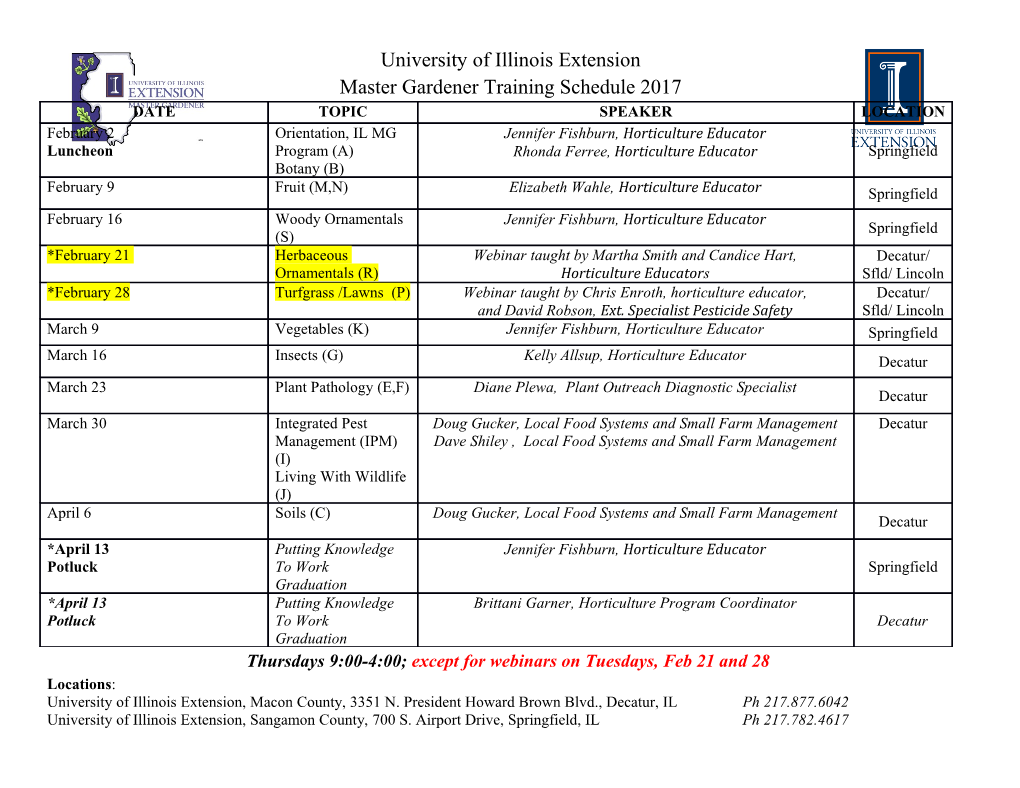
Mon. Not. R. Astron. Soc. 000, 1–11 (2011) Printed 5 November 2018 (MN LATEX style file v2.2) Directed follow-up strategy of low-cadence photometric surveys in Search of transiting exoplanets - I. Bayesian approach for adaptive scheduling Yifat Dzigan1⋆ and Shay Zucker1† 1Department of Geophysics and Planetary Sciences, Tel Aviv University, Tel Aviv 69978, Israel Accepted for publication in Monthly Notices of the Royal Astronomical Society Main Journal. Accepted 2011 April 8. ABSTRACT We propose a novel approach to utilize low-cadence photometric surveys for exoplan- etary transit search. Even if transits are undetectable in the survey database alone, it can still be useful for finding preferred times for directed follow-up observations that will maximize the chances to detect transits. We demonstrate the approach through a few simulated cases. These simulations are based on the Hipparcos Epoch Photometry data base, and the transiting planets whose transits were already detected there. In principle, the approach we propose will be suitable for the directed follow-up of the photometry from the planned Gaia mission, and it can hopefully significantly increase the yield of exoplanetary transits detected, thanks to Gaia. Key words: methods: data analysis – methods: observational – methods: statistical – techniques: photometric – surveys – planetary systems. 1 INTRODUCTION 2002). Thus the posterior detections motivated us to re- examine Hipparcos Epoch Photometry data and to look for a The idea to detect transits of exoplanets in the Hip- way to utilize this survey and similar low-cadence photomet- parcos Epoch Photometry (ESA 1997) trigerred several ric surveys, to detect exoplanets. The approach we propose studies that checked the feasibility of such an attempt. here is to use the data to maximize the chances to detect H´ebrard & Lecavelier Des Etangs (2006) concluded that transits during hypothetical follow-up campaigns, i.e., in- Hipparcos photometry did not look like an efficient tool stead of attempting to detect a transit, we use the data to for transit detection, without using any prior informa- schedule follow-up observations that together with the old tion. Indeed, some teams have made posterior detec- arXiv:1105.5393v1 [astro-ph.EP] 26 May 2011 data set may enable its detection. tions of the transits of HD 209458 (Robichon & Arenou In order to maximize the probability of sampling a tran- 2000; Castellano et al. 2000; Soderhjelm 1999), and of sit in those surveys, and in order to predict the best possible HD 189733 (Bouchy, F. et al. 2005), a detection that future observing times, we chose to use Bayesian inference H´ebrard & Lecavelier Des Etangs (2006) confirmed. Those methods. teams used the previously available knowledge of the orbital Bayesian analysis is based on Bayes theorem and can elements of the exoplanets (especially the period and the be written as: transit phase), in order to phase the Hipparcos data of those stars. Using the large time span that had elapsed since the Hipparcos observations (for example, about 830 orbital pe- p(Hi|I)p(D|Hi,I) p(Hi|D,I)= , (1) riods between Hipparcos observations and the observations p(D|I) that Castellano et al. (2000) used for HD 209458), the teams managed to drastically reduce the uncertainties of the known where p(Hi|D,I) is the posterior probability of the Hypoth- periods. The posterior detections of both transits prove that esis Hi, given the prior information, I, and the data, D. information about the transits does exist in the data, al- p(D|Hi,I) is the probability of obtaining the data D, though it is obviously futile to try to detect the transits in given that Hi and I are true. It is also known as the likeli- the na¨ıve Box Least Squares (BLS) approach (Kov´acs et al. hood function L(Hi). p(D|I) = i p(Hi|I)p(D|Hi,I) is a normalization fac- tor that ensuresP that i p(Hi|D,I) = 1. It is usually re- ⋆ E-mail: [email protected] ferred to as the priorP predictive probability for D, or the † E-mail: [email protected] global likelihood for the entire class of hypotheses. © 2011 RAS 2 Y. Dzigan and S. Zucker In the Bayesian framework we start from a prior knowl- 2 DIRECTED FOLLOW-UP edge we introduce into the prior probability distribution, Our ultimate goal is to detect transiting exoplanets using p(H0|I). The choice of prior distribution can affect the pos- terior distribution, especially if our observed data do not follow-up observations, which will be carefully scheduled to strongly constrain the model parameters. If our prior knowl- increase the chances to capture transits, should they exist. Thus, our approach does not focus on obtaining a detailed edge is poor, p(H0|I) can spread over a wide range of possi- ble values for the model parameters. transit model that best fits the available data, but on build- ing a probability distribution function of the parameters of Whenever new data are available, it is possible to in- a simple model, based on these data. The transit model that corporate the new data in our model through the likelihood we use in this work is a very simplistic one, based on the BLS function, combined with the prior, to obtain a new posterior philosophy (Kov´acs et al. 2002). Thus, we model a transit density probability, p(H0|D1,I), for the parameter. As soon light curve as a box-shaped transit with two phases, in and as we obtain another set of data, D2, we recalculate the pos- out of transit, and ignore the duration of the ingress and terior density probability in order for it to reflect our new egress phases, as well as the details of the limb darkening. state of knowledge. The possibility to combine new data sets These details are less relevant in low-precision, low-cadence into the original data we have will allow us to accomplish surveys, and using fewer parameters makes the model more our goal of detecting transiting exoplanets using scheduled robust. We use the Bayesian MH algorithm to obtain a pos- follow-up observations. terior probability distribution for the model parameters, and Gregory (2005a), Ford (2006) and others have already then use this distribution to prioritize the timing of the ob- shown that Bayesian inference is a useful tool for ana- servations of the chosen stars for follow-up observations. lyzing precise radial velocity (RV) data of planet-hosting The directed follow-up approach is not suitable for stars. Gregory (2007) used Bayesian inference model se- space missions like Corot or Kepler. Such missions, due lection for the problem of multiple planets, and Gregory to their high cadence, will not benefit from the approach (2005a) used it to construct posterior probability density since their phase and period coverage are already quite functions of the light-curve parameters. Defa¨yet al. (2001) complete. Instead, we aim for all-sky surveys like Hip- and Aigrain & Favata (2002) demonstrated the use of the parcos (van Leeuwen et al. 1997), or its successor, Gaia Bayesian approach to study planetary transits. (Jordi C. et al. 2006), in order to use their extensive low- Our implementation of Bayesian inference is based on cadence photometric databases for exoplanets search. the Metropolis-Hastings (MH) algorithm, which is a version A simplified (BLS-like) transit light curve is of the more general Markov-Chain Monte Carlo (MCMC) parametrized by five quantities, e.g., the period, phase, approach (Gregory 2005b). and width of the transit, and the flux levels in-transit and ex-transit. The first step in our proposed procedure is to A Markov chain is calculated using an initial set apply the MH algorithm to the Hipparcos measurements of of parameter values, X , and a transition probability, 0 a target star. This results in five Markov chains that include p(X |X ,I), that describes the probability of moving n+1 n the successful iterations for each one of the parameters. A from the current state to the next one. The transition proba- successful iteration is one that was accepted by the MH bility depends on the acceptance probability, described later algorithm. After removing the “burning time”, each chain in Section 3, and if properly constructed, then after exclud- represents the stationary distribution of the parameters, ing the so-called “burning time”, we can use the chain as a which we use as their estimated Bayesian posterior dis- sample from the desired distribution. MH Algorithm is an tributions, for our current state of knowledge (Gregory implementation of the MCMC that is used for obtaining a 2005b). Unlike the case of precise high-cadence surveys, sequence of random samples from a probability distribution. even if the star does host a transiting exoplanet, due to the The MH algorithm does not require good initial guess low precision and low cadence of the observations we do of the parameters values in order to estimate the posterior not expect the distribution to concentrate around a single distribution. This is one of the most important advantages solution, but rather show different periods that might fit of the algorithm. The algorithm is capable of exploring all the data, besides the unknown correct one. regions of the parameter space having significant probabil- The next step of our procedure is to assign each point ities (assuming it meets several basic requirements). The in time the probability that a transit will occur at that time. analysis also yields the marginal posterior probability dis- Calculating this probability is easy using the posterior dis- tribution functions for each of the model parameters, and tributions we found in the first stage.
Details
-
File Typepdf
-
Upload Time-
-
Content LanguagesEnglish
-
Upload UserAnonymous/Not logged-in
-
File Pages11 Page
-
File Size-