes (contoures) Summary A First Glimpse on Clustering References 9 Color Histogram DM534 Arthur Zimek Color Histograms as Feature Spaces for Representation of Images Distances Features for Images Summary A First Glimpse on Clustering References I a histogram represents the distribution of colors over the pixels of an image I definition of an color histogram: I choose a color space (RGB, HSV, HLS, . ) I choose number of representants (sample points) in the color space I possibly normalization (to account for different image sizes) 10 Color Space Example: RGB cube DM534 Arthur Zimek Color Histograms as Feature Spaces for Representation of Images Distances Features for Images Summary A First Glimpse on Clustering References 11 Impact of Number of Representants DM534 Arthur Zimek original images in full RGB space (2563 = 16; 777; 216) Color Histograms as Feature Spaces for Representation of Images Distances Features for Images Summary A First Glimpse on Clustering References 12 Impact of Number of Representants DM534 Arthur Zimek Color Histograms as Feature Spaces for Representation of Images Distances Features for Images Summary A First Glimpse on Clustering References 23 33 43 163 13 Impact of Number of Representants DM534 Arthur Zimek Color Histograms as Feature Spaces for Representation of Images Distances Features for Images Summary A First Glimpse on Clustering References 23 33 43 163 14 Impact of Number of Representants DM534 Arthur Zimek Color Histograms as Feature Spaces for Representation of Images Distances Features for Images Summary A First Glimpse on Clustering References 23 33 43 163 15 Impact of Number of Representants DM534 Arthur Zimek Color Histograms as Feature Spaces for Representation of Images Distances Features for Images Summary A First Glimpse on Clustering References 23 33 43 163 16 Impact of Number of Representants DM534 Arthur Zimek Color Histograms as Feature Spaces for Representation of Images Distances Features for Images Summary A First Glimpse on Clustering References The histogram for each image is essentially a visualization of a vector: (0:77; 0; 0; 0; 0:08; 0; 0:15; 0)( 0:8; 0; 0; 0; 0:11; 0; 0:09; 0) (0:9; 0; 0; 0; 0:05; 0; 0:05; 0)( 0:955; 0; 0; 0; 0:045; 0; 0; 0) 17 Impact of Number of Representants DM534 Arthur Zimek Color Histograms as Feature Spaces for Representation of Images Distances Features for Images Summary A First Glimpse on Clustering References 17 Impact of Number of Representants DM534 Arthur Zimek Color Histograms as Feature Spaces for Representation of Images Distances Features for Images Summary A First Glimpse on Clustering References 17 Distances for Color Histograms DM534 Arthur Zimek Euclidean distance for images P and Q using the color Color Histograms as Feature Spaces for histograms hP and hQ: Representation of Images Distances q Features for Images T Summary dist(P; Q) = (hP − hQ) · (hP − hQ) A First Glimpse on Clustering References p dist(RED; PINK) = 2 p dist(RED; BLUE) = 2 p (1; 0; 0)(0; 1; 0)(0; 0; 1) dist(BLUE; PINK) = 2 A ‘psychologic’ distance would consider that red is (in our perception) more similar to pink than to blue. 18 Example for the Distance Computation of Histograms DM534 Arthur Zimek q Color Histograms as T Feature Spaces for dist(P; Q) = (h − h ) · (h − h ) Representation of P Q P Q Images Distances Features for Images Summary p T A First Glimpse on dist(RED; PINK) = ((1; 0; 0) − (0; 1; 0)) · ((1; 0; 0) − (0; 1; 0)) Clustering p References = (1; −1; 0) · (1; −1; 0)T p = (1 · 1 + (−1) · (−1) + 0 · 0) p = 2 19 Distances for Color Histograms DM534 Arthur Zimek Quadratic form with ‘psychological’ similarity matrix Color Histograms as 2 3 Feature Spaces for 1 a12 ::: Representation of Images 6a21 1 ::: 7 ? Distances A = 6 7 where a (= a ) describe the Features for Images 6 . .. .7 ij ji Summary 4 . .5 A First Glimpse on Clustering ::: 1 References subjective similarity of the features i and j in the color histogram: q T distA(P; Q) = (hP − hQ) · A · (hP − hQ) 2 3 p 1 0:9 0 dist(RED; PINK) = 0:2 A0 = 40:9 1 05 p dist(RED; BLUE) = 2 0 0 1 p dist( ; ) = 20 BLUE PINK 2 Outline DM534 Arthur Zimek Color Histograms as Color Histograms as Feature Spaces for Representation of Images Feature Spaces for Representation of Distances Images Distances Features for Images Features for Images Summary Summary A First Glimpse on Clustering A First Glimpse on Clustering References 21 Your Choice of a Distance Measure DM534 Arthur Zimek There are hundreds of distance functions [Deza and Deza, Color Histograms as Feature Spaces for 2009]. Representation of Images Distances I For time series: DTW, EDR, ERP, LCSS, . Features for Images Summary I For texts: Cosine and normalizations A First Glimpse on Clustering I For sets – based on intersection, union, . (Jaccard) References I For clusters (single-link, average-link, etc.) I For histograms: histogram intersection, “Earth movers distance”, quadratic forms with color similarity I With normalization: Canberra, . I Quadratic forms / bilinear forms: d(x; y) := xT My for some positive (usually symmetric) definite matrix M. Note that: Choosing the appropriate distance function can be seen as a part of “preprocessing”. 22 Summary DM534 Arthur Zimek Color Histograms as You learned in this section: Feature Spaces for Representation of Images I distances (Lp-norms, weighted, quadratic form) Distances Features for Images I color histograms as feature (vector) descriptors for Summary A First Glimpse on images Clustering References I impact of the granularity of color histograms on similarity measures 23 Outline DM534 Arthur Zimek Color Histograms as Color Histograms as Feature Spaces for Representation of Images Feature Spaces for Representation of Images A First Glimpse on A First Glimpse on Clustering Clustering General Purpose of General Purpose of Clustering Clustering Partitional Clustering Partitional Clustering Algorithm Algorithm Visualization: Algorithmic Visualization: Algorithmic Differences Differences Summary Summary References 24 Outline DM534 Arthur Zimek Color Histograms as Color Histograms as Feature Spaces for Representation of Images Feature Spaces for Representation of Images A First Glimpse on A First Glimpse on Clustering Clustering General Purpose of General Purpose of Clustering Clustering Partitional Clustering Partitional Clustering Algorithm Algorithm Visualization: Algorithmic Visualization: Algorithmic Differences Differences Summary Summary References 25 Purpose of Clustering DM534 Arthur Zimek Color Histograms as I identify a
Details
-
File Typepdf
-
Upload Time-
-
Content LanguagesEnglish
-
Upload UserAnonymous/Not logged-in
-
File Pages81 Page
-
File Size-