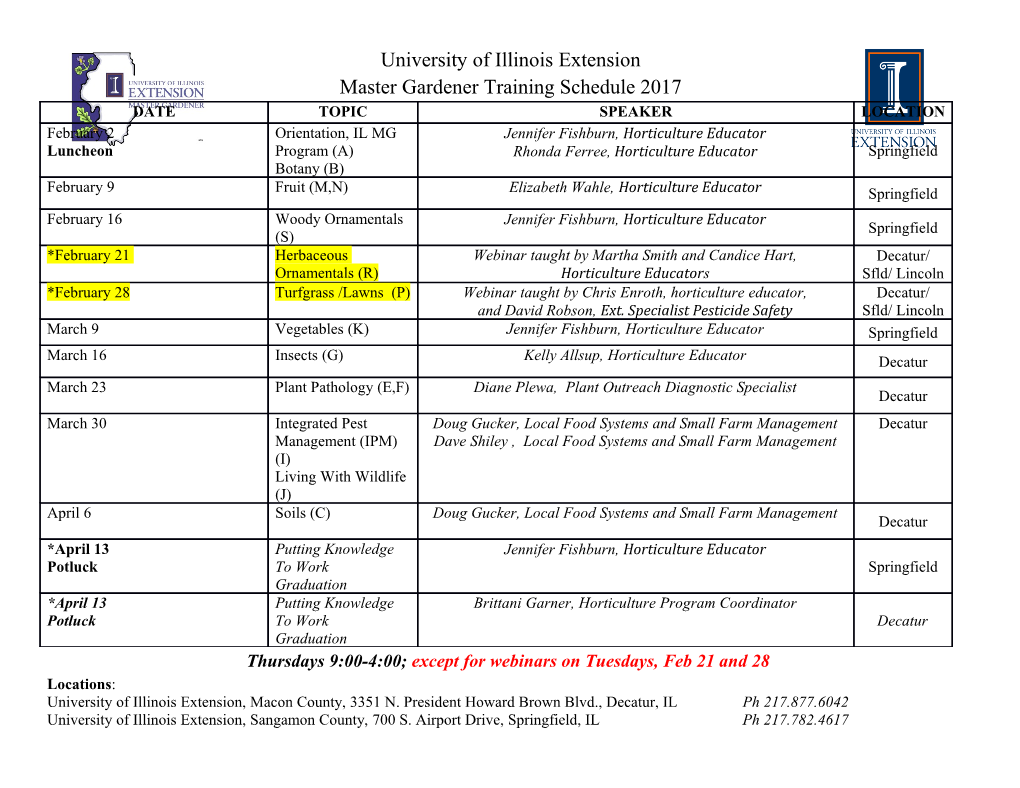
University of Warsaw Faculty of Mathematics, Informatics and Mechanics Arkadiusz Socała Lower Bounds Under Strong Complexity Assumptions PhD dissertation Supervisor dr hab. Łukasz Kowalik Institute of Informatics University of Warsaw May 2017 Author’s declaration: I hereby declare that this dissertation is my own work. May 20, 2017 . Arkadiusz Socała Supervisor’s declaration: The dissertation is ready to be reviewed May 20, 2017 . dr hab. Łukasz Kowalik Abstract This work is devoted to lower bounds on running time under strong complexity assumptions. We prove that under the Exponential Time Hypothesis there is no algorithm working in time • 2o(n log n) (times a polynomial in the bit size) for Channel Assignment, p • 2o(n log n) for Subgraph Isomorphism, 3=2 • 2o(n ) for Rainbow k-Coloring (for any k ≥ 2), • f(b) · 2o(log b)·n for (a:b)-coloring (for any computable f(b)), • O∗(2o(d log d)) for Minimax Approval Voting. This in particular exclude the existence of algorithms solving these problems in time O∗(cn) (or O∗(cd) in the case of Minimax Approval Voting) for any constant c. Moreover in the cases of Channel Assignment, (a:b)-coloring and Mini- max Approval Voting our lower bounds are tight, showing that currently known algorithms are optimal (unless ETH fails). We also give a lower bound of a different flavor. We show that improving over the best known algorithm for 4-OPT Detection (a problem related to Traveling Salesman Problem), would imply an improvement for All Pairs Shortest Paths. Keywords: Lower Bounds, Fine Grained Complexity, Exponential Time Hypothesis. ACM Classification: F.2.2. 2 Contents 1 Introduction5 1.1 Organization of the dissertation.....................8 1.2 Considered problems...........................8 1.2.1 Channel Assignment .....................8 1.2.2 Subgraph Isomorphism .................... 10 1.2.3 Rainbow k-Coloring ..................... 12 1.2.4 Multicoloring and low degree monomial testing........ 13 1.2.5 Minimax Approval Voting ................. 17 1.2.6 k-OPT Detection ....................... 20 1.3 Articles.................................. 22 1.4 Acknowledgements............................ 23 2 Notation and Preliminaries 25 2.1 Notation.................................. 25 2.2 Exponential-Time Hypothesis toolbox.................. 25 2.2.1 Reducing from 3-CNF-SAT ................... 25 2.2.2 Reducing from other problems.................. 26 3 Channel Assignment 29 3.1 Hardness of Equal Weight Matchings ............... 29 3.1.1 From 3-CNF-SAT to Family Intersection.......... 29 3.1.2 From Family Intersection to Equal Weight Matchings. 31 3.2 Hardness of Channel Assignment .................. 38 4 Subgraph Isomorphism 49 4.1 Overview of the reduction........................ 49 4.2 Grouping clauses............................. 51 4.3 From (3;4)-CNF-SAT to Subgraph Isomorphism with colors... 52 4.4 Removing the colors........................... 56 4.5 From Graph Homomorphism to Subgraph Isomorphism .... 60 5 Rainbow Coloring 61 5.1 Overview.................................. 61 5.2 From (3;4)-CNF-SAT to Subset Rainbow k-Coloring ...... 63 5.3 From Subset Rainbow k-Coloring to Rainbow k-Coloring .. 74 3 5.4 Putting everything together....................... 79 6 Multicoloring and low-degree monomial testing 81 6.1 Preliminaries............................... 81 6.2 Hardness of List (a:b)-coloring .................... 83 6.2.1 The nonuniform case....................... 84 6.2.2 The uniform case......................... 88 6.3 From List (a:b)-coloring to (a:b)-coloring ............ 89 6.4 Low-degree testing............................ 91 7 Minimax Approval Voting 97 7.1 Reduction from (3;4)-CNF-SAT ..................... 97 7.2 Reduction from k × k-Clique ...................... 100 8 4-OPT Detection for Traveling Salesman Problem 103 9 Further Work 107 A Problem Definitions 119 4 Chapter 1 Introduction We would like to be able to quickly solve combinatorial problems that appear in theory or in the real life. However for many of them we do not know efficient algorithms. Therefore the question arises: is it because it is generally impossible or maybe just we do not know appropriate tools yet? In order to answer this question we need to understand the reason of the hardness of the particular problem. One approach to investigate it is to reduce another, perhaps more familiar, problem to our target problem. Such a reduction shows that the target problem has enough expressive power to capture the hardness of the source problem. If the reduced instance is concise enough we can usually derive interesting conclusions about the dependency between the time complexities of these two problems. This approach is commonly used for NP-hard problems. For example, one can reduce an n-variable instance of 3-CNF-SAT to an equivalent nO(1)-vertex instance of 3-Coloring and the reduction algorithm works in polynomial time. There are two ways of looking at the consequences of this reduction. First, it means that if P 6= NP then 3-Coloring has no polynomial time algorithm. However, even if we do not believe that P 6= NP the reduction carries an important message: instead of working on a polynomial time algorithm for 3-Coloring one should rather focus on the structurally simpler 3-CNF-SAT. In this thesis we investigate reductions that are more constrained than the classic polynomial reductions. For example one can observe that the classic reduction from 3-CNF-SAT to 3-Coloring transforms an input formula ' into an O(j'j)-vertex graph, i.e., the size of the output instance is not only polynomial but even linear. This means that a 2o(n)-algorithm for 3-Coloring implies a 2o(j'j) algorithm for 3-CNF-SAT. Again we can interpret this in two ways. First, it is a hint for the researchers to study rather 3-CNF-SAT directly than try to improve the algorithm for 3-Coloring, that captures the full power of 3-CNF-SAT in more convoluted way. On the other hand, if one believes that no 2o(j'j) algorithm for 3-CNF-SAT exists it means there is no hope for 2o(n) algorithm for 3-Coloring. This belief can be captured as a hypothesis, which strengthens P 6= NP; and says that 3-CNF-SAT has no 2o(j'j)-time algorithm. Actually, Impagliazzo, Paturi and Zane [76, 77] show that it is equivalent to assume a slightly weaker claim as follows. 5 Hypothesis 1 (Exponential Time Hypothesis (ETH) [76]). There exists a constant c > 0, such that there is no deterministic algorithm solving 3-CNF-SAT in time O∗(2cn).1 By now ETH became one of standard assumptions for proving lower bounds (see [102] for a survey). As discussed above, a linear reduction from 3-CNF-SAT to 3-Coloring shows that the known O(1:33n)-time algorithm for 3-Coloring [10] is essentially optimal (up to constants). The same situation holds for many NP-complete graph problems, i.e., we know algorithms running in time 2O(n); and this is tight under ETH. This is obviously the case for problems asking for a set of vertices, like Clique or Vertex Cover, or more generally, for problems which admit polynomially (or even subexpo- nentially) checkable O(n)-bit certificates. But there are 2O(n)-time algorithms also for some problems for which such certificates are not known, including e.g., Hamiltonic- ity [71] and Graph Coloring [90]. However, for some problems 2O(n) algorithms are not known, for example the Channel Assignment problem, which is a gener- alization of Graph Coloring, where every edge uv of the graph comes with integer weight w; which means that the colors of u and v must differ by at least w: The best known algorithm for Channel Assignment works in 2O(n log n) time. How can we prove it is tight? For example this thesis contains a reduction from 3-CNF-SAT to Channel As- signment which transforms an instance of 3-CNF-SAT with n variables (and O(n) clauses, which will be explained later) into an instance of Channel Assignment of size O(n= log n). This compression is an important feature of our reduction and it is different than in the case of many classic reductions for showing NP-hardness. As a corollary, a hypothetical 2o(n log n)-time algorithm for Channel Assignment would imply a 2o(n)-time algorithm for 3-CNF-SAT. If we assume the Exponential Time Hypothesis (ETH) then we obtain that Channel Assignment cannot be solved in time 2o(n log n) and the currently known algorithm is essentially optimal. It is worth to note that even though our reduction works in polynomial time it could work as well in arbitrarily large time complexity that is in 2o(n) and still composed with a 2o(n log n)-time algorithm for Channel Assignment it would solve 3-CNF-SAT in time 2o(n). In this thesis we mostly focus on such instance-compressing reductions which exclude 2O(n) algorithms. By studying these dependencies we try to discover the underlying structure of hardness among NP-complete problems. This young subfield, living on the border of algorithmics and complexity theory, is known under the name of Fine Grained Complexity. Let us mention also a stronger version of ETH called Strong Exponential Time Hypothesis (SETH) [76, 77] that says that for every " < 1 there exists k > 3 such that k-CNF-SAT cannot be solved in time O∗(2n): Indeed, an algorithm refuting even this stronger version would be a breakthrough. SETH can be useful for example if one cares about the multiplicative constant in the exponent, i.e., if one’s goal is to 1The O∗ notation suppresses factors polynomial in the input size. 6 prove a lower bound for some problem that would be of the form Ω∗(2cn) for some particular value of c > 0.
Details
-
File Typepdf
-
Upload Time-
-
Content LanguagesEnglish
-
Upload UserAnonymous/Not logged-in
-
File Pages126 Page
-
File Size-