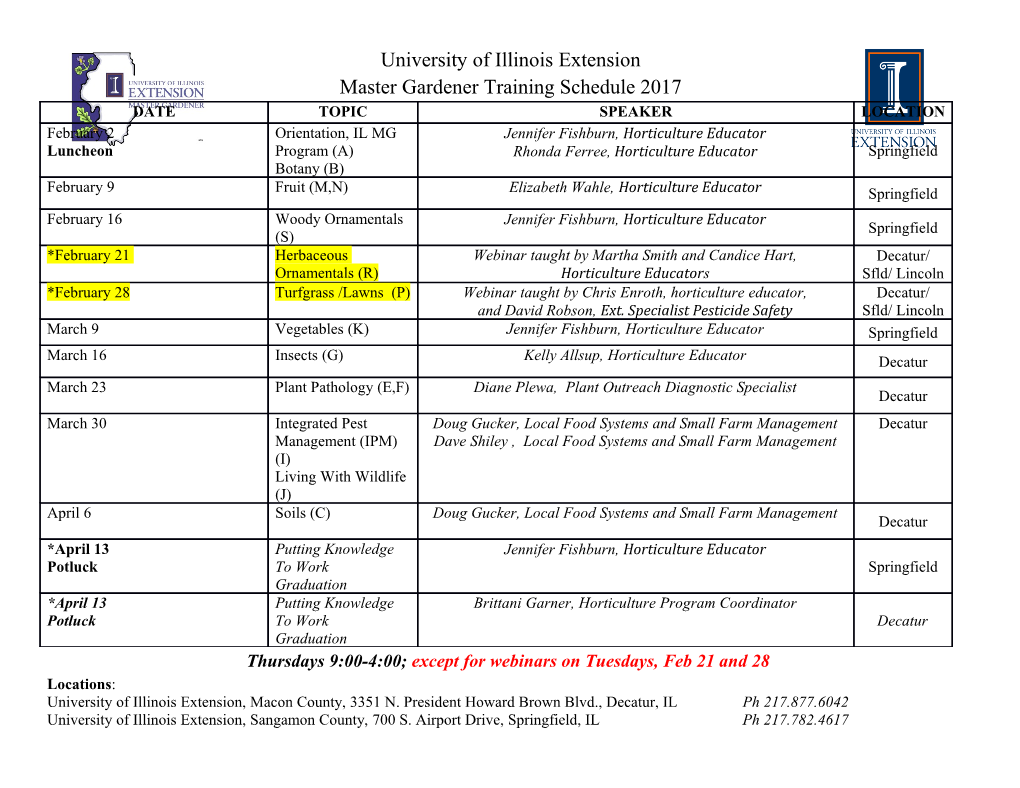
EBOOK-ASTROINFORMATICS SERIES: IEEECSCONNECT-AN INITIATIVE OF IEEE COMPUTER SOCIETY BANGALORE CHAPTER A MANUAL ON MACHINE LEARNING AND ASTRONOMY: AUTHORED, EDITED AND COMPILED BY SNEHANSHU SAHA Page 1 of 316 June 15, 2019 Chapter contributions from: Suryoday Basak, Rahul Yedida, Kakoli Bora Archana Mathur, Surbhi Agrawal, Margarita Safonova Nithin Nagaraj, Gowri Srinivasa, Jayant Murthy PES University University of Texas at Arlington North Carolina State University Indian Statistical Institute National Institute for Advanced Studies Indian Institute of Astrophysics June 15, 2019 2 Preface The E-book is dedicated to the new field of Astroinformatics: an interdisciplinary area of research where astronomers, mathematicians and computer scientists collaborate to solve problems in astronomy through the application of techniques developed in data science. Classical problems in astronomy now involve the accumulation of large volumes of complex data with different formats and characteristic and cannot be addressed using classical tech- niques. As a result, machine learning (ML) algorithms and data analytic techniques have exploded in importance, often without a mature understanding of the pitfalls in such studies. This E-book aims to capture the baseline, set the tempo for future research in India and abroad, and prepare a scholastic primer that would serve as a standard document for future research. The E-book should serve as a primer for young astronomers willing to apply ML in astronomy, a way that could rightfully be called "Machine Learning Done Right", borrowing the phrase from Sheldon Axler ("Linear Algebra Done Right")! The motivation of this handbook has two specific objectives: • develop efficient models for complex computer experiments and data analytic tech- niques which can be used in astronomical data analysis in the short term, and various related branches in physical, statistical, computational sciences much later (larger goal as far as memetic algorithm is concerned). • develop a set of fundamentally correct thumb rules and experiments, backed by solid mathematical theory, and render the marriage of astronomy and Machine Learning stability for far reaching impact. We will do this in the context of specific science prob- lems of interest to the proposers: the classification of exoplanets, classification of nova, separation of stars, galaxies and quasars in the survey catalogs, and the classification of multi-wavelength sources. We hope the E-book serves its purpose and inspires scientists across communities to collabo- rate and develop a very promising field. We gratefully acknowledge the grant (File Number: EMR/2016/005687) received from SCIENCE & ENGINEERING RESEARCH BOARD (SERB), under the scheme- Extra Mural Research (EMR), a division of DST. ************************************************************************************************ Sincerely, Authors Page 3 of 316 Contents 1 Introduction 10 2 Pros and Cons of Classification of Exoplanets: in Search for the Right Habitability Metric 12 2.1 References:......................................... 17 3 A Comparative Study in Classification Methods of Exoplanets: Machine Learning Exploration via Mining and Automatic Labeling of the Habitability Catalog 19 3.1 Introduction........................................ 19 3.2 Motivation......................................... 23 3.3 Methods.......................................... 24 3.3.1 Naïve Bayes.................................... 25 3.3.2 Metric Classifiers................................. 26 3.3.3 Non-Metric Classifiers.............................. 29 3.4 Framework and Experimental Set Up......................... 32 3.4.1 Data Acquisition: Web Scraping........................ 32 3.4.2 Classification of Data............................... 34 3.5 Complexity of the data set used and Results..................... 36 3.5.1 Classification performed on an unbalanced and smaller Data Set.... 36 3.5.2 Classification performed on a balanced and smaller data set....... 37 3.5.3 Classification performed on a balanced and larger data set........ 39 3.6 Discussion......................................... 44 3.6.1 Note on new classes in PHL-EC......................... 44 3.6.2 Missing attributes................................. 44 3.6.3 Reason for extremely high accuracy of classifiers before artificial balanc- ing of data set................................... 45 3.6.4 Demonstration of the necessity for artificial balancing........... 46 3.6.5 Order of importance of features........................ 46 3.6.6 Why are the results from SVM, K-NN and LDA relatively poor?...... 47 3.6.7 Reason for better performance of decision trees............... 47 3.6.8 Explanation of OOB error visualization.................... 49 3.6.9 What is remarkable about random forests?.................. 50 3.6.10 Random forest: mathematical representation of binomial distribution and an example.................................. 50 Page 4 of 316 3.7 Binomial distribution based confidence splitting criteria.............. 51 3.7.1 Margins and convergence in random forests................. 53 3.7.2 Upper bound of error and Chebyshev inequality.............. 53 3.7.3 Gradient tree boosting and XGBoosted trees................. 53 3.7.4 Classification of conservative and optimistic samples of potentially hab- itable planets................................... 57 3.8 Habitability Classification System applied to Proxima b.............. 58 3.9 Data Synthesis and Artificial Augmentation..................... 58 3.9.1 Generating Data by Assuming a Distribution................. 59 3.9.2 Artificially Augmenting Data in a Bounded Manner............. 59 3.9.3 Fitting a Distribution to the Data Points.................... 62 3.9.4 Generating Data by Analyzing the Distribution of Existing Data Empiri- cally: Window Estimation Approach...................... 71 3.9.5 Estimating Density................................ 71 3.9.6 Generating Synthetic Samples......................... 72 3.10 Results of Classification on Artificially Augmented Data Sets........... 73 3.11 Conclusion......................................... 74 4 CD-HPF: New Habitability Score Via Data Analytic Modeling 78 4.1 Introduction........................................ 78 4.1.0.1 Biological Complexity Index (BCI) ................. 80 4.2 CD-HPF: Cobb-Douglas Habitability Production Function............ 81 4.3 Cobb-Douglas Habitability Production Function CD-HPF............. 83 4.4 Cobb-Douglas Habitability Score estimation..................... 85 4.5 The Theorem for Maximization of Cobb-Douglas habitability production function 86 4.6 Implementation of the Model.............................. 88 4.7 Computation of CDHS in DRS phase......................... 89 4.8 Computation of CDHS in CRS phase.......................... 89 4.9 Attribute Enhanced K-NN Algorithm: A Machine learning approach....... 94 4.10 Results and Discussion ................................. 95 4.11 Conclusion and Future Work.............................. 100 5 Theoretical validation of potential habitability via analytical and boosted tree meth- ods: An optimistic study on recently discovered exoplanets 102 5.1 Introduction........................................ 102 5.2 Analytical Approach via CDHS: Explicit Score Computation of Proxima b.... 105 Page 5 of 316 5.2.1 Earth Similarity Index.............................. 105 5.2.2 Cobb Douglas Habitability Score (CDHS)................... 106 5.2.3 CDHS calculation using radius, density, escape velocity and surface temperature.................................... 107 5.2.4 Missing attribute values: Surface Temperature of 11 rocky planets (Table I)107 5.2.5 CDHS calculation using stellar flux and radius................ 109 5.2.6 CDHS calculation using stellar flux and mass................ 110 5.3 Elasticity computation: Stochastic Gradient Ascent (SGA)............. 111 5.3.1 Computing Elasticity via Gradient Ascent................... 111 5.3.2 Computing Elasticity via Constrained Optimization............ 112 6 Comparing Habitability Metrics 116 6.1 Earth Similarity Index (ESI)............................... 117 6.2 Discussion......................................... 124 7 Supernova Classification 128 7.1 Introduction........................................ 128 7.2 Categorization of Supernova.............................. 129 7.3 Type I supernova..................................... 129 7.4 Type II supernova..................................... 130 7.5 Machine Learning Techniques............................. 131 7.6 Supernovae Data source and classification...................... 133 7.7 Results and Analysis................................... 133 7.8 Conclusion......................................... 134 7.9 Future Research Directions............................... 134 8 Machine Learning Done Right: A Case Study in Quasar-Star Classification 136 8.1 Introduction........................................ 136 8.2 Motivation and Contribution.............................. 138 8.3 Star-Quasar Classification: Existing Literature.................... 140 8.4 Data Acquisition..................................... 141 8.5 Methods.......................................... 143 8.5.1 Artificial Balancing of Data........................... 143 9 An Introduction to Image Processing 144 9.1................................................ 144 Page 6 of 316 10 A study in emergence of AstroInformatics: A Novel Method in Big Data Mining 145 10.1 INTRODUCTION..................................... 145 10.2 The depths of Dimensionality Reduction......................
Details
-
File Typepdf
-
Upload Time-
-
Content LanguagesEnglish
-
Upload UserAnonymous/Not logged-in
-
File Pages316 Page
-
File Size-