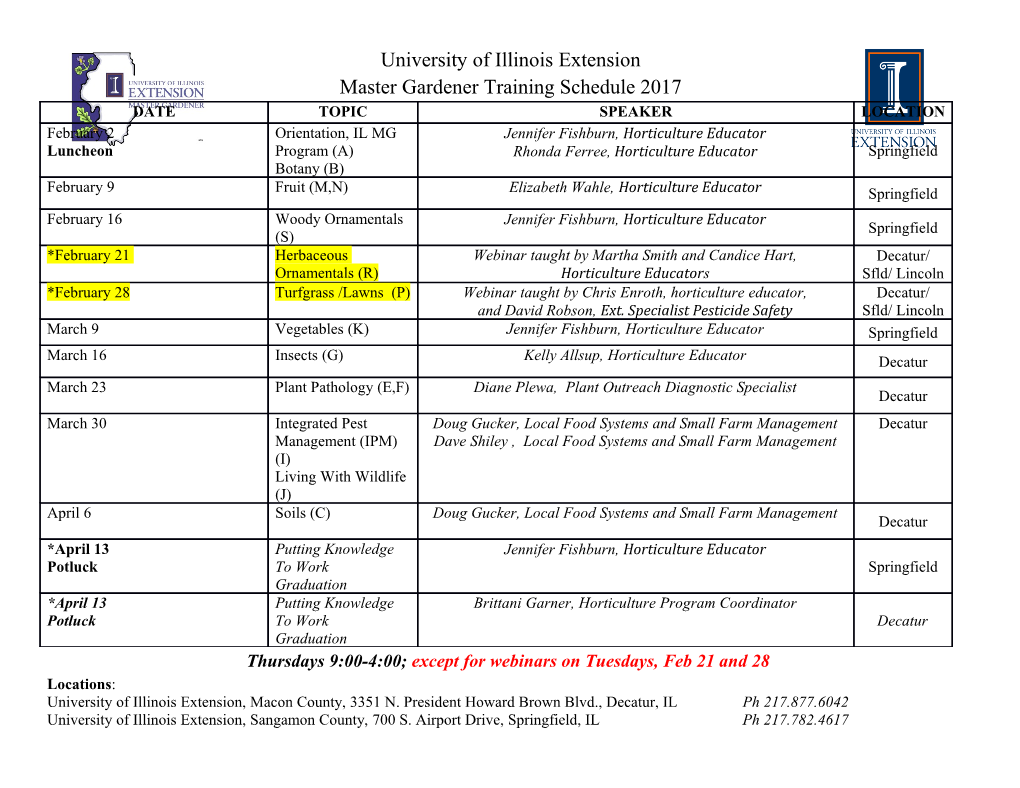
¡ ¢ Physics Letters A 9909 2000 xxx ¥ ¤ www.elsevier.nl£ locate pla Localized Lyapunov exponents and the prediction of predictability ¦ Christine Ziehmann a, § , Leonard A. Smith a,b,c, JurgenÈ Kurths a ¨ a Uni© ersitat Potsdam, Institut fur Physik, Nichtlineare Dynamik, Postfach 60 15 53, D-14469, Potsdam, Germany ÈÈ b Mathematical Institute,Uni ersity of Oxford, Oxford, OX1 3LB, UK c London School of Economics, London WC2A 2AE, UK Received 9 December 1999; received in revised form 19 April 2000; accepted 3 May 2000 Communicated by C.R. Doering Abstract Every forecast should include an estimate of its likely accuracy, a current measure of predictability. Two distinct types of localized Lyapunov exponents based on infinitesimal uncertainty dynamics are investigated to reflect this predictability. Regions of high predictability within which any initial uncertainty will decrease are proven to exist in two common chaotic systems; potential implications of these regions are considered. The relevance of these results for finite size uncertainties is discussed and illustrated numerically. 2000 Elsevier Science B.V. All rights reserved. PACS: 05.45.-a; 05.10.-a; 05.45.Tp Keywords: Lyapunov exponents; Predictability; Chaos; Weather forecasting; Ensemble prediction; Nonlinear dynamics 1. Introduction ! difficulty in quantifying predictability: 1 the depen- ! dence of measures of predictability upon the particu- " # $ lar metric adopted 2 the dependence of uncertainty Prediction of predictability refers to the quantita- ! dynamics upon the magnitude of the uncertainty in % & tive attempt to assess the likely error in a particular the initial condition, and 3 the fact that errors in the forecast a priori. There are at least three sources of model are often unknown until after predictions are ' ( ) observed to fail. Lyapunov exponents 1±3 quantify * predictability through globally averaged effective + growth rates of uncertainty in the limits of large time , and small uncertainty; thus by construction they are ) of limited use. To obtain a quantitative estimate of Corresponding author. Tel.: 49-331-977-1302, fax: 49- the accuracy of a particular forecast, the local dy- 331-977-1142. E-mail address: [email protected] C. Ziehmann . - namics of uncertainties about that initial condition / 0 0375-9601. 00 $ - see front matter 2000 Elsevier Science B.V. All rights reserved. 2 PII: S0375-9601 1 00 00336-4 2 C. Ziehmann et al. 3 Physics Letters A 00 ()2000 000±000 4 5 , are more relevant 4±9 . By allowing better risk not considered in this paper as there is no systematic , D D assessment, a prediction of predictability is of value manner to include system H model mismatches, thus it ? in 6 any field from physics to economics; weather is assumed throughout the paper that the perfect forecasting provides a particular example relevant to model is known. 7 both fields. + Effecti 8 e growth rates defined over a fixed dura- tion are also used to quantify predictability; they are I 9 employed daily in the operational weather forecast 2. Localized Lyapunov exponents : ; < centers of Europe and North America 10,11 . In Section 2, the distinction between what will be called The dynamics of infinitesimal uncertainties about , J finite time exponents and finite sample exponents is K L M N = a point x0 in an m-dimensional state space are > J shown to lie in the particular initial orientation of + L O P Q > 9 governed by the linear propagator, x0 , t , which U T the perturbation each considers for a given initial 9 m L R S < evolves any infinitesimal initial uncertainty 0 R > J , condition; this can result in dramatically different , L V + 8 8 about x0 forward for a time t along the system's effecti e growth rates. Both types of exponent are J W < trajectory to x : called `Local Lyapunov exponents', and recognizing t ^ _ ` a > ] J X Y Z [ b the distinction between them resolves some confu- L t x, \ t 0 .1 sion in the literature. In terms of predicting the d forecast accuracy, the finite time exponents are shown c In discrete time maps, the linear propagator over k to be the more relevant quantities in Section 3, where ? iterations is simply the product of Jacobians along e f g h d k l m J J n o L j it is also proven that the mean of the largest finite i the trajectory, that is x0 ,k xk 1 ... p q r s t u v w J time exponent does not provide an unbiased estimate J x10x . x ) of the corresponding global exponent, and similarly For high dimensional systems, interest tends to be for the mean of the smallest finite time exponent. In focused on subspaces which are likely to contain the y z , addition, in regions of state space where the largest fastest growing perturbations 4,10,11 . Two orienta- { | * 6 finite time exponent is less than zero all perturba- tions of particular interest are i that which will tions will shrink independent of their orientation; this } have grown the most under the linearized dynamics ? ~ n d J is investigated in Section 4 where such regions are , after k steps, k x , and ii the local orientation of 1 * proven to exist in two common chaotic maps. the globally fastest growing direction, lx, which 1 Each class of Lyapunov exponent discussed in is sometimes called the Lyapunov vector 13 . The this paper assumes the observational uncertainty is first of these orientations is defined by the singular ? infinitesimal; of course as long as it remains in- B value decomposition 14 of the propagator: the n , finitesimal it cannot limit predictability, and once it k J x are simply the right singular vectors of i d is finite its growth is no longer quantified by Lya- J x,k . Each is associated with a singular value, n * J k punov exponents. Therefore, the rigorous results re- x ; by convention, . The finite time iii 1 stricted to infinitesimal uncertainties are contrasted Lyapuno 8 exponents 15 are @ with numerical demonstrations for finite uncertain- ° ± n ¡ ¢ n £ ¤ ¤ ¥ ¥ ª « ¬ ­ ® ¯ $ ¨ © d ties in Section 4. Part of the popularity of global ¦ 1 § J J k J k Lyapunov exponents stems from the fact that their i x d log 2 x,k i x A k B value does not depend upon the metric or coordinate C ² º » n ³ · ¸ ¹ system used; this is not the case for the exponents 1 ¶ J ´ 7 k ¼ µ d log x .2 based upon a finite length of trajectory, yet in prac- k 2 i tice only the latter are available. We return to this issue in Section 5. Finally, there is the question of ½ Properties of these exponents have been noted by ¾ ¿ À Á Â Ã Ä D model error in nonlinear forecasting, either paramet- Lorenz 4 , Grassberger et al. 16 , Abarbanel 5 and E F Å Æ G ric or structural 12 . Arguably, model error may be references thereof. By Oseledec's Theorem 1 , in Ç n È É Ê d Ë Ì Í < k J more responsible for poor predictions of real nonlin- the limit k the i x converge to a unique set 9 ) ear systems than `chaos.' Model imperfections are of values, the Lyapunov exponents Î i, which are the C. Ziehmann et al. Ï Physics Letters A 00 ()2000 000±000 3 n c d J @ a b , d , k same for almost all x with respect to an ergodic and 11approach , yet for the relatively small k measure. ) over which forecasts are typically made their proper- ties are quite different and neither is constrained by Ú Û Ð Ñ Ò Ó n Ô Õ Ö × Ø $ 1 k Ù e lim d log , the value of . Ý Þ ii Ü 2 i 1 k k à á K i ß 1,2,...,m 3 f 3. Properties of localized Lyapunov exponents If the sum of the â i is negative, a volume 9 element in state space will shrink, on average, as it g 9 evolves along a trajectory, and most initial condi- General constraints on the relative magnitudes of tions will evolve towards an attractor of dimension the largest and the smallest finite time and finite ã ä J ) less than K m 17 . At each point x on such an sample exponents are now derived from the defini- å æ ! , attractor, the orientation lxdenotes the orienta- tions above, and then illustrated below. By construc- 1 tion, maximum growth corresponds to , thus ç n i j k l n m n o tion corresponding to 1, that is the orientation h 1 J q r J J p ? k x k x for each x, and therefore this in- s t è u v towards which almost every uncertainty in the 11 n 9 k x equality also holds for the mean values w { | } ~ n 1 z sufficiently distant past would have evolved, when y ! é ê k J , , where denotes an arithmetic average U the trajectory reaches x. Similarly, define lxas 1 ¼ m ë 2 taken with respect to the natural measure , and U the orientation corresponding to ì for details, see N m í î ï ð ñ ò ó , , < L a numerical approximation with sample size N.Ex- 17 . Numerically, lx10and lxU m 0 can be approx- , amples from two chaotic systems are given in Fig. 1. imated by evolving the singular vectors of ô õ ö ÷ ø ù ù J ú û ý ü The Henon map 24 is x j ,2 j , that is, the 2 j step propagator about ù ù ¼ * J th þ L 6 the j pre-image of x , forward j steps until the 2 0 x 1 ax y , y bx ,6 i 1 iii1 i J L trajectory reaches x .
Details
-
File Typepdf
-
Upload Time-
-
Content LanguagesEnglish
-
Upload UserAnonymous/Not logged-in
-
File Pages15 Page
-
File Size-