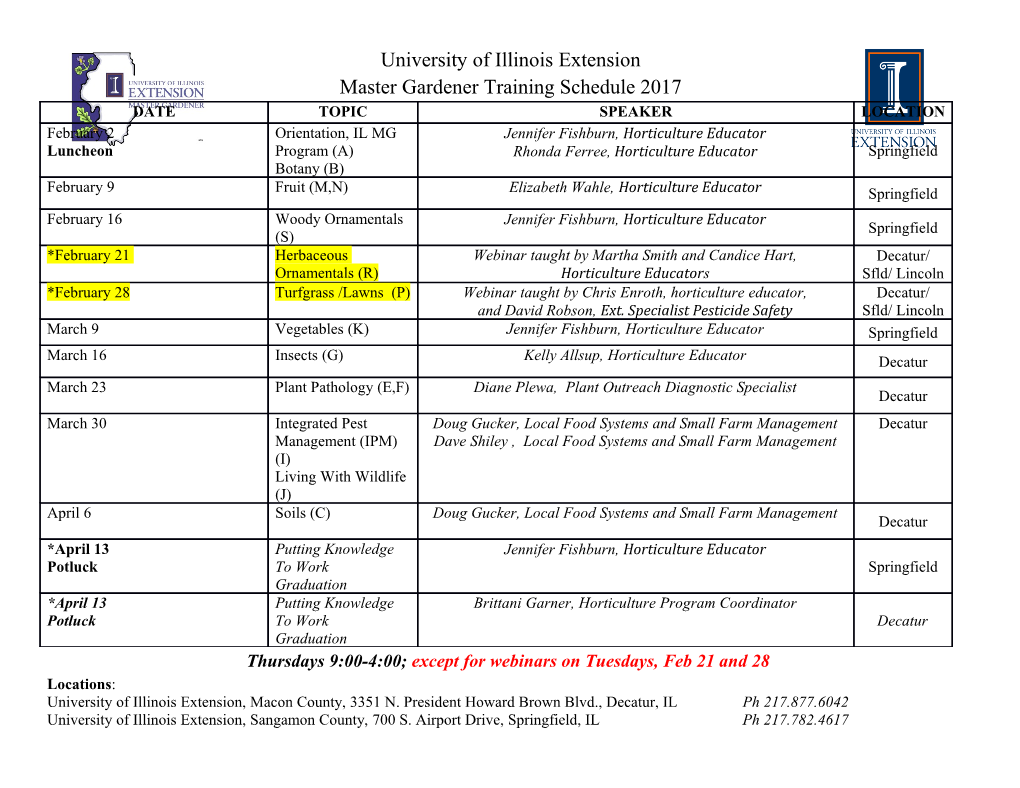
L. Vandenberghe ECE236C (Spring 2020) 15. Quasi-Newton methods variable metric methods • quasi-Newton methods • BFGS update • limited-memory quasi-Newton methods • 15.1 Newton method for unconstrained minimization minimize f x ¹ º f convex, twice continously differentiable Newton method 2 1 x = x t f x − f x k+1 k − kr ¹ kº r ¹ kº advantages: fast convergence, robustness, affine invariance • disadvantages: requires second derivatives and solution of linear equation • can be too expensive for large scale applications Quasi-Newton methods 15.2 Variable metric methods 1 x = x t H− f x k+1 k − k k r ¹ kº the positive definite matrix Hk is an approximatian of the Hessian at xk, chosen to: avoid calculation of second derivatives • simplify computation of search direction • ‘Variable metric’ interpretation (236B, lecture 10, page 11) 1 ∆x = H− f x − r ¹ º is the steepest descent direction at x for the quadratic norm 1 2 z = zT Hz / k kH Quasi-Newton methods 15.3 Quasi-Newton methods given: starting point x dom f , H 0 0 2 0 for k = 0;1;::: 1. compute quasi-Newton direction ∆x = H 1 f x k − k− r ¹ kº 2. determine step size tk (e.g., by backtracking line search) 3. compute xk+1 = xk + tk∆xk 4. compute Hk+1 different update rules exist for H in step 4 • k+1 can also propagate H 1 or a factorization of H to simplify calculation of ∆x • k− k k Quasi-Newton methods 15.4 Broyden–Fletcher–Goldfarb–Shanno (BFGS) update BFGS update yyT H ssT H H = H + k k k+1 k T T y s − s Hk s where s = x x ; y = f x f x k+1 − k r ¹ k+1º − r ¹ kº Inverse update T T T 1 sy 1 ys ss H− = I H− I + k+1 − yT s k − yT s yT s note that yT s > 0 for strictly convex f ; see page 1.8 • cost of update or inverse update is O n2 operations • ¹ º Quasi-Newton methods 15.5 Positive definiteness if yT s > 0, BFGS update preserves positive definitess of H • k this ensures that ∆x = H 1 f x is a descent direction • − k− r ¹ kº Proof: from inverse update formula, T T T T 2 T 1 s v 1 s v s v v H− v = v y H− v y + ¹ º k+1 − sT y k − sT y yT s if H 0, both terms are nonnegative for all v • k second term is zero only if sT v = 0; then first term is zero only if v = 0 • Quasi-Newton methods 15.6 Secant condition the BFGS update satisfies the secant condition Hk+1s = y where s = x x and y = f x f x k+1 − k r ¹ k+1º − r ¹ kº Interpretation: we define a quadratic approximation of f around xk+1 T 1 T f˜ x = f x + + f x + x x + + x x + H + x x + ¹ º ¹ k 1º r ¹ k 1º ¹ − k 1º 2¹ − k 1º k 1¹ − k 1º by construction f˜ x = f x • r ¹ k+1º r ¹ k+1º secant condition implies that also f˜ x = f x : • r ¹ kº r ¹ kº f˜ x = f x + H x x r ¹ kº r ¹ k+1º k+1¹ k − k+1º = f x r ¹ kº Quasi-Newton methods 15.7 Secant method for f : R R, BFGS with unit step size gives the secant method ! f 0 xk f 0 xk f 0 xk 1 xk+1 = xk ¹ º; Hk = ¹ º − ¹ − º − Hk xk xk 1 − − xk 1 xk xk+1 − f˜ x 0( ) f x 0( ) Quasi-Newton methods 15.8 Convergence Global result if f is strongly convex, BFGS with backtracking line search (EE236B, lecture 10-6) converges from any x , H 0 0 0 Local convergence if f is strongly convex and 2 f x is Lipschitz continuous, local convergence is r ¹ º superlinear: for sufficiently large k, x x? c x x? k k+1 − k2 ≤ k k k − k2 where c 0 k ! (cf., quadratic local convergence of Newton method) Quasi-Newton methods 15.9 Example m T X T minimize c x log bi ai x − i=1 ¹ − º n = 100, m = 500 Newton BFGS 103 103 100 100 ? ? f 3 f 3 10− 10− ) − ) − k k x 6 x 6 ( 10− ( 10− f f 9 9 10− 10− 10 12 10 12 − 0 2 4 6 8 10 12 − 0 50 100 150 k k cost per Newton iteration: O n3 plus computing 2 f x • ¹ º r ¹ º cost per BFGS iteration: O n2 • ¹ º Quasi-Newton methods 15.10 Square root BFGS update T to improve numerical stability, propagate Hk in factored form Hk = Lk Lk if H = L LT then H = L LT with • k k k k+1 k+1 k+1 αy˜ s˜ s˜T Lk+1 = Lk I + ¹ − º ; s˜T s˜ where T 1 2 1 T s˜ s˜ / y˜ = L− y; s˜ = L s; α = k k yT s if L is triangular, cost of reducing L to triangular form is O n2 • k k+1 ¹ º Quasi-Newton methods 15.11 Optimality of BFGS update X = Hk+1 solves the convex optimization problem minimize tr H 1X log det H 1X n ¹ k− º − ¹ k− º − subject to Xs = y cost function is nonnegative, equal to zero only if X = H • k also known as relative entropy between densities N 0; X , N 0; H • ¹ º ¹ kº BFGS update is a least-change secant update • optimality result follows from KKT conditions: X = Hk+1 satisfies 1 1 1 T T X− = H− sν + νs ; Xs = y; X 0 k − 2¹ º with yT 1y! ! 1 1 Hk− ν = 2H− y 1 + s sT y k − yT s Quasi-Newton methods 15.12 Davidon–Fletcher–Powell (DFP) update switch Hk and X in objective on previous page minimize tr H X 1 log det H X 1 n ¹ k − º − ¹ k − º − subject to Xs = y minimize relative entropy between N 0; H and N 0; X • ¹ kº ¹ º problem is convex in X 1 (with constraint written as s = X 1y) • − − solution is ‘dual’ of BFGS formula • ysT syT yyT Hk+1 = I Hk I + − sT y − sT y sT y (known as DFP update) predates BFGS update, but is less often used Quasi-Newton methods 15.13 Limited memory quasi-Newton methods 1 main disadvantage of quasi-Newton method is need to store Hk, Hk− , or Lk Limited-memory BFGS 1 (L-BFGS): do not store Hk− explicitly instead we store up to m (e.g., m = 30) values of • s = x x ; y = f x f x j j+1 − j j r ¹ j+1º − r ¹ jº we evaluate ∆x = H 1 f x recursively, using • k k− r ¹ kº T ! T ! T sj y yj s sj s 1 j 1 j j H− = I H− I + j+1 − T j − T T yj sj yj sj yj sj for j = k 1;:::; k m, assuming, for example, Hk m = I − − − an alternative is to restart after m iterations • cost per iteration is O nm , storage is O nm • ¹ º ¹ º Quasi-Newton methods 15.14 Interpretation of CG as restarted BFGS method first two iterations of BFGS (page 15.5) if H0 = I: 1 x = x t f x ; x = x t H− f x 1 0 − 0r ¹ 0º 2 1 − 1 1 r ¹ 1º where H is computed from s = x x and y = f x f x via 1 1 − 0 r ¹ 1º − r ¹ 0º T T T T 1 y y ss ys + sy H− = I + 1 + 1 ¹ sT y ºyT s − yT s if t is determined by exact line search, then f x T s = 0 • 0 r ¹ 1º quasi-Newton step in second iteration simplifies to • T 1 y f x1 H− f x1 = f x1 + r ¹ ºs − 1 r ¹ º −r ¹ º yT s this is the Hestenes–Stiefel conjugate gradient update nonlinear CG can be interpreted as L-BFGS with m = 1 Quasi-Newton methods 15.15 References J. E. Dennis, Jr., and R. B. Schabel, Numerical Methods for Unconstrained Optimization and • Nonlinear Equations (1996), chapter 9. C. T. Kelley, Iterative Methods for Optimization (1999), chapter 4. • J. Nocedal and S. J. Wright, Numerical Optimization (2006), chapter 6 and section 7.2. • Quasi-Newton methods 15.16.
Details
-
File Typepdf
-
Upload Time-
-
Content LanguagesEnglish
-
Upload UserAnonymous/Not logged-in
-
File Pages16 Page
-
File Size-