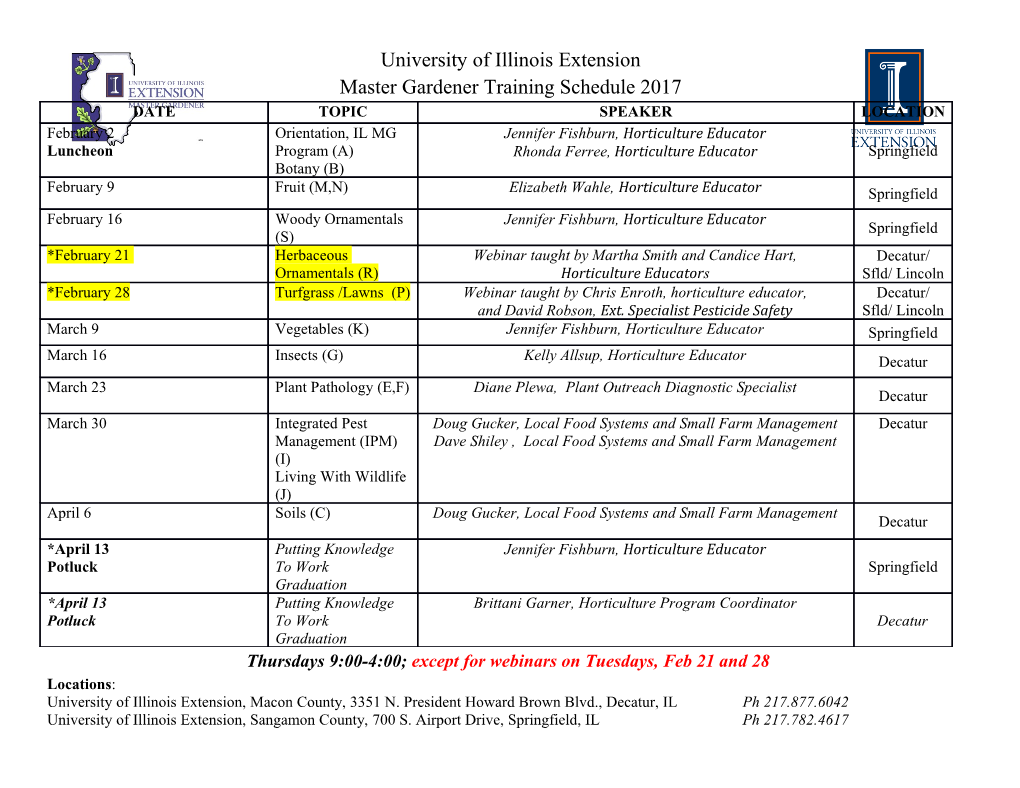
Investigation of Nonlinearity in Hyperspectral Remotely Sensed Imagery by Tian Han B.Sc., Ocean University of Qingdao, 1984 M.Sc., University of Victoria, 2003 A Dissertation Submitted in Partial Fulfillment of the Requirements for the Degree of DOCTOR OF PHILOSOPHY in the Department of Computer Science © Tian Han, 2009 University of Victoria All rights reserved. This dissertation may not be reproduced in whole or in part, by photocopying or other means, without the permission of the author. Library and Archives Bibliothèque et Canada Archives Canada Published Heritage Direction du Branch Patrimoine de l’édition 395 Wellington Street 395, rue Wellington Ottawa ON K1A 0N4 Ottawa ON K1A 0N4 Canada Canada Your file Votre référence ISBN: 978-0-494-60725-1 Our file Notre référence ISBN: 978-0-494-60725-1 NOTICE: AVIS: The author has granted a non- L’auteur a accordé une licence non exclusive exclusive license allowing Library and permettant à la Bibliothèque et Archives Archives Canada to reproduce, Canada de reproduire, publier, archiver, publish, archive, preserve, conserve, sauvegarder, conserver, transmettre au public communicate to the public by par télécommunication ou par l’Internet, prêter, telecommunication or on the Internet, distribuer et vendre des thèses partout dans le loan, distribute and sell theses monde, à des fins commerciales ou autres, sur worldwide, for commercial or non- support microforme, papier, électronique et/ou commercial purposes, in microform, autres formats. paper, electronic and/or any other formats. The author retains copyright L’auteur conserve la propriété du droit d’auteur ownership and moral rights in this et des droits moraux qui protège cette thèse. Ni thesis. Neither the thesis nor la thèse ni des extraits substantiels de celle-ci substantial extracts from it may be ne doivent être imprimés ou autrement printed or otherwise reproduced reproduits sans son autorisation. without the author’s permission. In compliance with the Canadian Conformément à la loi canadienne sur la Privacy Act some supporting forms protection de la vie privée, quelques may have been removed from this formulaires secondaires ont été enlevés de thesis. cette thèse. While these forms may be included Bien que ces formulaires aient inclus dans in the document page count, their la pagination, il n’y aura aucun contenu removal does not represent any loss manquant. of content from the thesis. ii Investigation of Nonlinearity in Hyperspectral Remotely Sensed Imagery By Tian Han B.Sc., Ocean University of Qingdao, 1984 M.Sc., University of Victoria, 2003 Supervisory Committee Dr. David J. Goodenough, Supervisor (Department of Computer Science) Dr. Jens H. Weber, Co-Supervisor (Department of Computer Science) Dr. Dale Olesky, Departmental Member (Department of Computer Science) Dr. K. Olaf Niemann, Outside Member (Department of Geography) iii Supervisory Committee Dr. David J. Goodenough, Supervisor (Department of Computer Science) Dr. Jens H. Weber, Co-Supervisor (Department of Computer Science) Dr. Dale Olesky, Departmental Member (Department of Computer Science) Dr. K. Olaf Niemann, Outside Member (Department of Geography) Abstract Hyperspectral remote sensing excels in its high spectral resolution, which enables the generation of contiguous spectral profiles covering the visible to shortwave infrared region (400 – 2500 nm) of the solar electromagnetic spectrum. The high spectral resolution has greatly stimulated the applications of hyperspectral remote sensing in different disciplines. The initial applications have been found in mineral exploration, followed by applications in environmental research, forest health evaluation, vegetation species mapping, precision farming, water pollution monitoring, and military target identification. It has been noticed, however, that there is an inconsistency between the statistical characteristics of hyperspectral remotely sensed data and the methods employed to model and process the data for information extraction. On the one hand, hyperspectral data are considered inherently nonlinear, due to the multiple nonlinear sources involved in the data formation. On the other hand, hyperspectral data has long been modeled and processed as realisations of some linear stochastic processes. What is the impact of this inconsistency on hyperspectral data analysis? This dissertation is prepared to address this question by firstly evaluating the significance of nonlinearity in hyperspectral data and iv secondly examining the influence of nonlinearity on dimensionality estimation, and noise reduction. This dissertation proved that nonlinearity existed in hyperspectral data and it was statistically significant. It was found that the dimension of hyperspectral data was substantially smaller when the nonlinearity was considered compared to estimations based on linear algorithms. It was demonstrated that improved noise reduction was achieved without compromising spectral absorption features if the nonlinearity was taken into consideration. The algorithms discussed in this dissertation were implemented, which provided a useful tool set for those who are interested in studying the nonlinear behaviours in hyperspectral data, which are not available in commercial remote sensing software packages. v Table of Contents Supervisory committee .............................................................................................. ii Abstract...................................................................................................................... iii Table of contents .........................................................................................................v List of tables.............................................................................................................. vii List of figures........................................................................................................... viii Acknowledgements .....................................................................................................x Dedication .................................................................................................................. xi 1 Introduction..............................................................................................................1 1.0 Hyperspectral remote sensing technology ..........................................................4 1.1 Applications of hyperspectral remote sensing ....................................................8 1.2 Characteristics of hyperspectral data ................................................................12 1.3 Hyperspectral data of interest ...........................................................................16 2 Investigation of nonlinearity .................................................................................19 2.0 Motivation.........................................................................................................19 2.1 Data sets............................................................................................................23 2.2 Algorithm description .......................................................................................24 2.3 Results and discussion ......................................................................................29 2.4 Chapter summary..............................................................................................40 3 Nonlinearity-counted dimension estimation........................................................41 3.0 Motivation.........................................................................................................41 3.1 Data sets............................................................................................................44 3.2 Algorithm description .......................................................................................44 3.3 Results and discussion ......................................................................................46 vi 3.4 Computational complexity................................................................................54 3.5 Chapter summary..............................................................................................58 4 Nonlinearity-counted noise reduction..................................................................60 4.0 Motivation.........................................................................................................61 4.1 Data sets............................................................................................................64 4.2 Algorithm description .......................................................................................64 4.3 Results and discussion ......................................................................................69 4.4 Computational complexity................................................................................76 4.5 Chapter summary..............................................................................................77 5 Algorithm implementation....................................................................................80 5.0 Motivation.........................................................................................................80 5.1 Application programming interface..................................................................81 5.2 Algorithm implementation................................................................................83 5.3 Chapter summary..............................................................................................89
Details
-
File Typepdf
-
Upload Time-
-
Content LanguagesEnglish
-
Upload UserAnonymous/Not logged-in
-
File Pages122 Page
-
File Size-