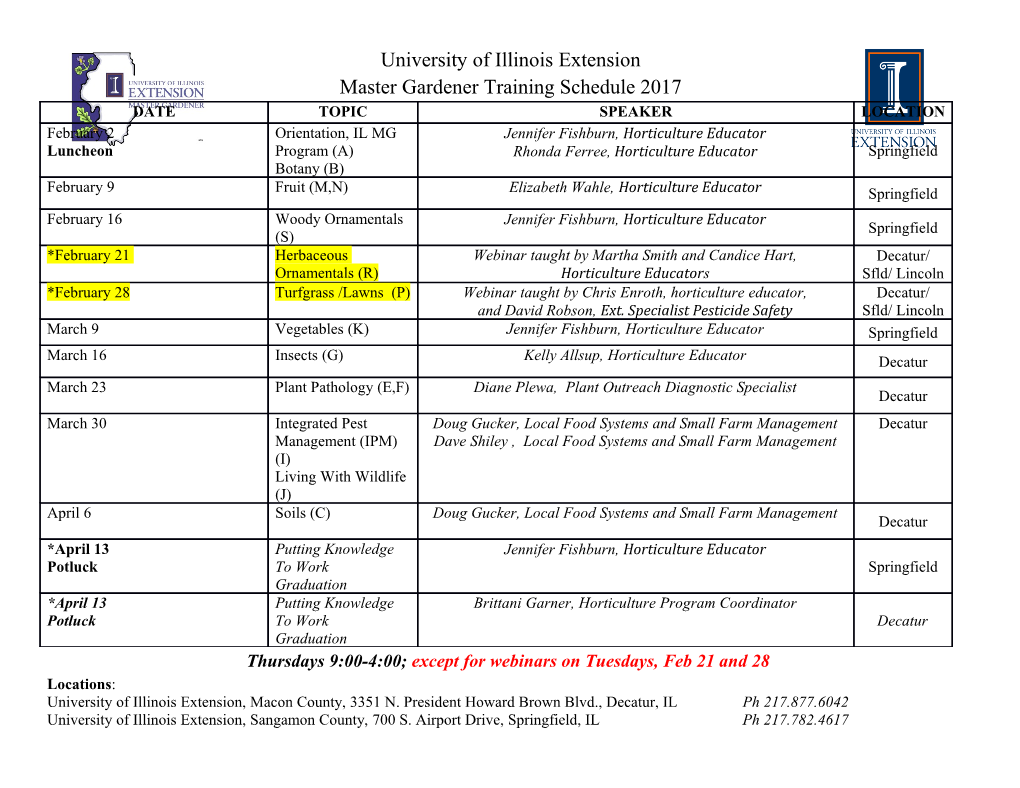
Integrating biclustering techniques with de novo gene regulatory network discovery using RNA-seq from skeletal tissues A Thesis Submitted to the College of Graduate Studies and Research in Partial Fulfillment of the Requirements for the degree of Master of Science in the Department of Computer Science University of Saskatchewan Saskatoon By Katie Ovens c Katie Ovens, October 2016. All rights reserved. Permission to Use In presenting this thesis in partial fulfilment of the requirements for a Postgraduate degree from the University of Saskatchewan, I agree that the Libraries of this University may make it freely available for inspection. I further agree that permission for copying of this thesis in any manner, in whole or in part, for scholarly purposes may be granted by the professor or professors who supervised my thesis work or, in their absence, by the Head of the Department or the Dean of the College in which my thesis work was done. It is understood that any copying or publication or use of this thesis or parts thereof for financial gain shall not be allowed without my written permission. It is also understood that due recognition shall be given to me and to the University of Saskatchewan in any scholarly use which may be made of any material in my thesis. Requests for permission to copy or to make other use of material in this thesis in whole or part should be addressed to: Head of the Department of Computer Science 176 Thorvaldson Building 110 Science Place University of Saskatchewan Saskatoon, Saskatchewan Canada S7N 5C9 i Abstract In order to improve upon stem cell therapy for osteoarthritis, it is necessary to understand the molecular and cellular processes behind bone development and the differences from cartilage formation. To further elucidate these processes would provide a means to analyze the relatedness of bone and cartilage tissue by determining genes that are expressed and regulated for stem cells to differentiate into skeletal tissues. It would also contribute to the classification of differences in normal skeletogenesis and degenerative conditions involving these tissues. The three predominant skeletal tissues of interest are bone, immature cartilage and mature cartilage. Analysis of the transcriptome of these skeletal tissues using RNA-seq technology was performed using differential expression, clustering and biclustering algorithms, to detect similarly expressed genes, which provides evidence for genes potentially interacting together to produce a particular phenotype. Identifying key regulators in the gene regulatory networks (GRNs) driving cartilage and bone development and the differences in the GRNs they drive will facilitate a means to make comparisons between the tissues at the transcriptomic level. Due to a small number of available samples for gene expression data in bone, immature and mature cartilage, it is necessary to determine how the number of samples influences the ability to make accurate GRN predictions. Machine learning techniques for GRN prediction that can incorporate multiple data types have not been well evaluated for complex organisms, nor has RNA-seq data been used often for evaluating these methods. Therefore, techniques identified to work well with microarray data were applied to RNA- seq data from mouse embryonic stem cells, where more samples are available for evaluation compared to the skeletal tissue RNA-seq samples. The RNA-seq data was combined with ChIP-seq data to determine if the machine learning methods outperform simple, correlation-based methods that have been evaluated using RNA-seq data alone. Two of the best performing GRN prediction algorithms from previous large-scale evaluations, which are incapable of incorporating data beyond expression data, were used as a baseline to determine if the addition of multiple data types could help reduce the number of gene expression samples. It was also necessary to identify a biclustering algorithm that could identify potentially biologically relevant modules. Publicly available ChIP-seq and RNA-seq samples from embryonic stem cells were used to measure the performance and consistency of each method, as there was a well-established network in mouse embryonic stem cells to compare results. The methods were then compared to cMonkey2, a biclustering method used in conjunction with ChIP-seq for two important transcription factors in the embryonic stem cell network. This was done to determine if any of these GRN prediction methods could potentially use the small number of skeletal tissue samples available to determine transcription factors orchestrating the expression of other genes driving cartilage and bone formation. Using the embryonic stem cell RNA-seq samples, it was found that sample size, if above 10, does not have a significant impact on the number of true positives in the top predicted interactions. Random forest methods outperform correlation-based methods when using RNA-seq, with area under ROC (AUROC) for evaluation, ii but the number of true positive interactions predicted when compared to a literature network were similar when using a strict cut-off. Using a limited set of ChIP-seq data was found to not improve the confidence in the transcription factor interactions and had no obvious affect on biclustering results. Correlation-based methods are likely the safest option when based on consistency of the results over multiple runs, but there is still the challenge of determining an appropriate cut-off to the predictions. To predict the skeletal tissue GRNs, cMonkey was used as an initial feature selection method to identify important genes in skeletal tissues and compared with other biclustering methods that do not use ChIP-seq. The predicted skeletal tissue GRNs will be utilized in future analyses of skeletal tissues, focussing on the evolutionary relationship between the GRNs driving skeletal tissue development. iii Acknowledgements I would like to thank my supervisors Ian McQuillan and Brian Eames for their constant support and encouragement. Their expectations have pushed me to new heights in my ability to effectively communicate my research to others. I feel the quality of my work has skyrocketed by applying their advice. They have also helped me to identify areas to continue improving, from their pain-staking effort proofreading my written work, watching and commenting on my presentations, and meeting with me weekly to discuss my progress. Patsy G´omezis also a continuing source of information regarding the biological background of this thesis, and was responsible for collecting the skeletal tissue data. Her and Brian's work have made the motivations behind my part in the research increasingly clear and I am constantly learning new things from them about the biological context of the project. Furthermore, I would like to thank the other members of my committee, Tony Kusalik, and Kevin Stanley for their time and valuable feedback on my thesis, from the research proposal to the final document. Also a special thanks to Chris Eskiw, who took the time out of his schedule to participate as an external examiner for my defence and provide me with feedback. Finally, I would like to thank my parents, who have always shown interest (or at least made it appear as such) in anything I have decided to pursue. Funding for my Masters thesis was provided by the Department of Computer Science at the University of Saskatchewan, as well as Ian and Brian through NSERC. iv Contents Permission to Use i Abstract ii Acknowledgements iv Contents v List of Tables vii List of Figures viii List of Abbreviations ix 1 Introduction 1 2 Research Objectives and Thesis Outline 5 3 Background 7 3.1 Gene Regulatory Networks . 7 3.1.1 Microarrays . 8 3.1.2 RNA-seq . 9 3.1.3 Sequence Data ie. ChIP-chip/seq . 9 3.1.4 Proteome, Metabolome Data and Biological Annotation . 10 3.2 Key Transcription Factors in Skeletal Cells . 10 3.3 Differential Expression and GRN Prediction . 13 3.4 Computational Methods for de novo GRN Discovery . 14 3.4.1 Clustering . 14 3.4.2 Biclustering Algorithms . 16 3.4.3 Review of Performance Evaluation of Biclustering Algorithms . 16 3.5 Beyond Feature Selection for GRN Discovery . 17 3.6 Limitations of Small Sample Sizes . 22 4 Methodology for Analysis of Gene Expression in Skeletal Tissues 25 4.1 Dataset Overview . 25 4.2 RNA-seq Analysis Pipeline and Comparison to Sox9 and Runx2 Literature Networks . 27 4.2.1 Mapping and Transcript Quantification . 27 4.2.2 Venn Diagrams of Genes Expressed in Skeletal Tissues . 28 4.2.3 Normalization and Differential Expression . 29 4.3 Model-Based Clustering . 29 4.3.1 Algorithm Description for Model-based Clustering . 29 4.4 Differentially Expressed and Unique Isoforms . 31 5 Results of Applied Bioinformatics Analysis to RNA-seq Data from Skeletal Tissues 33 5.1 Comparison of RNA-seq Data to Literature Networks for Sox9 and Runx2 . 33 5.1.1 Venn Diagrams of Genes Expressed in Skeletal Tissues . 33 5.1.2 Differential Expression . 37 5.2 Model-based Clustering . 39 5.2.1 Results . 39 5.3 Preliminary Analysis of Splice Variants . 43 v 5.3.1 Unique Isoforms . 44 5.3.2 Conclusion . 44 6 Methodology for GRN performance Evaluations for RNA-seq Data 47 6.1 Comparison of Biclustering Methods for GRN Discovery . 47 6.1.1 Biclustering Programs . 48 6.1.2 Evaluation Metrics for Plaid, SAMBA and FABIA . 49 6.2 Performance Evaluation of GRN Prediction Methods in Mouse . 50 6.2.1 Na¨ıve Embryonic Stem Cell (ESC) Gene Regulatory Network . 50 6.3 Random Forest . 53 6.3.1 GENIE3 . 53 6.4 ChIP-seq Data Integration . 53 6.4.1 iRafnet . 54 6.4.2 cMonkey2 . 56 6.4.3 Inferelator . 57 6.5 Evaluation . 57 6.6 Microarray and RNA-seq Comparisons . 58 6.6.1 Generation of ROC curves . 58 6.7 Measuring Consistency of GRN Prediction Methods .
Details
-
File Typepdf
-
Upload Time-
-
Content LanguagesEnglish
-
Upload UserAnonymous/Not logged-in
-
File Pages160 Page
-
File Size-