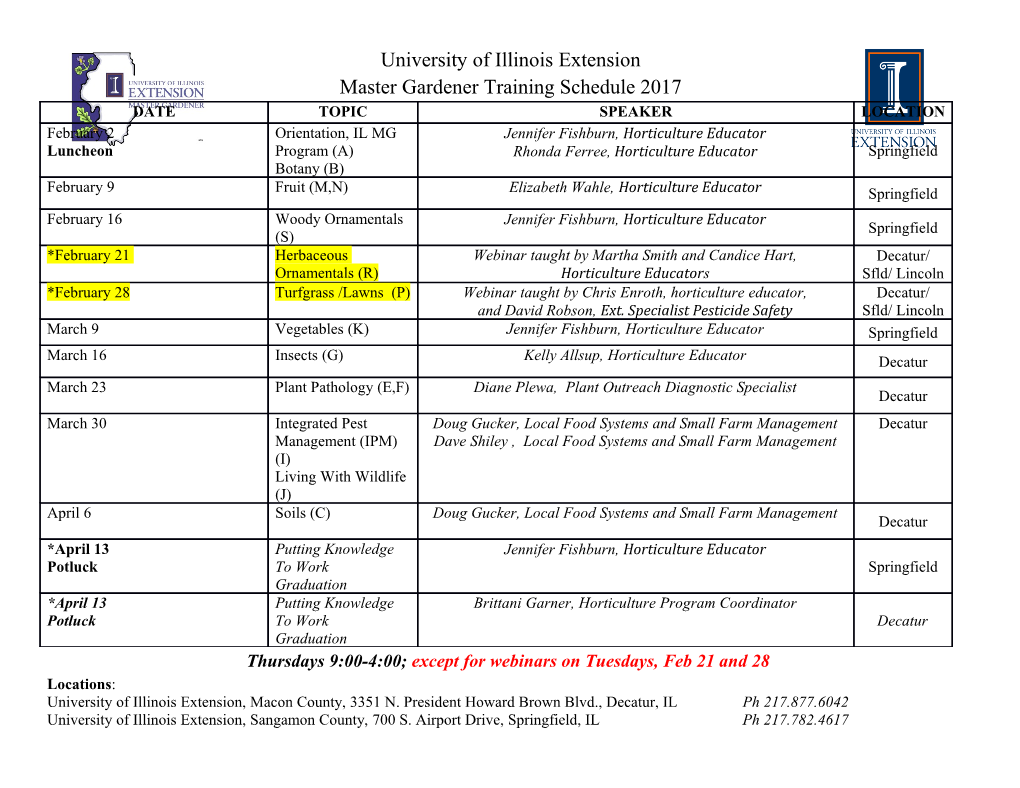
Crowdsource Annotation and Automatic Reconstruction of Online Discussion Threads Vom Fachbereich Informatik der Technischen Universität Darmstadt genehmigte Dissertation zur Erlangung des akademischen Grades Doktor der Naturwissenschaften vorgelegt von Emily K. Jamison, M.A. geboren in Minnesota, USA Tag der Einreichung: 14. December 2015 Tag der Disputation: 17. February 2016 Referenten: Prof. Dr. phil. Iryna Gurevych, Darmstadt Prof. Johannes Fürnkranz, PhD, Darmstadt Prof. Walter Daelemans, PhD, Antwerp Darmstadt 2016 D17 Please cite this document as URN: urn:nbn:de:tuda-tuprints-53850 URL: http://tuprints.ulb.tu-darmstadt.de/5385/ This document is provided by tuprints, E-Publishing-Service of the TU Darmstadt http://tuprints.ulb.tu-darmstadt.de [email protected] This work is published under the following Creative Commons license: Attribution – Non Commercial – No Derivative Works 3.0 Germany http://creativecommons.org/licenses/by-nc-nd/3.0/de/deed.en Abstract Modern communication relies on electronic messages organized in the form of discussion threads. Emails, IMs, SMS, website comments, and forums are all composed of threads, which consist of individual user messages connected by metadata and discourse coherence to mes- sages from other users. Threads are used to display user messages effectively in aGUIsuch as an email client, providing a background context for understanding a single message. Many messages are meaningless without the context provided by their thread. However, a num- ber of factors may result in missing thread structure, ranging from user mistake (replying to the wrong message), to missing metadata (some email clients do not produce/save headers that fully encapsulate thread structure; and, conversion of archived threads from over repos- itory to another may also result in lost metadata), to covert use (users may avoid metadata to render discussions difficult for third parties to understand). In the field of security, law enforcement agencies may obtain vast collections of discussion turns that require automatic thread reconstruction to understand. For example, the Enron Email Corpus, obtained by the Federal Energy Regulatory Commission during its investigation of the Enron Corporation, has no inherent thread structure. In this thesis, we will use natural language processing approaches to reconstruct threads from message content. Reconstruction based on message content sidesteps the problem of missing metadata, permitting post hoc reorganization and discussion understanding. We will investigate corpora of email threads and Wikipedia discussions. However, there is a scarcity of annotated corpora for this task. For example, the Enron Emails Corpus contains no inher- ent thread structure. Therefore, we also investigate issues faced when creating crowdsourced datasets and learning statistical models of them. Several of our findings are applicable for other natural language machine classification tasks, beyond thread reconstruction. We will divide our investigation of discussion thread reconstruction into two parts. First, we explore techniques needed to create a corpus for our thread reconstruction re- search. Like other NLP pairwise classification tasks such as Wikipedia discussion turn/edit alignment and sentence pair text similarity rating, email thread disentanglement is a heav- ily class-imbalanced problem, and although the advent of crowdsourcing has reduced anno- III tation costs, the common practice of crowdsourcing redundancy is too expensive for class- imbalanced tasks. As the first contribution of this thesis, we evaluate alternative strategies for reducing crowdsourcing annotation redundancy for class-imbalanced NLP tasks. We also examine techniques to learn the best machine classifier from our crowdsourced labels. In or- der to reduce noise in training data, most natural language crowdsourcing annotation tasks gather redundant labels and aggregate them into an integrated label, which is provided to the classifier. However, aggregation discards potentially useful information from linguistically ambiguous instances. For the second contribution of this thesis, we show that, for four of five natural language tasks, filtering of the training dataset based on crowdsource annotation item agreement improves task performance, while soft labeling based on crowdsource annotations does not improve task performance. Second, we investigate thread reconstruction as divided into the tasks of thread disen- tanglement and adjacency recognition. We present the Enron Threads Corpus, a newly-ex- tracted corpus of 70,178 multi-email threads with emails from the Enron Email Corpus. In the original Enron Emails Corpus, emails are not sorted by thread. To disentangle these threads, and as the third contribution of this thesis, we perform pairwise classification, using text similarity measures on non-quoted texts in emails. We show that i) content text similarity metrics outperform style and structure text similarity metrics in both a class-balanced and class-imbalanced setting, and ii) although feature performance is dependent on the semantic similarity of the corpus, content features are still effective even when controlling for semantic similarity. To reconstruct threads, it is also necessary to identify adjacency relations among pairs. For the forum of Wikipedia discussions, metadata is not available, and dialogue act typologies, helpful for other domains, are inapplicable. As our fourth contribution, via our experiments, we show that adjacency pair recognition can be performed using lexical pair features, without a dialogue act typology or metadata, and that this is robust to controlling for topic bias of the discussions. Yet, lexical pair features do not effectively model the lexical se- mantic relations between adjacency pairs. To model lexical semantic relations, and as our fifth contribution, we perform adjacency recognition using extracted keyphrases enhanced with se- mantically related terms. While this technique outperforms a most frequent class baseline, it fails to outperform lexical pair features or tf-idf weighted cosine similarity. Our investigation shows that this is the result of poor word sense disambiguation and poor keyphrase extraction causing spurious false positive semantic connections. Publications of the contributions are listed in Section 1.3. Figure 1.1 shows an overview of the topics of the contributions and how they are interrelated. In concluding this thesis, we also reflect on open issues and unanswered questions re- maining after our research contributions, discuss applications for thread reconstruction, and suggest some directions for future work. IV Zusammenfassung Moderne Kommunikation beruht auf elektronischen Nachrichten, die in Form von Threads or- ganisiert sind. E-Mails, Sofortnachrichten, SMS, Kommentare auf Webseiten und in Foren sind aus solchen Threads aufgebaut - diese wiederum bestehen aus einzelnen Benutzernachrichten, die mithilfe von Metadaten verbunden sind und zwischen denen Diskurskohärenz besteht. Threads werden benutzt, um Benutzernachrichten effektiv in einer GUI, wie etwa einemE- Mail-Programm, zu visualisieren. Sie stellen also einen Hintergrundkontext bereit, ohne den einzelne Nachrichten oft nicht verstanden werden können. Allerdings kann es durch eine Reihe von Faktoren dazu kommen, dass eine solche Thread-Struktur verloren geht: Ange- fangen von Benutzerfehlern (z.B. dem Antworten auf eine falsche Nachricht), über fehlende Metadaten (manche E-Mail-Programme erzeugen E-Mail-Header, die nicht die volle Thread- Struktur enthalten; auch Konvertierungen von alten Threads können in fehlenden Metadaten resultieren) bis hin zu absichtlich verschleierter Struktur (etwa durch Benutzer, die es Dritten erschweren wollen, eine Diskussion nachzuvollziehen, und dazu Metadaten vermeiden oder entfernen). Im Bereich Sicherheit benötigen Strafverfolgungsbehörden daher eine automatis- che Thread-Rekonstruktion, um große Mengen an gesammelten elektronischen Nachrichten aus Diskussionen verstehen zu können. Beispielsweise besitzt das Enron Email Corpus, das von der Federal Energy Regulatory Commission der USA während der Ermittlungen beim Energiekonzern Enron zusammengetragen wurde, keine inhärente Thread-Struktur. In dieser Arbeit verwenden wir Ansätze aus der maschinellen Sprachverarbeitung (Natu- ral Language Processing, NLP), um Threads aus Nachrichteninhalten zu rekonstruieren. Eine solche Rekonstruktion basierend auf den Inhalten umgeht das Problem fehlender Metadaten und erlaubt eine nachträgliche Restrukturierung und damit auch ein Verstehen der gesamten Diskussion. Wir untersuchen Korpora bestehend aus E-Mail-Threads und Wikipedia-Dis- kussionen. Allerdings herrscht eine Knappheit an geeigneten, annotierten Korpora. Zum Beispiel enthält das Enron Emails Corpus keine Angaben zur Thread-Struktur. Aus diesem Grund erforschen wir außerdem Probleme, die beim Erstellen von crowdgesourcten Daten- sätzen und beim Trainieren maschineller Lernverfahren auf solchen Datensätzen auftreten. V Viele unserer Ergebnisse sind daher über die Thread-Rekonstruktion hinaus auch auf andere automatische Klassifizierungsaufgaben für natürliche Sprache anwendbar. Wir gliedern unsere Erforschung der Rekonstruktion von Diskussions-Threads in zwei Teile auf. Zuerst untersuchen wir Methoden für die Erstellung eines Korpus, das der Forschung an Thread-Rekonstruktion dienen soll. Wie andere Problemstellungen im Bereich paarweiser Klassifikation in NLP, etwa die Textähnlichkeitsbewertung für Satzpaare oder
Details
-
File Typepdf
-
Upload Time-
-
Content LanguagesEnglish
-
Upload UserAnonymous/Not logged-in
-
File Pages238 Page
-
File Size-