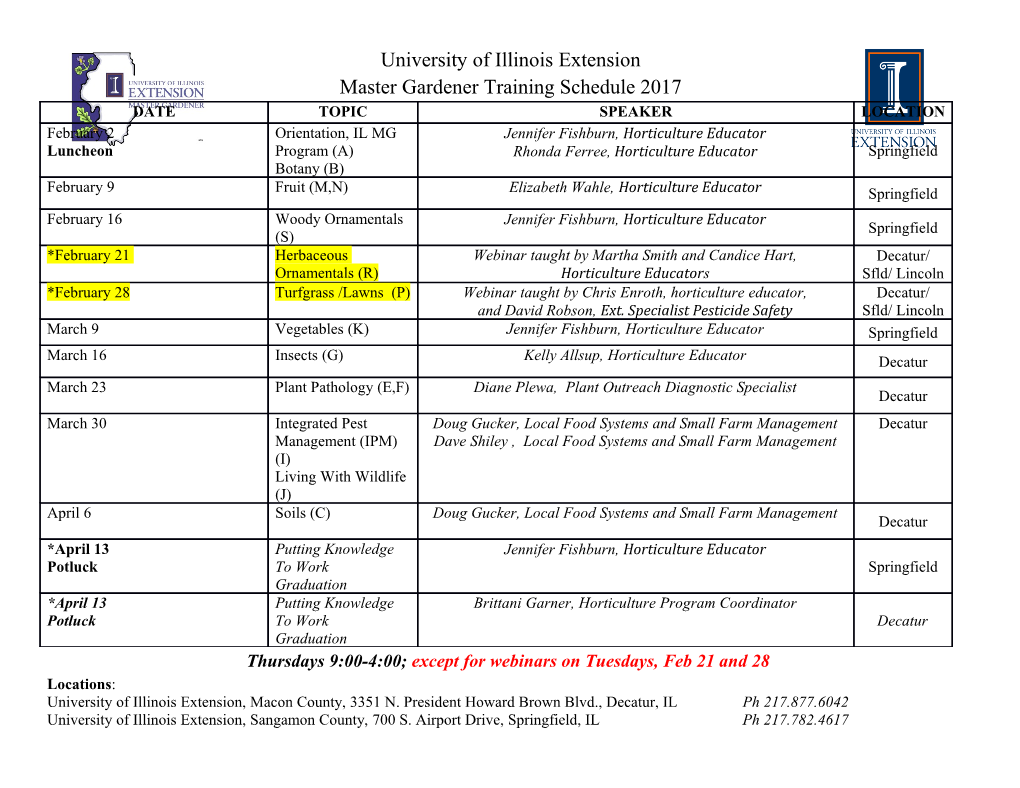
Stochastic Time-Series Spectroscopy John Scoville1 San Jose State University, Dept. of Physics, San Jose, CA 95192-0106, USA Spectroscopically measuring low levels of non-equilibrium phenomena (e.g. emission in the presence of a large thermal background) can be problematic due to an unfavorable signal-to-noise ratio. An approach is presented to use time-series spectroscopy to separate non-equilibrium quantities from slowly varying equilibria. A stochastic process associated with the non-equilibrium part of the spectrum is characterized in terms of its central moments or cumulants, which may vary over time. This parameterization encodes information about the non-equilibrium behavior of the system. Stochastic time-series spectroscopy (STSS) can be implemented at very little expense in many settings since a series of scans are typically recorded in order to generate a low-noise averaged spectrum. Higher moments or cumulants may be readily calculated from this series, enabling the observation of quantities that would be difficult or impossible to determine from an average spectrum or from prinicipal components analysis (PCA). This method is more scalable than PCA, having linear time complexity, yet it can produce comparable or superior results, as shown in example applications. One example compares an STSS-derived CO2 bending mode to a standard reference spectrum and the result of PCA. A second example shows that STSS can reveal conditions of stress in rocks, a scenario where traditional methods such as PCA are inadequate. This allows spectral lines and non-equilibrium behavior to be precisely resolved. A relationship between 2nd order STSS and a time-varying form of PCA is considered. Although the possible applications of STSS have not been fully explored, it promises to reveal information that previously could not be easily measured, possibly enabling new domains of spectroscopy and remote sensing. I. INTRODUCTION II. CHARACTERIZING DEPARTURES FROM EQUILIBRIUM Statistical systems tend to fluctuate around equilib- A. Moments ria, and the non-equilibrium behavior of a system can be characterized by its fluctuations around equilibrium. Moreover, many quantum mechanical effects, e.g. emis- In order to analyze the statistical distribution of spec- sion from radiative transitions, introduce an intrinsically tral fluctuations from equilibrium, it is useful to consider random component to spectral data. In contrast to the a parameterization of its probability density function in classical equilibrium (which is time-independent by defi- terms of moments, cumulants, or other parameters. The nition) these transient random phenomena exhibit time- distribution of a random variable X gleaned from spec- dependent behavior. Repeated measurements of these tral data will generally be unknown, but it may be char- random events will reveal a characteristic statistical dis- acterized by the moments of the distribution of X. The n tribution that can potentially change over time. The as- nth raw moment is the expected value of X , where n is sociated random variables are often interpreted as noise an integer, and may be directly estimated from sample that has been superimposed onto an equilbrium spectra, data. Without taking into account sample bias, this is: and multiple spectra are often averaged in an attempt to n cancel their contribution, i.e. produce a less noisy spec- mn = E(X ) (1) trum. A moment generating function may be written for- We propose that, in addition to the average that is mally as the expected value of etX : traditionally extracted from the distribution of values tX present in a series of spectra, higher moments of this Mn(t) = E(e ) (2) distribution also contain useful information. In many cases, these higher moments reveal salient spectral fea- The moments mn are the coefficients in the MacLaurin arXiv:1504.01436v1 [physics.geo-ph] 6 Apr 2015 tures (e.g. emission lines) in much greater detail than expansion of M(t) averaged spectra. By considering the time series associ- dn ated with a particular spectral band as samples from a mn = M(0) (3) statistical distribution, we can extract information about dtn the non-equilbrium behavior of a system. Given this series expansion, knowlege of the moments In this paper, we focus on series of spectra that are pa- is equivalent to knowledge of the distribution of X. rameterized by time, characterizing statistical variations Sometimes 'moment' is used somewhat colloquially to over this parameter. However, the same analysis could refer to either the raw moment, as defined above, or the be applied to other parameters, spatial or otherwise, or central moments. The raw moments of X are taken about even multidimensional parameter spaces if the appropri- t = 0. In many cases, however, the quantities of interest ate multivariate statistics are considered. are the central moments, which are the moments taken 2 about t = µ. These correspond to the raw moments of a III. LIMITING CASES AND RELATED CONCEPTS distribution in which the mean has been shifted to zero. The nth central moment is equal to the nth raw moment A. Relation to p-norms of the X − µ. Whereas the first raw moment of X is its mean, µ, In some cases, it is useful to consider the trend of the the first central moment is zero, since X − µ necessar- nth moment as n becomes large. Since the value of the ily has mean zero. Higher central moments correspond nth moment tends to scale as xn, it is helpful in such to familiar statistics: the second central moment is the cases to consider the nth root of the nth moment, which variance, whose square root is the standard. The third we will call L , in order to maintain linearity. central moment the skew, and the fourth the kurtosis. n If a set of spectra is parameterized in more than one X n 1 L (X) = (jXj ) n (6) dimension (for example, two spatial dimensions) the mul- n tivariate moments over these parameters are tensors. A Defined in this way, Ln is equal to the p-norm of a multivariate second moment, for instance, may be repre- vector whose components are the elements of X, where sented by a matrix of covariances. n = p. Since the sum differs from the expectation op- erator by only a constant factor equal to the number of elements in the sum, the p-norm differs from the pth root B. Cumulants of the pth moment by the pth root of this constant. The familiar standard deviation of a Gaussian distribution, Equivalently, a statistical distribution can be repre- being the square root of variance, is essentially an L2 sented as a sequence of cumulants rather than a series of norm. One advantage of considering the p-norms rather moments. A cumulant generating function may be writ- than moments is that the p-norms are defined such that ten as the logarithm of the moment generating function. they remain linear in the units associated with the ran- g(t) = ln E(etX ) (4) dom variable X. The limit as n ! 1 is a special case, corresponding The cumulants κn are the coefficients in the MacLaurin to the p-norm where limp!1, the L1 norm, also called expansion of g(t) the uniform norm, Chebyshev norm, or supremum (sup) norm. L (X) is equal to the maximum absolute value dn 1 κn = g(0) (5) of an element of X divided by c, the number of elements dtn in X: Thus, the first n cumulants are equivalent to the first n L1 = lim Ln = max(jXj) (7) moments. The first, second, and third cumulants agreed n!1 with the first, second, and third central moments, respec- tively. However, higher cumulants are polynomial func- This can be very useful analyzing spectral timeseries tions of the higher moments. The first cumulant is the and may be quickly evaluated without floating-point op- mean µt(ν), which is equal to the first raw moment. The erations. Another useful p-norm is L1. This is sometimes second cumulant, the variance, is equal to the second cen- called the Manhattan distance or Taxicab distance. Al- tral moment, and the third cumulant is equal to the third though this does not explicitly correspond to a moment central moment, the skewness. The fourth and higher cu- or cumulant of the distribution, it can be a very useful mulants are not generally equal to the fourth and higher way to characterize a distribution. moments, however. The fourth cumulant is the excess kurtosis, which is sometimes simply called kurtosis, even though it is not equal to the third central moment (kur- B. Fractional p-norms and moments tosis), which sometimes leads to confusion. The fourth cumulant (excess kurtosis) is equal to the fourth central Given the definition of the nth moment, it is natural moment (kurtosis) minus three times the second central to consider cases in which n could take any real value, moment. In general, central moments and cumulants be- rather than an integer. For non-integer values of n and yond the third must be considered separately. Only the p, we obtain fractional (or fractal, for short) moments 1 R 1 itx delta distribution δ(x) = 2π −∞ e dt and the normal and norms. In such a case, the moments or norms be- distribution have a finite number of nonzero cumulants. come complex numbers, and there is no a priori reason Although moments are more easily defined and calcu- to restrict n to the real line - n could take any value lated than cumulants, the cumulants may be more conve- in the complex plane. Likewise, p-norms may be analyti- nient than moments in certain applications. Because the cally continued to the complex plane in a straightforward cumulants are additive, they often arise naturally in the manner, while also remaining linear in the original units.
Details
-
File Typepdf
-
Upload Time-
-
Content LanguagesEnglish
-
Upload UserAnonymous/Not logged-in
-
File Pages13 Page
-
File Size-